Artificial neural networks are a method that artificial intelligence utilizes to simulate how the human brain functions. A neural network "learns" from input from datasets and produces a forecast based on the available data.
But now, MIT Computer Science and Artificial Intelligence Lab (MIT CSAIL) researchers found a faster method to solve an equation that is employed in the algorithms for "liquid" neural neurons, according to a report by Interesting Engineering.
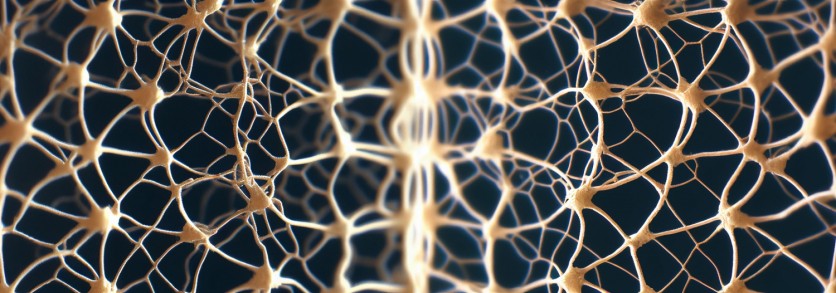
Liquid Neural Neurons
Researchers from MIT created liquid neural neurons last year, drawing inspiration from the brains of microscopic species.
It is described as "liquid" since the algorithm can modify the equations in response to fresh information, allowing it to adapt to changes encountered in real-world systems.
The researchers who developed the liquid neurons have found a technique to simplify the differential equations underlying the interaction of two neurons through synapses.
Differential equations make it possible to calculate the state of the world or a phenomenon across time as it develops step-by-step rather than from beginning to end, as noted by Interesting Engineering.
This gave them access to a new class of quicker artificial intelligence algorithms. Since they are adaptable and understandable, the modes have similar qualities to liquid neural nets, but what makes them novel is how much faster and more scalable they are.
The liquid neural network is a cutting-edge type of neural network that can change its behavior after reviewing input data. The research team discovered that the models were expensive as a result of the high number of synapses and neurons that were required to address the mathematical problems for the algorithms.
The magnitude of the equations made the math problems more challenging to solve, frequently taking numerous computing steps to arrive at a solution and obtain an answer.
Closed-Form Continuous-Time
The "closed-form continuous-time" (CfC) neural network is the name of the new network. In terms of making predictions and finishing tasks, it has surpassed other forms of artificial neural networks, according to the team.
It also performs faster and with better accuracy when identifying human activities from motion sensors, simulating the physical dynamics of a walker robot, and other tasks.
When 8,000 patients were sampled for medical predictions, the new prototypes outperformed their equivalents by 220 times.
The team claims that there is evidence that "liquid" CfC models can acquire activities in one environment and then transfer those skills and capacities, without additional training, to a completely different one.
To handle bigger problems, this would involve creating neural networks on a wider scale. This framework should serve as the fundamental step for future intelligence systems since it can aid in the resolution of more challenging machine learning tasks, improving representation learning, as per the team.
The researchers believe that by accelerating the output process, safety commercial and defense systems can one day benefit from computational efficiency.
Related Article : MIT Says NASA James Webb May Be Too Advanced; Experts Claim Data Misinterpretation Might Happen
This article is owned by Tech Times
Written by Jace Dela Cruz