Siwei Lyu, a computer scientist at the University at Buffalo (UB) and an expert in deepfake technology, has introduced novel deepfake detection algorithms designed to mitigate biases present in existing models.
The algorithms aim to address disparities in accuracy across races and genders, a prevalent issue observed in many current deepfake detection systems.
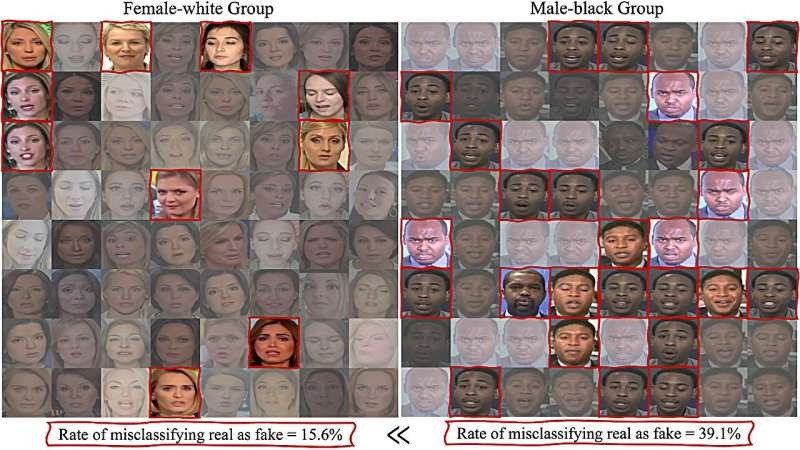
Bias in Deepfake Detection Systems
Lyu, who co-directs the UB Center for Information Integrity, revealed that the motivation for developing these algorithms arose from the inherent bias found in detection systems, including their own.
Lyu created a photo collage from the hundreds of real faces that his detection algorithms had wrongly classified as fake. Strikingly, the new composition had a predominantly darker skin tone, highlighting the bias in the algorithms.
"A detection algorithm's accuracy should be statistically independent from factors like race, but obviously many existing algorithms, including our own, inherit a bias," Lyu said in a statement.
The two machine learning methods Lyu and his team devised represent an ambitious effort to create deepfake detection algorithms with reduced biases.
The methods take different approaches - one makes algorithms aware of demographics, while the other keeps them blind to such information. Both methods aim to diminish disparities in accuracy based on race and gender while, in some instances, enhancing overall accuracy.
Lyu noted that recent studies have revealed significant discrepancies in error rates among different races in existing algorithms, with some performing better on lighter-skinned subjects than darker-skinned ones.
These disparities can lead to certain groups facing a higher risk of having their authentic images misclassified as fake or, more detrimentally, manipulated images of them being erroneously identified as real.
Lyu explained that the root of the problem lies in the data used to train these algorithms. Photo and video datasets often overrepresent middle-aged white men, resulting in algorithms that are more adept at analyzing this group and less accurate with underrepresented demographics.
"Say one demographic group has 10,000 samples in the dataset and the other only has 100. The algorithm will sacrifice accuracy on the smaller group in order to minimize errors on the larger group. So it reduces overall errors, but at the expense of the smaller group," Lyu noted.
Read Also : Camera Giants Nikon, Sony, and Canon Unite to Combat Deepfakes with Cutting-Edge Digital Signatures
Improving the Fairness of Algorithms
While prior attempts have sought to rectify this problem by achieving demographic balance in datasets, Lyu's team claims to be the first to concentrate on improving the algorithms' fairness.
Lyu employed an analogy to explain their approach, comparing it to evaluating a teacher based on student test scores. He clarified that instead of favoring the dominant group, their method seeks to provide a weighted average to middle-group students, compelling the algorithm to focus more broadly and thereby mitigating bias.
The FaceForensic++ dataset and the Xception detection algorithm were utilized to assess the two methods: demographic-aware and demographic-agnostic.
The demographic-aware method demonstrated superior performance, exhibiting enhancements in fairness metrics, including equalizing false positive rates among different races.
Crucially, Lyu emphasized that their methods not only increased fairness but also enhanced the overall detection accuracy of the algorithm, from 91.49% to as high as 94.17%.
Lyu acknowledged a small tradeoff between performance and fairness but emphasized that their methods ensure limited performance degradation.
He noted that while improving dataset quality remains the fundamental solution to bias problems, incorporating fairness into algorithms is a crucial first step. The study's findings were published in arXiv.
Related Article : Experts Warn AI Misinformation May Get Worse These Upcoming Elections
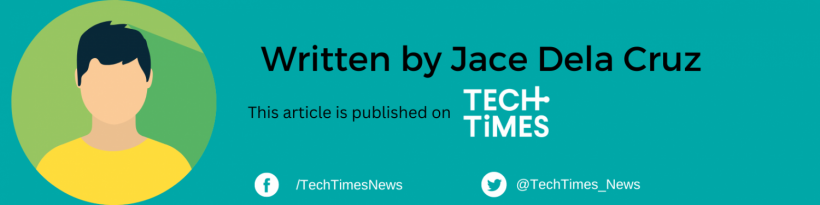