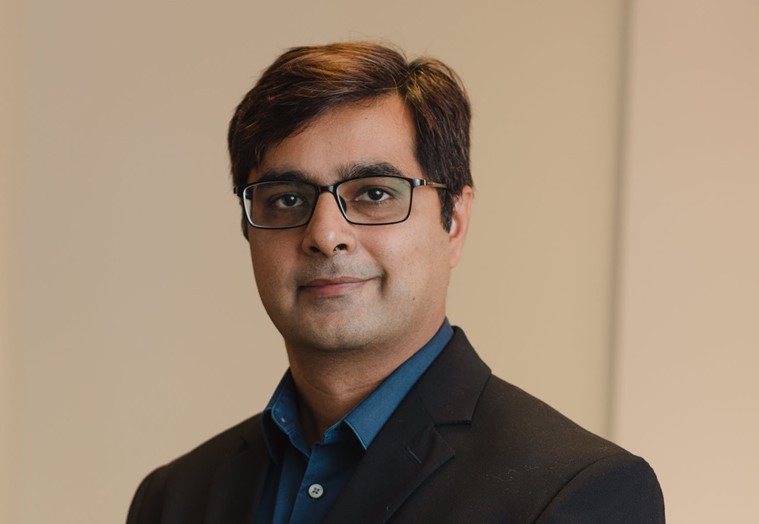
Among the skyscrapers of Chicago's financial district, Nitesh Khullar is quietly orchestrating a technological overhaul of credit risk management. A senior vice president at a major international banking firm, Khullar seeks to wield the capabilities of machine learning for improved operationality, mitigating financial risk and ensuring that the right loans go to those who need them most. Khullar is an industry leader with immense experience in data and predictive modeling. His contributions have led his financial institution to strike a balance between risk and growth of the business and better prepare for recession scenarios.
Credit risk assessment as a whole has been turned on its head in recent years. Gone are the days when bankers relied solely on traditional credit scores and gut feelings. Today, sophisticated algorithms analyze vast datasets to uncover patterns that were once invisible. Khullar, among the first to realize this potential, lends insight into the developing world of advanced and secure financial technology.
A New Dawn in Credit Risk Management
"The financial world is at a crossroads," Khullar remarks. "We're moving away from conventional scorecards and replacing them with machine learning models that can adapt in real-time. This means, as the market and economy change, so too will our assessment systems." Khullar's experience in predictive modeling has led to the adoption of advanced ML algorithms and systems, which helped to make decisions within a few seconds.
Such an upgrade couldn't come at a more critical time. With global economic uncertainties casting long shadows, being able to predict credit risk accurately is more vital than ever before.
According to the International Monetary Fund, global growth is projected to slow to 2.8% in 2024 from 3.0% in 2023, highlighting the urgent need for effective risk management tools in an unpredictable economy.
Khullar's team is among the few directly working on the implementation of these next-generation tools. "Our machine learning models sift through mountains of alternative data—from social media activity to utility bill payments—offering a more realistic view of a borrower's financial standing," he explains.
Khullar emphasizes the use of alternate data sources to evaluate the risk pattern. Alternate data sources such as utility bills, rental, and transactional patterns can be leveraged to evaluate the risk behavior of credit invisibles or low credit history, giving banks another chance to make credit decisions for these consumers.
Industry trends appear to follow the same path, with one 2023 survey by Forrester Research finding that 68% of financial institutions are ramping up their investments in AI and machine learning for risk assessment.
The Double-Edged Sword of AI in Finance
The rise of Machine learning/AI in credit decision-making isn't without its detractors. One data scientist and author known for her critical take on big data warns, "While ML/AI promises greater accuracy, it also risks making existing biases worse, especially in lending practices. Marginalized groups may be ignored even more."
Khullar has acknowledged these concerns in his work but argues that well-crafted ML/AI systems can actually help reduce bias. "We've prioritized fairness checks first and foremost, including disparate impact analysis. This way, we can be sure that our models won't discriminate against protected classes," he asserts.
As the adoption of artificial intelligence throughout the finance world continues, regulatory scrutiny appears to voice the same concerns. The U.S. Consumer Financial Protection Bureau (CFPB) has signaled heightened oversight of ML/AI-driven lending practices. Fortunately, Khullar's experience managing audits from regulators like the CFPB and OCC has ascertained that his work is entirely above board and in compliance.
"Compliance isn't just about ticking boxes," Khullar emphasizes. "Ultimately, the goal is to build trust. We're working to make our ML/AI models more transparent—not just to regulators but also to consumers."
Charting the Course Ahead
When quizzed on the trajectory of his work, Khullar hopes for even greater integration of ML/AI in financial services. "By 2030, we might see a world where credit decisions are made in milliseconds, all based on a vast ecosystem of interconnected data," he predicts. Industry forecasts seem to support this concept, as PwC estimates that ML/AI could contribute up to $15.7 trillion to the global economy by 2030, with financial services being a key beneficiary.
However, as the financial world marches toward this ML/AI-driven future, Khullar remains grounded in the human element of his work.
"At the end of the day," he reflects thoughtfully, "these models affect real people's lives. We must never lose sight of the individuals behind the data points."
In this digital age, the efforts of Khullar and other industry experts may well shape the very future of banking as a whole. Fortunately, with the focus kept on ethics and equitability, that future appears to be a hopeful one.