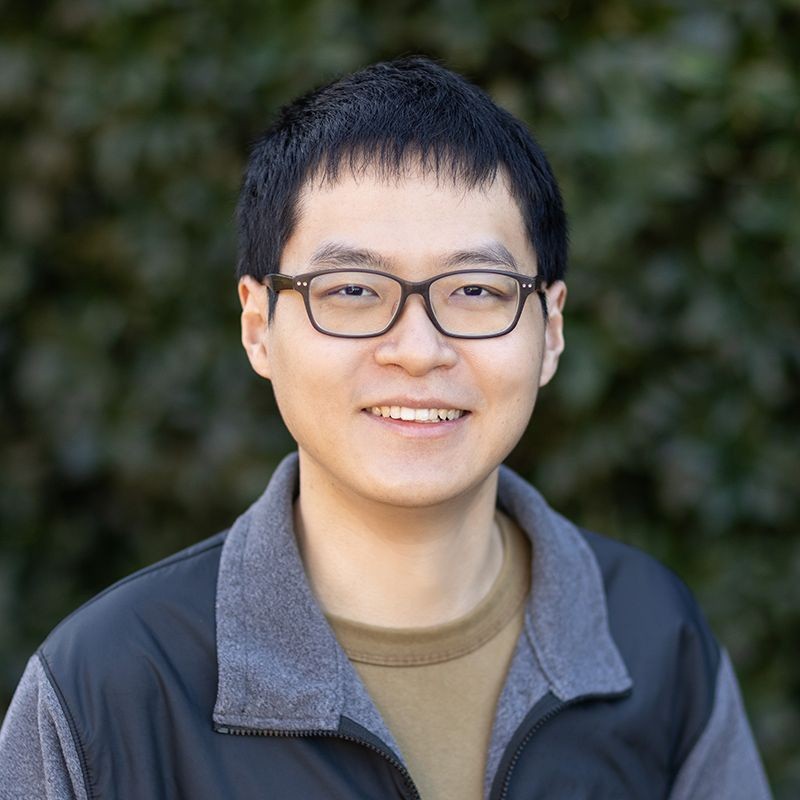
Every day, millions of vehicles navigate a complex network of roads. Traffic congestions, accidents, and stalled vehicles can quickly disrupt this flow, causing delays, frustrations, and even danger. Fortunately, surveillance cameras watch our roadways, providing valuable data for traffic management and public safety. But what if these systems could do more? What if they could identify unusual events and potential dangers lurking within the ordinary traffic flow?
According to the National Highway Traffic Safety Administration (NHTSA) crash statistics, over 19,000 people are estimated to have died in motor vehicle traffic crashes across the United States in just the first half of 2023. This data stresses the urgent need for improved road safety measures.
Traditional surveillance systems, while helpful, can only capture events after they unfold. Shining Yu, a software engineer with a background in computer vision, recognizes the limitations of traditional surveillance and is leading the development of a better, smarter system. At Panasonic R&D Center Singapore, Yu and his team developed an AI-driven solution by integrating anomaly detection in traffic surveillance videos. The AI algorithms can analyze traffic patterns and quickly identify unusual road events. Better identification allows authorities to be rapidly notified for quicker response. A monitoring that not only sees but also understands significantly transforming the roads to make every journey safer.
The Call for a Better Road Monitoring
Traditional surveillance systems were initially designed as a deterrent to criminal activity and a tool for post-event analysis. Video footage captured by these systems can be reviewed to investigate accidents, identify perpetrators of crime, and provide evidence for legal proceedings. While this purpose remains valuable, the limitations of these surveillance systems become apparent when considering a proactive response. These systems function primarily as recording devices, passively capturing footage without the inherent capability to analyze it in real-time and only providing information about incidents after they have already occurred. For instance, a monitoring device capturing footage of a car swerving erratically may have lost time responding to the situation when a human reviews the footage and identifies the danger.
Furthermore, traditional systems need more analytical capabilities to monitor extensive traffic networks effectively. They rely on human operators to sift through hours of video footage, a time-consuming and error-prone process. The creation of video review rooms equipped with multiple monitors and playback functionalities aimed to address this challenge. However, human fatigue and limitations in attention span can also lead operators to miss subtle anomalies in traffic patterns that might indicate an impending accident. The vast amount of video data these systems collect also presents a challenge. Manually reviewing all this data is impractical, making it likely that critical events will be missed or responses will be delayed.
With a keen eye for these limitations, Shining Yu focused on integrating anomaly detection into these surveillance systems—anomaly detection that will act as an attentive observer, constantly monitoring the traffic flow and keeping a watchful eye for anything that is out of the ordinary. By analyzing vast amounts of video data using deep learning algorithms, anomaly detection can identify patterns in traffic flow and flag deviations from these patterns. When the system detects an anomaly, such as stalled vehicles blocking lanes, it can trigger real-time alerts, notifying authorities and prompting a swift response to potential hazards. This proactiveness can significantly reduce the window of time between when an incident occurs and when help arrives, potentially saving lives.
Technology's Important Role in Addressing Enhanced Road Safety
At the heart of Yu's innovation lies the role of advanced technology. The critical aspects of this approach are the use of AI and a dual-mode framework inspired by the well-known two-stream network for understanding videos. This framework comprises two essential components—a slow and fast-mode stream.
The slow-mode stream detects static objects and environmental elements, such as road signs, traffic lights, and lane markings. This examination ensures a deep understanding of the contents in which vehicles are operating. On the other hand, fast-mode streams focus on capturing the dynamic movements of vehicles, such as acceleration and maneuvering.
By integrating data from both streams with deep learning models and predictive analytics, Yu's framework ensures that the movement of vehicles complies with the static elements present on the road. If a vehicle is speeding towards an unmoving object in the lane, like debris or roadblocks, it may infer a potential car crash that poses risks to the driver and other road users. The system promptly detects such anomalies and alerts authorities to take corrective action.
The Road Ahead: Better, Safer, Smarter
We can all agree that road safety is of the utmost importance, and Yu's innovation with anomaly detection in traffic surveillance transcends mere efficiency—it is a future where the roads no longer carry an undercurrent of fear.
His initiative paves the way for traffic management to become preventive, not reactive. Through this, the road we can look forward to is indeed better, safer, and smarter, thanks to the ingenuity of those like Yu, who dare to envision and build a future where every journey feels a little less stressful and a lot safer.