An AI-controlled drone system named "Swift" has reportedly achieved an impressive feat by outperforming three professional human drone pilots in a series of head-to-head races.
According to ScienceAlert, this autonomous system, developed by Elia Kaufmann, a robotics engineer at the University of Zurich, in collaboration with researchers at Intel Labs, demonstrated its superiority by winning 15 out of 25 races on a custom-designed racing track filled with challenging twists and turns.
AI-Controlled 'Swift' Drone System
The Swift system is the result of AI-learning algorithms, a single onboard camera, and sensors that enable it to detect its surroundings and movements.
Swift doesn't rely on external motion cameras for inputs, unlike its predecessors. Instead, it employs its onboard camera and inertial sensor to accurately gauge its acceleration and rotation, using this data to establish its position in relation to the gates on the racing course and generate precise control commands.
In comparison to drone-racing pilots relying on a camera-attached drone's perspective through headsets, Swift's AI algorithms process data to produce control commands, allowing it to navigate the track efficiently.
While it didn't emerge victorious in 40 percent of the races, Swift managed to surpass each human pilot on multiple occasions and even secured the fastest recorded race time, finishing half a second quicker than the best human attempt.
In their paper, Kaufmann and colleagues highlighted the challenges of creating an autonomous drone that can rival professional pilots.
They emphasized the need for the autonomous system to fly at its physical limits while simultaneously estimating its position and speed based solely on its onboard sensors. The researchers tackled these complexities by integrating AI algorithms that incorporated deep reinforcement learning.
This innovation lies in Swift's deployment of a second artificial neural network, which utilizes deep reinforcement learning. This approach allows the system to learn through trial and error during training, adapting its controls to real-world situations.
Much like its human counterparts, Swift underwent a simulation-based training regimen on the racing track. The deep learning algorithm enabled Swift to explore various routes through the track's gates, ultimately identifying the fastest paths.
Second Neural Network
Guido de Croon, a robotics researcher at the Delft University of Technology, lauded Swift's utilization of the second neural network and deep reinforcement learning.
He noted that while there are minor disparities between the simulation and reality, the neural network effectively learns from these differences, enhancing the simulation and refining the system's strategy.
Despite Swift's impressive performance, De Croon noted that further advancements are essential before the system can effectively tackle outdoor environments with unpredictable conditions.
Nonetheless, he expressed confidence that autonomous drones will eventually surpass human pilots even in challenging conditions due to drones' rapid data acquisition capabilities compared to humans' reliance on delayed images.
While Swift's success isn't the first instance of drones navigating obstacles, it stands out for its precision and potential for improvement. As the field of autonomous drones advances, Swift's achievements underscore the continuous evolution of AI-driven technology in various sectors.
The study detailing Swift's capabilities was recently published in the journal Nature.
Related Article : Archaeologists Discover Neolithic Cave Paintings in Spain Via Drones
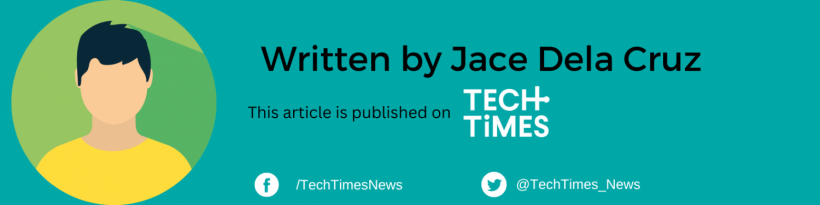