Researchers at the University of Texas at Austin have introduced a new framework to address the issues of artificial intelligence (AI) models mistakenly reproducing copyrighted images.
These AI models, including DALL-E, Midjourney, and Stable Diffusion, can create realistic images from textual descriptions.
However, these models have faced criticism and legal action from artists who allege that the AI-generated images closely replicate their original work.
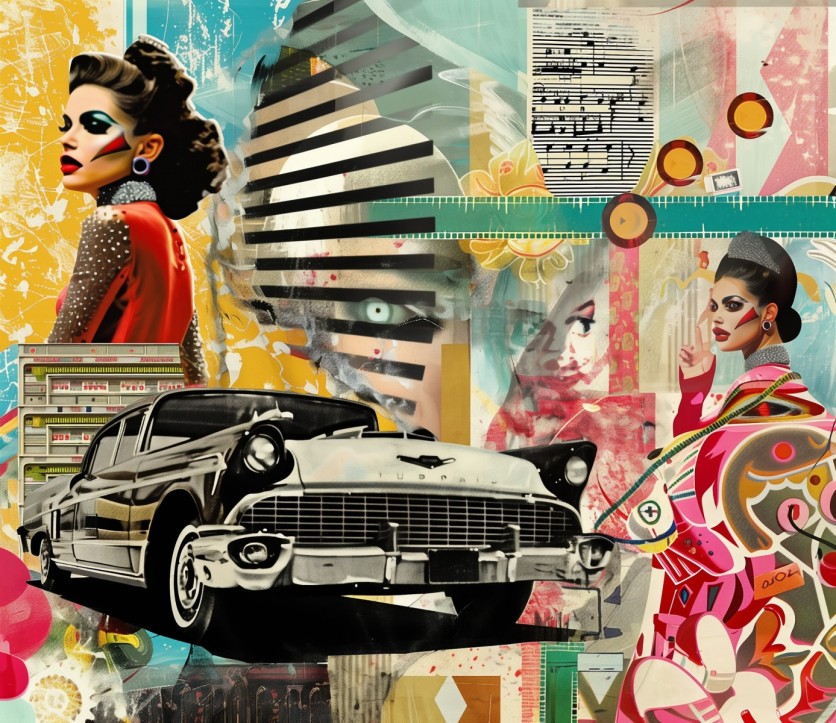
Introducing the Ambient Diffusion
The research team from UT Austin aims to resolve this by training AI models using corrupted images that do not resemble the original data.
The research introduces a framework called Ambient Diffusion, which trains AI models using images corrupted beyond recognition. The models are exposed to significantly altered data, making it impossible for them to memorize and replicate the original images.
This method allows the AI to draw inspiration from the corrupted data rather than copying it directly.
Training diffusion models with corrupted image data demonstrated the framework's effectiveness. Early results show that the AI can still generate high-quality images without having access to recognizable original images, according to the research team's claims.
This approach was first presented at the NeurIPS machine-learning conference in 2023. Since then, the framework has been further developed, leading to a follow-up paper titled "Consistent Diffusion Meets Tweedie."
The UT Austin team collaborated with Constantinos Daskalakis from the Massachusetts Institute of Technology (MIT) to expand the framework to include training models on larger datasets corrupted by various types of noise rather than merely masking pixels.
Beyond AI-generated Art
The new framework is a potential solution for scientific and medical applications where obtaining uncorrupted data is either costly or impossible.
Adam Klivans, a computer science professor involved in the research, emphasized the potential applications of this framework beyond the realm of AI-generated art. He highlighted its usefulness in scientific fields where uncorrupted data is hard to come by.
"The framework could prove useful for scientific and medical applications, too," Klivans said in an official statement.
"That would be true for basically any research where it is expensive or impossible to have a full set of uncorrupted data, from black hole imaging to certain types of MRI scans."
The researchers conducted experiments by training a diffusion model on a dataset of 3,000 celebrity images. Initially, the model trained on clean data blatantly copied the examples.
However, when the training data was corrupted by randomly masking up to 90% of individual pixels, the model's generated samples were high quality. Still, according to the team, they looked distinctly different from the training images.
The researchers noted that their framework controls the trade-off between memorization and performance. According to Giannis Daras, a computer science graduate student who led the work, increasing corruption during training decreases the model's tendency to memorize the training set, thus mitigating the risk of replicating original images.
The findings of the research team were published in arXiv.
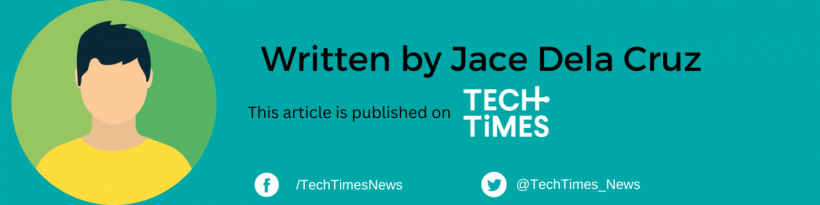