Strokes are a leading cause of death and disability worldwide, and prompt diagnosis and treatment are crucial to preventing devastating outcomes.
However, diagnosing strokes can be challenging due to the many conditions that mimic the symptoms, and misdiagnosis can lead to delayed treatment and long-term consequences.

Fortunately, researchers at Carnegie Mellon University (CMU), Florida International University (FIU), and Santa Clara University (SCU) have developed a groundbreaking new tool to accurately predict strokes using machine learning.
Their study reveals how this innovative model could revolutionize stroke diagnosis and treatment, particularly for underserved communities.
Predicting the Likelihood of Stroke
The model utilizes data collected during a patient's admission to forecast the likelihood of stroke. This prediction can be made without the need for diagnostic imaging or laboratory test results.
The algorithm integrates various variables such as age, gender, race, ethnicity, number of chronic illnesses, and primary payer.
It also considers the social determinants of health, which encompass the circumstances in which people are born, raised, and grow old in.
The researchers' model yielded impressive results, boasting an accuracy rate of 84% in predicting strokes. This rate surpasses that of existing scales, which often miss up to 30% of strokes.
The model's sensitivity is also notable, as it can detect strokes even when symptoms are mild or atypical.
In addition, the model can be used alongside existing stroke scoring systems employed in hospitals to rapidly prioritize patients for necessary intervention.
Potential Impact
The potential impact of this tool is significant, particularly in hospitals where there is a scarcity of medical resources and clinical staff.
By swiftly identifying patients who are at high risk of stroke and ensuring that they receive the appropriate care, the algorithm can be of immense help.
Furthermore, in emergency departments of low-volume or non-stroke centers and rural areas with limited access to sensitive diagnostic tools, this model can prove particularly useful.
"Machine learning methods have been used to help detect stroke by interpreting detailed data such as clinical notes and diagnostic imaging results," the study's lead author, Rema Padman, said in a statement.
"But such information may not be readily available when patients are initially triaged in hospital emergency departments, especially in rural and underserved communities."
Read Also : Scientists Achieve Sharpest-Ever Scan of a Brain-64 Million Times Clearer Than Ever Before!
Limitations of the Model
The study authors acknowledge several limitations of their research. As a retrospective study, confirmation of stroke cases was based on International Classification of Diseases codes rather than a thorough review of patients' medical records.
They also caution that while their algorithm showed promising results, it should not be regarded as a definitive method for diagnosing strokes. Instead, it should be viewed as a supplementary tool to the existing stroke scoring systems used in hospitals.
The study's conclusions were also limited by the unavailability of certain social determinants of health variables in administrative data, which may have influenced the algorithm's predictive accuracy.
The findings of the team were published in the Journal of Medical Internet Research.
Related Article : Biden Admin Proposes Update to Privacy Law, Blocking Access to Reproductive Health Information
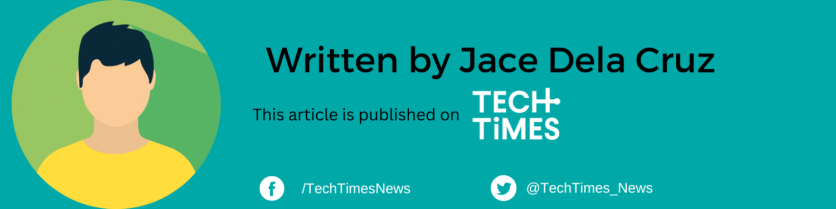