The role of large language models (LLMs) like ChatGPT in scientific research, particularly as potential co-authors of research papers, is a topic of debate and exploration in various industries.
While some funding agencies have raised concerns about the reliability of generative text AIs for peer review due to the reported inconsistencies in their analyses and the opacity of their training models, two professors from George Washington University view the evolving capabilities of LLMs with careful consideration.
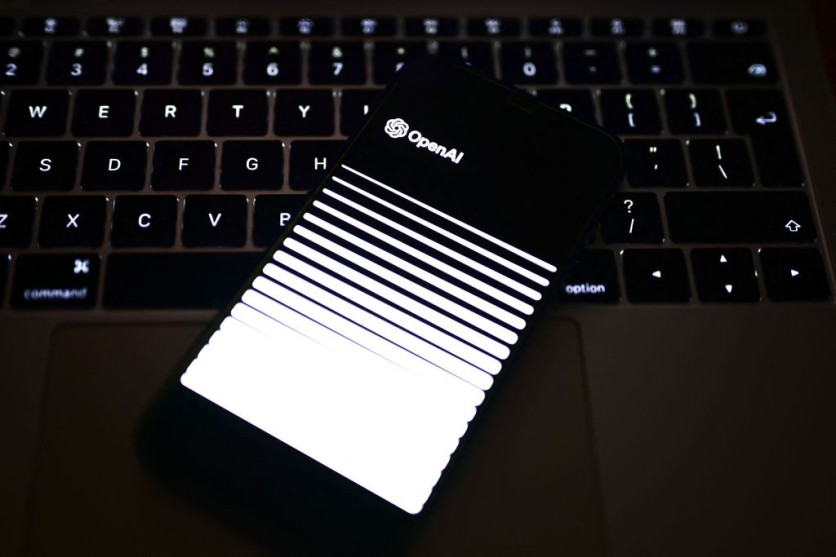
The Role of ChatGPT in Streamlining Scientific Processes
John Paul Helveston, an assistant professor of engineering management and systems engineering, and Ryan Watkins, a professor and director of the Educational Technology Leadership program, noted that LLMs, such as ChatGPT, could play a valuable role in streamlining scientific processes and enabling increased research output.
However, they emphasized the importance of proper education about the capabilities and limitations of these algorithms, as well as the existing norms and standards of AI use within scientific disciplines. Thus, they noted that ChatGPT cannot be a co-author in a scientific study, but it may help with the research.
The two professors managed an online repository called LLMs in Science, aimed at documenting and providing resources for scientists and educators interested in using LLMs as investigative tools. Helveston and Watkins recognized that LLMs are not merely data regurgitation systems.
These algorithms, which are trained on extensive datasets, can predict patterns and generate responses that mimic human-like language. ChatGPT-3, for instance, was trained on over 570GB of text data, equivalent to 300 billion words.
The professors pointed out that LLMs can be particularly useful in tasks that do not require human creativity or collaboration, such as producing boilerplate language, drafting grant proposals, and generating training data for analytical tools.
Their repository offers information on potential scientific applications of LLMs, tutorials, guidance for peer-reviewers, a database of LLM-related studies, and more.
Read Also : ChatGPT Enterprise: OpenAI's New Version of the Chatbot with Unlimited Access-For Business?
Limitations of LLMs
However, Helveston demonstrated the limitations of LLMs to his students. He illustrated how LLMs like ChatGPT struggle with accuracy when translating sentences or generating complex code. That highlights the necessity for understanding the language and context in which LLMs operate.
Watkins and Helveston noted that proper education can lead to better utilization of LLMs as learning tools, potentially enhancing student performance. Given the growing relevance of AI across various disciplines, Watkins suggested that including AI-related topics in syllabi is essential.
While concerns about academic integrity with LLMs have been raised, the professors maintain that when integrated appropriately into educational contexts, LLMs can empower students to enhance their learning experience.
The future role of LLMs in academia remains to be seen, as educators aim to harness their potential while equipping students with the critical skills to question and interpret the results generated by these tools.
"What we don't know yet is whether this will change how students learn," Helveston said in a statement. "We want to teach students to use these tools, because [AI is] the future of how certain tasks will get done. But we also want them to know how to question the results."
Related Article : Theoretical Physicists Call AI Chatbots Just 'Glorified Tape Recorders' as Fear of Artificial Intelligence Dies Down
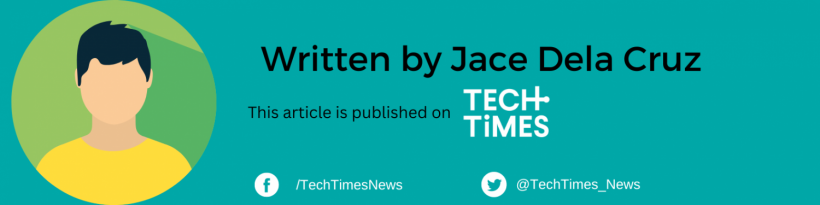