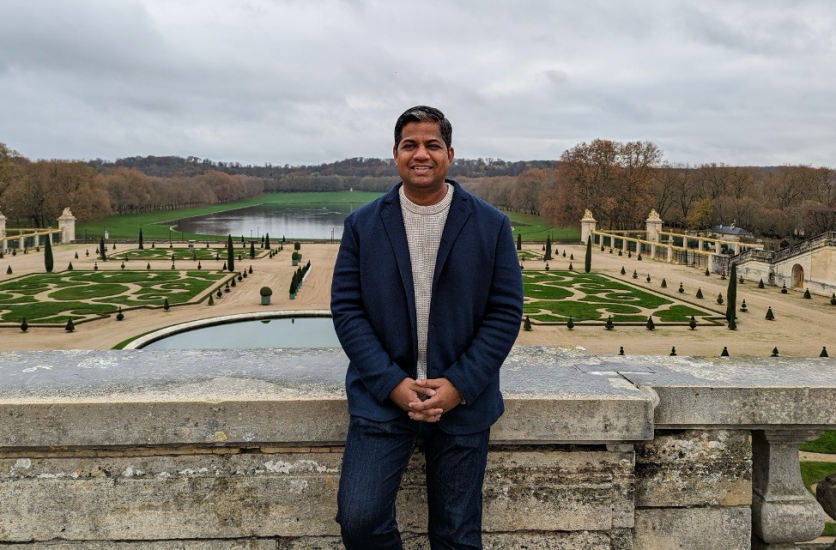
Businesses are racing to harness sprawling data across multiple cloud platforms. Ashitosh Chitnis stands at the forefront of this movement—an architect of scalable, enterprise-grade data solutions with deep expertise spanning Google BigQuery, AWS, SAP's public cloud ecosystem, Snowflake, and Databricks.
Over a 16+ year career, he has navigated the evolution from traditional data warehouses to cutting-edge data lakes, lakehouses, and data meshes. The result is a unique mastery of multi-cloud and modern data architectures that enable organizations to unlock value from their data in unprecedented ways.
A seasoned data professional, Chitnis has successfully architected and implemented petabyte-scale data warehouses, powered business intelligence platforms, and delivered pure-play analytics projects—all in service of uncovering hidden data insights and driving organizational success.
His focus areas span AI/ML, advanced analytics, data engineering, visualization, and solution architecture, reflecting a holistic approach to enterprise data strategy. Industry recognition has followed: Chitnis was recently honored with an Outstanding Technical Innovation award for his leadership in Advanced Analytics and AI/ML. He has also served as a Globee Awards judge, evaluating global business and technology innovations.
This feature article explores Chitnis's journey and vision through eight themes, from multi-cloud strategy to the rise of data mesh, drawing on his insights and the latest research in the field.
In an era when only 32% of companies realize tangible value from their data and just 3% of data meets basic quality standards, Chitnis's story offers a roadmap for leveraging modern architectures to close this "data-value gap."
A Journey Through Data-Driven Giants
Chitnis's career path has been defined by diverse experiences at some of the world's top technology and consulting organizations. "Driven by a love of data and its potential to influence choices and corporate development, my path started," he recalls, emphasizing that an early passion for data guided his professional direction.
Beginning in the consulting realm, he honed his skills in delivering business intelligence solutions for enterprise clients before moving on to lead major analytics initiatives at IBM. "Leading large-scale analytics initiatives across sectors at IBM helped me to first experience different corporate demands and how data may be leveraged to meet them," Chitnis notes, highlighting how working with varied industries taught him to tailor data strategies to unique business needs.
This broad exposure proved invaluable as he progressed to roles at Google and, eventually, Apple.
Each stint added a new dimension to his expertise. At Google, Chitnis focused on operationalizing machine learning at scale. "My job at Google was mostly about productionizing machine learning solutions utilizing Vertex AI, mostly for financial regulations, thereby driving efficiency and trust in enterprise financial operations," he says, referencing how he deployed Google Cloud's Vertex AI platform to automate and scale financial analytics.
This experience in a cloud-native, AI-driven environment would later inform his multi-cloud strategies. By the time Chitnis joined Apple as a software engineer, he had become adept in blending data engineering and AI to build robust systems.
His tenure across Apple, Google, IBM, and Deloitte (among others) has equipped him with a 360-degree view of enterprise data challenges and solutions. It's a journey that reflects not only technical growth but a deepening understanding that technology must ultimately serve business outcomes.
Enterprise leaders increasingly recognize that simply accumulating data isn't enough—it must be translated into actionable intelligence. Chitnis's multifaceted background exemplifies the kind of cross-domain expertise required to ignite data-driven transformation, as he did in each of his roles.
Motivation for a New Data Paradigm
Having witnessed the shortcomings of traditional data management up close, Chitnis was inspired to champion a radically new approach to enterprise analytics. "Over my career, I saw consistent problems with centralized data management—lack of agility, segregated insights, and delayed decision-making," he explains.
In large organizations, data was often siloed in monolithic warehouses or ERP systems, making it difficult to get timely, holistic insights. Changes were slow, and advanced analytics initiatives (like machine learning pilots) struggled to make an impact in these rigid environments. This realization set the stage for Chitnis's exploration of emerging paradigms that could address these pain points.
His answer came in the form of data mesh—an up-and-coming architectural philosophy that decentralizes data ownership. "Data Mesh changes how companies handle data management by seeing data as a product with distributed ownership," Chitnis says.
Instead of funneling all data into one central team or platform, a data mesh empowers individual business domains (such as finance, marketing, or supply chain) to own and serve their data as products for the rest of the organization.
This approach promised to solve the agility and bottleneck issues he had encountered by aligning data closer to those who know it best. Chitnis was so convinced by this paradigm that he decided to share his knowledge by authoring a book, AI-Powered Data Mesh for SAP: Engineering the Future of Enterprise Analytics.
In it, he lays out a blueprint for blending data mesh principles with artificial intelligence in SAP-centric enterprises—a bold response to the "consistent problems" he observed.
Research shows that the timing could not be more apt. Enterprises today manage ever-growing volumes of data (the global datasphere is expected to double to 181 zettabytes by 2025) and struggle with centralized models—as evidenced by only 32% of firms managing to get value from their data.
Traditional data warehouses often become bottlenecks that "create real friction for organizations that need to discover, understand, and leverage data." By contrast, treating data as a product in a distributed mesh can increase agility and accountability.
Chitnis's angle, combining this mesh concept with AI/ML, positions organizations to leapfrog into an era of intelligent, adaptive data infrastructure. It is about building systems that not only gather data but continuously learn and improve from it.
Gartner analysts have noted that 85% of AI projects fail due to poor data quality or lack of relevant data—a sobering statistic that underscores why Chitnis's focus on a new data paradigm is so critical. He tackles the root of the issue: the way data is organized and owned in the enterprise.
Decentralizing Data with Mesh and Domain Ownership
For Chitnis, data mesh is more than a technical architecture—it represents a cultural shift in how companies think about data. "It's not just a tech framework but a new mindset. Data mesh encourages organizations to treat data less as an IT byproduct and more as a strategic asset owned by the business," he explains.
This philosophy stands in contrast to the legacy view of data as something generated and handled by back-office IT systems. In a mesh, cross-functional domain teams (composed of data engineers, analysts, and subject matter experts in a business area) take end-to-end responsibility for their data pipelines and outputs.
Each domain publishes its data in a usable form (often through APIs or data products) for others to consume under a federated governance model. This domain-oriented ownership ensures that data products are closer to the business context and inherently aligned with business needs.
Chitnis emphasizes that this approach directly addresses the agility and silo issues he saw in the past. "For SAP-based systems, this change directly empowers domain specialists, hence enhancing agility, responsiveness, and insight quality," he notes.
In many enterprises (especially those running large ERP systems like SAP), data has historically been locked in module-specific silos managed by central IT. By implementing a data mesh, these companies can decentralize control to the specialists in each domain (for example, allowing the finance department to manage and innovate on finance data on their terms while still adhering to overall governance).
The result, Chitnis observes, is faster turn-around on analytics requests and more relevant insights—because the people who understand the data deeply are the ones in charge of shaping it.
Notably, Chitnis's focus area of SAP public cloud technologies ties into this narrative. SAP's latest data management offerings (such as SAP Data Warehouse Cloud and SAP Datasphere) are themselves moving toward a more open, federated model, which complements data mesh principles.
By applying a data mesh in an SAP environment, Chitnis essentially bridges modern architecture with a legacy-rich ecosystem. Industry experts agree that data mesh can work in harmony with technologies like data lakes and data warehouses—it's an overarching approach rather than a single product.
Companies can still use a data lakehouse (which combines a data lake's flexibility with data warehouse features) within each domain, for instance. The key difference is who manages the data and how it's shared.
Chitnis's implementations often entail creating domain-specific data repositories (be it a lake, lakehouse, or traditional warehouse) and then linking them via a mesh of data products and unified governance policies.
This way, enterprises get the best of both worlds: decentralization for speed and context and central oversight for standards and security.
This decentralized model requires a robust governance layer—something Chitnis does not overlook. He advocates for federated governance, where a central team sets guardrails (like data definitions, access policies, and privacy rules), but domain teams have the freedom to operate within those guardrails.
As one engineering overview puts it, a successful data mesh "requires organizational changes like new team structures" and strong coordination to keep the mesh from devolving into chaos. Chitnis's dual focus on architecture and solution governance reflects this balance.
Harnessing AI/ML and Advanced Analytics
A crucial element of Chitnis's vision is weaving artificial intelligence and machine learning into the fabric of modern data architectures. In his view, AI/ML should not be an afterthought or a siloed initiative; it needs to be deeply integrated with the data pipeline. His time at Google provided a clear demonstration of this integration.
"My job at Google was mostly about productionizing machine learning solutions utilizing Vertex AI, mostly for financial regulations, thereby driving efficiency and trust in enterprise financial operations," he says. By embedding ML models into Google's data ecosystem, Chitnis was able to automate complex tasks like detecting regulatory compliance issues in finance data, turning what used to be manual audits into continuous, real-time checks.
This experience underscores how advanced analytics and AI can deliver value when they are operationalized—meaning developed, deployed, and maintained as part of the regular business process rather than as one-off experiments.
In Chitnis's projects since then, he has repeatedly leveraged AI to solve thorny data problems. One striking example involved financial transaction data at a large enterprise. "We leveraged AI/ML to develop a data clustering solution to find duplicate payments, saving approximately $20 million annually," he shares.
In this case, machine learning algorithms were used to cluster and compare payment records, flagging potential duplicates or overpayments far more accurately and quickly than traditional rule-based methods. The impact was not only significant cost savings but also the establishment of trust in AI-driven insights—a big win in an industry often cautious about new technology.
Chitnis notes that achieving such results requires more than just training a model in a lab. The solution had to be integrated with the company's payment systems and workflows (so that identified duplicates could be reviewed and acted upon), and it needed governance to handle exceptions and ensure that false positives were minimal.
Another key aspect of tying AI into the data pipeline is data quality management. Chitnis's research work has shown that manual data quality checks struggle at the scale and speed of modern data streams. Instead, he advocates for ML-driven data observability—algorithms that continuously monitor data for anomalies, schema changes, or quality issues.
By automating these checks, organizations can maintain high data reliability even as they ingest terabytes of raw data daily. "Data quality and governance aren't afterthoughts—they're foundational," Chitnis insists, echoing this philosophy. In practice, this means every AI/ML deployment he leads includes robust validation steps.
For instance, an ML model's output might feed into a dashboard only after passing certain accuracy thresholds, or an alert is generated if input data drifts beyond the model's expected range (triggering a retraining workflow). This disciplined approach is crucial because AI is only as good as the data feeding it.
The payoff for integrating AI and advanced analytics in this manner is transformative. Companies move from reactive reporting to proactive prediction. Chitnis has helped clients implement predictive maintenance models on streaming IoT data, real-time fraud detection on multi-cloud transaction flows, and demand forecasting using deep learning—all riding on top of modern data architectures.
Such initiatives exemplify advanced analytics in action, where insights are not just descriptive (what happened) but predictive (what's likely to happen) or even prescriptive (what we should do about it). By infusing AI into each layer of the data stack, Chitnis enables enterprises not only to derive insights faster but also to embed intelligence into their daily operations.
It's worth noting that many organizations struggle to get AI projects past the experimental stage—an issue often dubbed "pilot purgatory." In 2024, an O'Reilly survey found that only 26% of AI initiatives had reached production deployment. Chitnis's work offers a counterpoint to that trend, demonstrating how a strong data engineering foundation can dramatically increase AI's success rate.
His insistence on automation, model governance, and alignment with business processes addresses the common pitfalls that cause AI projects to stall. In effect, he bridges the gap between data science and data engineering—a bridge that is critical for AI to deliver real-world value.
Multi-cloud Data Strategy and Governance
Modern enterprises rarely confine themselves to a single cloud vendor. One of Chitnis's specialties is designing data architectures that span multiple cloud platforms—an approach known as multi-cloud.
He understands that different clouds offer different strengths: Google Cloud might excel at analytics, AWS at versatile infrastructure, Azure at seamless Microsoft integration, and so on. By leveraging the best of each, organizations can optimize performance and avoid vendor lock-in.
However, Chitnis is also keenly aware of the challenges that come with this flexibility. "Multi-cloud setups offer great flexibility, but they also bring complexity in governance and security," he points out. Each cloud environment has its services, APIs, and quirks, which can lead to a fragmented ecosystem if not managed properly.
For example, ensuring consistent access controls and audit trails across AWS S3, Google Cloud Storage, and Azure Blob Storage is non-trivial, yet essential in a multi-cloud data lake strategy.
To mitigate these issues, Chitnis emphasizes a strong governance framework from the outset. "You need robust frameworks to ensure data is consistent, secure, and compliant across cloud environments," he advises. In practice, this might involve implementing a unified data catalog and governance tool that covers all data assets, whether they reside on-premises or in GCP, AWS, or any other cloud.
It also means abstracting certain layers of the data architecture. For instance, Chitnis often employs cloud-agnostic technologies (like Apache Spark or Kubernetes) and SaaS solutions (like Snowflake) that can operate on multiple clouds, thereby providing a consistent platform.
Snowflake is a good example—it's a cloud-based data warehouse that runs on AWS, Azure, or GCP with a uniform experience, which Chitnis has used to create a single source of truth accessible from anywhere. Similarly, tools for orchestration and monitoring (such as Terraform for infrastructure-as-code or Tableau/Looker for BI) are chosen for their multi-cloud compatibility.
Security and compliance are front and center in his multi-cloud designs. Data sovereignty regulations (like GDPR or country-specific data residency laws) add another layer of complexity.
Chitnis notes that when data flows between clouds and regions, one must carefully enforce policies so that sensitive data stays within allowed boundaries—an issue he's explored in his writings on data sovereignty in AI systems.
Encryption, tokenization, and federated identity management are some of the techniques he employs to maintain uniform security. An illustrative scenario he tackled involved a client in the financial sector operating on both AWS and Google Cloud: by implementing a centralized identity provider and uniform encryption standards, Chitnis helped them ensure that customer data was protected to the same degree on both platforms, satisfying audit requirements.
Industry research confirms the importance of these measures. Multi-cloud environments can introduce visibility gaps and inconsistent controls, making governance "multiply" in difficulty. Common challenges include differences in cloud vendor security settings, variances in service-level agreements, and the complexity of tracking costs across services.
Chitnis addresses these by designing for observability—for example, deploying monitoring agents and log aggregators that collect metrics from all clouds into a single dashboard. This way, operations teams get a holistic view of the entire pipeline's health and can detect issues like data latency or job failures regardless of where they occur.
Crucially, Chitnis approaches multi-cloud not as an end in itself but as a means to serve business needs. If a particular workload runs best on a certain cloud service, he'll integrate it, but always with an eye toward the big picture.
This pragmatic approach means some systems end up hybrid (mix of on-prem and cloud) or multi-cloud only where it makes sense. The guiding principle he imparts is to avoid siloing by the cloud. Data silos can just as easily form by cloud platform as they did by department.
Through consistent data models and governance across clouds, Chitnis ensures that, for example, a sales analytics dashboard can seamlessly combine CRM data from an Azure database with marketing data from a Google BigQuery lake—the multi-cloud nature is invisible to the end user.
The result is a flexible yet controlled environment where enterprises can innovate quickly using whichever cloud tools are optimal, all under a unified data strategy.
The benefits of multi-cloud are evident in resilience and choice, but without proper strategy, costs and risks can escalate. Chitnis's methodologies align with best practices experts recommend: standardize policies and design for portability.
His work serves as a case study of achieving the promise of a multi-cloud while taming its complexity.
Architecting Scalable, Enterprise-Grade Solutions
At the core of Chitnis's skill set is solution architecture—the ability to design data systems that are not only innovative but also scalable, reliable, and maintainable. One of his trademarks is selecting the right tool for the right job in a technology landscape that is constantly evolving.
"I've utilized platforms like Google BigQuery, AWS, Snowflake, and Databricks to architect solutions—each tool has its place when building an enterprise-grade data pipeline," Chitnis says.
Indeed, his projects often involve a heterogeneous mix of technologies: he might use BigQuery or Amazon Redshift as a data warehouse for its analytical query power, Databricks for data lake processing with Apache Spark, SAP HANA or SAP Data Warehouse Cloud for integrating with ERP data, and Snowflake as a scalable, cross-cloud analytics engine.
By knitting these together, Chitnis creates a data architecture that can ingest raw data, curate it into clean, structured forms, and deliver it to analytics and AI applications swiftly.
Scalability is a recurring theme in Chitnis's designs. He has worked on data pipelines that handle petabytes of data and millions of records per second. To achieve this, he leverages cloud-native features like auto-scaling, distributed computing, and serverless processing where appropriate.
For example, when building a petabyte-scale data lakehouse for an analytics project, he combined the storage durability of Amazon S3 with the distributed query engine of Databricks Spark, ensuring the system could grow horizontally as data volumes exploded.
In another instance, to support a high-concurrency reporting system, he used Google BigQuery's ability to handle large numbers of simultaneous queries without performance degradation. These choices illustrate a key aspect of his architectural philosophy: design for the "10x" growth.
Chitnis often asks, "If data and usage increased tenfold, what breaks?" and then addresses that in the design—be it through partitioning data, caching hot data, or choosing a more elastic service.
Another critical factor is reliability. Enterprise-grade solutions must be resilient to failures and ensure data accuracy. Chitnis incorporates concepts like idempotent data pipelines (so re-running them yields the same result, preventing duplicates), checkpointing and watermarks for streaming jobs (to handle interruptions without data loss), and thorough testing/monitoring.
As he puts it, "Data quality and governance aren't afterthoughts—they're foundational. We automated checks and validations in our pipelines to ensure trust in the data at every step." This means that at each stage of the data flow—from extraction and transformation to loading—there are validation rules.
For instance, a job that aggregates sales data might cross-verify totals against source systems; if a discrepancy beyond a threshold is found, the system flags it for investigation before it propagates to dashboards.
Chitnis also designs with maintainability and evolution in mind. He is a proponent of modular architecture—breaking the system into independent components (ingestion, processing, storage, serving) connected by well-defined interfaces.
This modularity paid off when one client decided to swap their visualization tool from Tableau to Power BI; because the data marts were exposed via standard SQL and APIs, the change was relatively smooth without overhauling the whole pipeline.
Similarly, when cloud services update or new ones emerge, an architecture with loosely coupled components can integrate improvements without a complete rewrite.
A testament to Chitnis's architectural acumen is how his solutions have remained in production, delivering value over the years. The systems he built at one company continued to scale with their growth from a few hundred gigabytes of data to many terabytes with minimal refactoring.
Such longevity is rare in an industry where platforms often buckle under the strain of new demands. It stems from foresight in anticipating future needs and a relentless focus on engineering best practices.
Chitnis encourages documentation of data lineage, clear data contracts between producers and consumers, and the use of CI/CD (continuous integration/continuous deployment) for analytics code—practices that bring software discipline to data engineering.
From Data to Insight: Visualization and Culture
Even the most sophisticated data architecture must ultimately serve the people who use data to make decisions. Chitnis fully appreciates this, placing a strong emphasis on data visualization and user adoption in his projects.
"The most advanced data platform means little if end users can't interpret the results. We rely on visualization tools to turn data into intuitive dashboards that drive informed decisions," he remarks.
In practice, this has meant deploying business intelligence (BI) and analytics tools like Tableau, Looker, or Power BI on top of the data infrastructure he builds. After all, a well-structured data lake or warehouse only becomes valuable when decision-makers can easily query it and understand the outcomes.
Chitnis often works closely with analysts and business stakeholders to design dashboards that highlight key performance indicators, trends, and outliers in visually compelling ways. For example, at one company, he enabled a marketing analytics dashboard that pulled cleaned data from a Snowflake warehouse and visualized customer acquisition metrics by region in real time, allowing regional managers to spot dips or spikes at a glance and react quickly.
A great visualization not only displays data but also tells a story. Chitnis encourages the use of clear charts, graphs, and even AI-driven natural language summaries to make insights accessible.
This approach has measurable benefits: studies have shown that effective data visualization "speeds decision-making and enhances cross-stakeholder collaboration." One example from Chitnis's work involved an executive dashboard for a supply chain operation.
By condensing thousands of data points into a simple set of gauges and color-coded indicators (green, yellow, and red status lights), the dashboard conveyed the overall health of the supply chain at a glance.
Executives could see where bottlenecks were emerging (red lights) and drill down into interactive charts for details rather than wading through spreadsheets. This not only saved time but also aligned different departments (procurement, manufacturing, logistics) around a single source of truth.
Beyond tools and charts, Chitnis is a champion of cultivating a data-driven culture. "Fostering a data-driven culture is as important as the tech. We work closely with business teams to ensure they trust and understand the data, making analytics part of their day-to-day decision process," he says.
In practical terms, this means he often leads user training sessions, creates data literacy programs, and sets up "analytics communities" within organizations. For instance, he might establish a weekly forum where analysts and data scientists demo new insights or use cases to business users, creating excitement and buy-in.
He also advocates for self-service analytics, empowering users to explore data on their own (within governed limits). By providing user-friendly semantic layers or data catalogs, Chitnis helps non-technical users find the data they need without writing complex SQL.
This democratization of data is a key step toward a truly data-driven enterprise.
Another aspect of building trust is ensuring data accuracy and transparency. If end users are going to rely on a dashboard for critical decisions, they need confidence in the numbers.
Chitnis addresses this by implementing explanation and lineage features—for example, adding footnotes or tooltips on dashboards that describe how a figure was calculated or enabling drill-throughs to underlying data sources. In one case, after rolling out a new
In an AI-powered sales forecasting tool, he included an "explainability" module that allowed sales managers to see the top factors influencing each forecast (like price changes or seasonal effects). This level of transparency helped users accept the AI insights rather than view them as a black box.
It reflects Chitnis's understanding that human factors (like trust and clarity) are as crucial as technical performance.
The outcome of prioritizing visualization and culture is evident in adoption metrics: systems architected by Chitnis often see high utilization rates. Instead of gathering dust, the data platforms become central to daily operations.
At a retail client, the analytics portal he built became the second most accessed internal web application after email, a sign that employees from store managers to C-suite were engaging with data regularly.
Data visualization turned abstract figures into concrete action items—something as simple as a well-designed chart showing a dip in customer satisfaction could trigger a store manager to retrain staff or adjust inventory, closing the loop between data and action.
This alignment of people, processes, and technology is frequently cited as the hallmark of analytical maturity. Harvard Business Review has noted that a data-driven culture—where employees ask questions of data and seek evidence for decisions—distinguishes top-performing companies.
By combining technological solutions with education and engagement, Chitnis effectively nurtures such a culture. In doing so, he ensures that the multi-cloud, AI-infused architectures he builds truly translate into business value on the ground.
Future Outlook and Final Thoughts
As someone at the cutting edge of data engineering and analytics, Chitnis maintains a forward-looking perspective on where the industry is headed. He sees a convergence of trends pushing towards smarter and more automated data ecosystems.
"In the coming years, I see data architectures becoming even more automated and intelligent—from self-healing data pipelines to real-time AI-driven insights," Chitnis predicts. Indeed, the rise of AIops and automation in data management is already underway. We can expect systems that automatically detect and fix data pipeline issues or analytics platforms that proactively surface insights without being asked.
Chitnis is particularly excited about the potential of real-time streaming analytics combined with AI, enabling use cases like instantaneous fraud detection across multiple clouds or dynamic supply chain optimizations based on live data.
He also mentions the growing importance of data mesh and data fabric concepts in a world of distributed data and how they will evolve with new tooling that makes implementation easier (perhaps using knowledge graphs or advanced metadata management to tie everything together).
Another future trend Chitnis highlights is the integration of generative AI and natural language interfaces with data systems. The idea that business users could simply ask a question in natural language and the system (powered by large language models) would generate the answer by querying the data is becoming a reality. This could further democratize data access.
However, Chitnis cautions that the fundamentals will remain crucial. In his words, "Don't adopt technology for its own sake. Build a strong data foundation, invest in talent and governance, and always tie data initiatives to business outcomes."
This advice is a reminder that buzzwords alone don't create value—sound data management practices do. As organizations clamor to implement the latest AI tool or analytics platform, Chitnis stresses the importance of strategy and alignment.
For professionals entering this field or companies embarking on transformation, Chitnis offers sage guidance. He encourages continuous learning given how fast the technology landscape changes.
What's cutting-edge today (like a new cloud service or ML library) might be outdated in a few years, so adaptability is key. Yet, he also notes that certain skills remain evergreen: SQL, data modeling, problem-solving, and communication.
On an organizational level, he advocates for incremental progress—proving value with small wins (such as an AI model that improves one process) and then scaling up, rather than trying to do a "big bang" overhaul. This agile, iterative approach often yields better adoption and less risk.
Chitnis's career trajectory—from building traditional BI solutions to pioneering AI-powered data meshes—exemplifies the blend of enduring principles and innovative thinking that drives success in data initiatives.
Advanced analytics and AI will only grow more influential in business, and those who can bridge the gap between complex technology and real-world application (as Chitnis does) will lead the way.
He believes the next frontier might also involve ethical AI and data governance becoming more prominent, ensuring that as we gain more power from data, we also handle it responsibly with regard to privacy and fairness.
In all, Chitnis remains optimistic: the tools are improving, awareness of data's value is higher than ever, and the next generation of data architectures promises to be more flexible and powerful. With leaders like him at the helm, enterprises are well-positioned to navigate this exciting future where data truly drives decisions at every level.
As organizations continue on their data-driven journeys, Chitnis's story serves as both inspiration and blueprint. It underscores that mastering multi-cloud architecture, embracing modern paradigms like data mesh, and integrating AI throughout can unlock transformative outcomes—but only if guided by a clear vision, solid engineering, and a focus on people.
This balanced approach is exactly what will carry enterprises through the next wave of digital transformation.
Chitnis's experience and insights paint the portrait of a data architect who is equal parts visionary and pragmatist. He has demonstrated how multi-cloud data ecosystems can be harnessed for agility, how data meshes and modern architectures can overcome longstanding bottlenecks, and how AI/ML can be embedded into enterprise DNA to yield proactive intelligence.
Throughout his 17-year journey, from IBM and Google to Apple, Chitnis has championed the idea that data solutions must scale technically and resonate with people culturally. The result is a track record of data platforms that have delivered business value—whether by saving millions through analytics, accelerating decision cycles with real-time dashboards, or enabling new AI capabilities in a governed, reliable manner.
His story offers a roadmap for organizations looking to navigate the complex, multi-cloud world and emerge as truly data-driven enterprises. In the end, Chitnis's work is about more than technology—it's about empowering organizations to leverage their data assets to the fullest, fostering innovation while maintaining rigor.
That ethos of mastering modern data architecture to drive meaningful outcomes is the legacy he continues to build, one solution at a time.