For years, scientists have long questioned the viability of predicting protein functional shapes through the concept of understanding their initial foundational structure or building blocks of the proteins. Generally, the idea itself has remained far too complex for the human mind as of yet, as these protein structures tend to take on far varying changes when interacted with on a physical level, thus leaving the idea rather open to interpretation.
That is, until today. Published in Nature Communications, scientists at Oak Ridge National Laboratory and Georgia Tech have figured out a way to bridge complicated and innumerable patterns made via proteins and essentially catalog these protein functional shapes in an artificial neural network model or deep learning AI. The process thus allows researchers to literally "see" protein functional shapes in a more three-dimensional construct and gives them the ability to predict how these forms are made going into the future.
The computational model, named AlphFold 2, can sift through data and numbers to uncover the biologically active process in proteins, as well as highlight the same feature in complexes, otherwise known as protein pairings. These discoveries would allow biologists and assorted researchers a whole new outlook on the potential of molecular systems and the process by which they are formed.
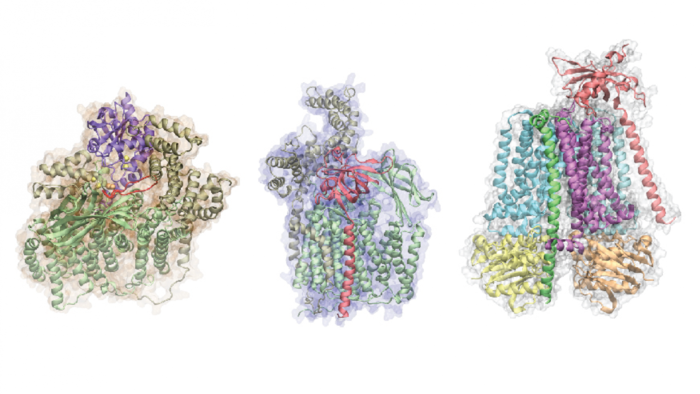
Jeffrey Skolnick, among the co-authors of the report and a Regents' Professor at the School of Biological Sciences, best described these findings as an ultimate breakthrough in the field if, of course, said computational models prove successful in the long run. Utilizing such deep learning processes will only ensure the ever-expanded growth of research, as well as streamline overall tests going forward.
The AlphaFold 2 program, which was designed by the artificial intelligence DeepMind lab in London, was able to perform successful blind tests of the researcher's Community Wide Experiment on Critical Assessment of Techniques for Protein Structure Prediction (CASP14) at the 14th bi-annual competition.
Mu Gao, co-author alongside Skolnick and a senior research scientist at the School of Biological Sciences, explained the entire complicated process with ease:
"The physical interactions between different [protein domains of the same sequence are essentially the same as the interactions gluing different proteins together. It quickly became clear that relatively simple modifications to AlphaFold 2 could allow it to predict the structural models of a protein complex."
Thus, the researchers went on to only advance their complicated procedures via a whole new program with the help of an undergrad student from the School of Computer Science, Davi Nakajima An. Taking these input features from the multitude of protein sequences and smashing those together with all new evaluation metrics that study proteins' interactive strength within the probe, the team then brought to life their new computational model: the AF2Complex.
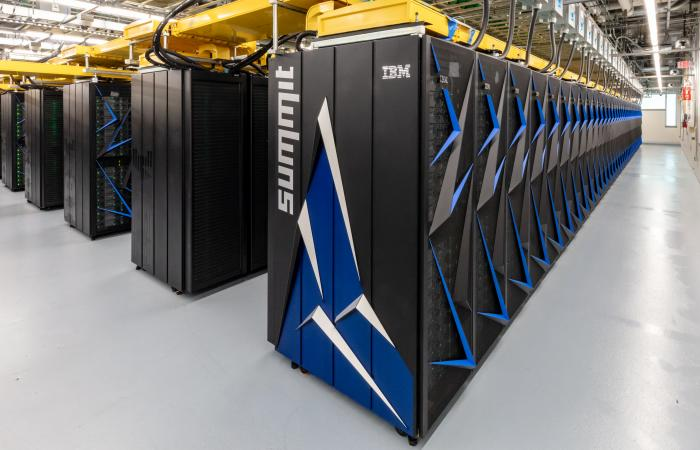
Alongside Georgia Tech's computing center, called Partnership for an Advanced Computing Environment (PACE), the team set AF2Complex to its most challenging paradigm. It had to uncover protein forms and sequences it had never before seen, which stunningly the AF2Complex proved its might by unveiling over twice as many protein complexes as per the original methodology of docking.
"Encouraged by these promising results, we extended this idea to an even bigger problem, which is to predict interactions among multiple arbitrarily chosen proteins, e.g., in a simple case, two arbitrary proteins," Skolnick explains. Out of 500 pairs of proteins, AF2Complex was then tasked with disclosing which of them were capable of forming a complex. It not only outperformed the docking method, but also even AlphaFold 2.
Taking the AF2Complex even further into its insight capabilities, the research team then paired up with Summit Oak Ridge Leadership Computing Facility to test the computational method on its swathe of powerful supercomputers. To the delight of the researchers, once more AF2Complex proved more than adequate, highlighting many various pairs of proteins that can form complexes, as well as some never before seen in any experimentation.
"These computational models now provide insights into the molecular mechanisms for how this biomolecular system works," Gao explained. Skolnick added, "Deep learning is changing the way one studies a biological system. We envision methods like AF2Complex will become powerful tools for any biologist who would like to understand molecular mechanisms of a biosystem involving protein interactions."