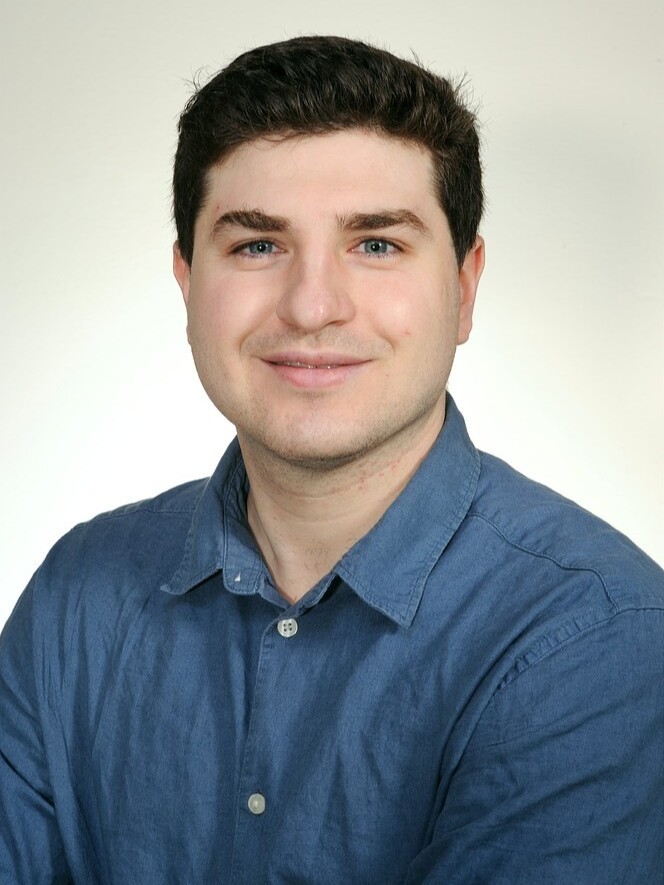
In today's artificial intelligence (AI) systems, everything depends on how machines understand data.
But beneath the buzzwords lies a quiet challenge: How do you know your AI system is aligned with your goals? The thought space of the models and internal representations often goes unchecked and unexplained. For Simon Reisch, that problem is both a technical flaw and an opportunity for innovation.
Reisch is the co-founder of Tessel, a company rethinking how people interact with AI models today. "Most companies treat models like fixed black-box systems," he says. "But we can do so much more, starting with understanding model behavior based on hypothesis-driven testing and remediating urgent issues. Right now, AI users don't have the right tools to make the right decisions."
The Importance of Rigorous Evaluation in ML Models
Born and raised in Germany, Reisch studied Computer Science at Karlsruhe Institute of Technology and Stanford, publishing research at leading AI conferences like NeurIPS. Throughout his academic journey, he encountered a critical gap: AI systems rely on internal representations whose inner workings are not considered when evaluating outcomes.
If a model mislabels a benign tumor as malignant, it's not enough to just note the mistake—understanding why it happened is needed. "Without knowing the cause," Reisch explains, "you can't tell whether it's an isolated failure or a systemic flaw that could impact similar cases."
Founding Tessel: Aligning AI with Real-World Business Goals
The reason why rigorous evaluation matters goes beyond academic curiosity is that it directly impacts business outcomes. Companies deploy AI to solve concrete problems: improving medical diagnosis accuracy, increasing conversion rates, reducing financial risk, or optimizing manufacturing processes. Yet, without a clear understanding of how and why an AI system reaches a given decision, businesses risk misaligned predictions and costly mistakes.
Reisch emphasizes this point clearly: "Understanding why a model behaves a certain way allows teams to connect AI performance directly to their most important business metrics. If your AI predicts cancer incorrectly, that's not just a statistical error—it's a patient's health at stake. Rigorous evaluation and debugging ensures your AI decisions are trustworthy and aligned precisely with what matters most to your business."
This is why Tessel moves beyond traditional research, bridging the gap between model behavior and measurable business outcomes. It provides companies with tools to clearly identify, debug, and align their models, making AI not just theoretically robust but practically reliable in the real world.
The Vision Ahead
Tessel's vision is to provide businesses with an evaluation and remediation platform that ensures model internals are aligned perfectly with your use case. Whether you're using ML models to perform cancer classification or legal document searches, you want to have a model that represents information in the best way for your goals.
Rather than treating model behavior as a black box, Tessel turns it into a structured feedback engine, empowering teams to build, test, and evolve AI systems with confidence.
For more on Simon Reisch and Tessel's work, visit Tessel or connect via LinkedIn.