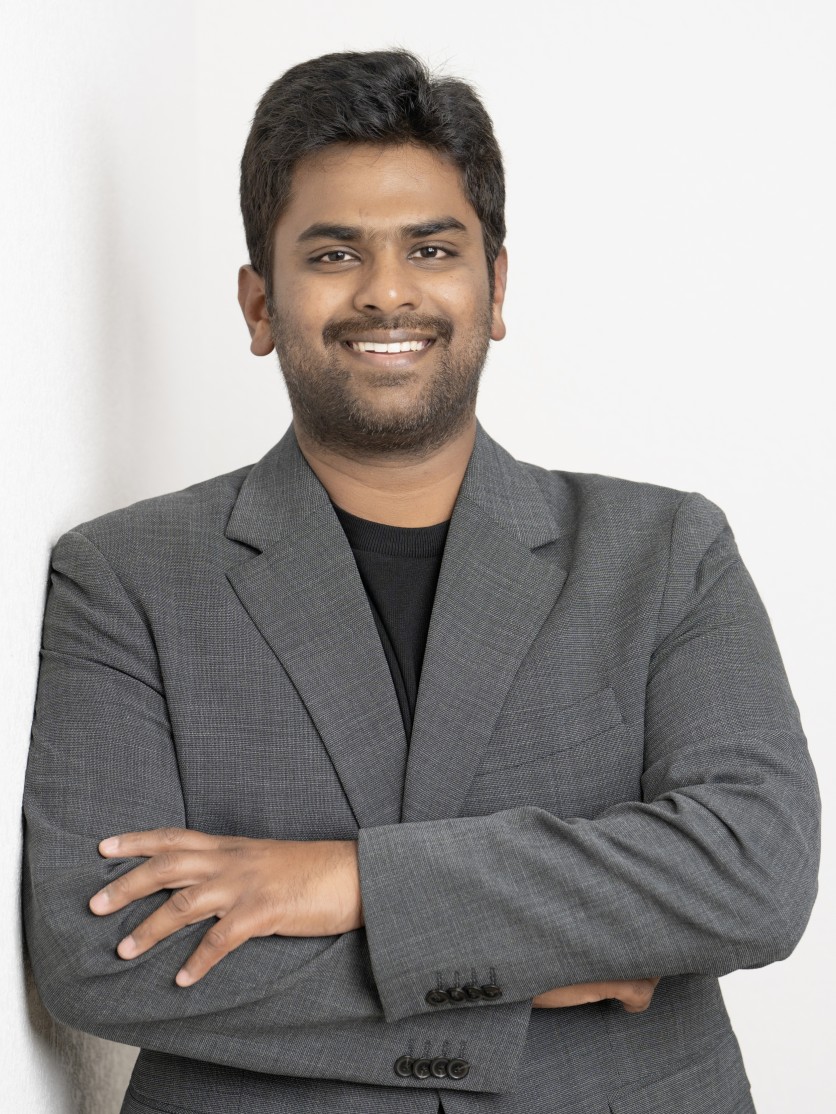
As Artificial Intelligence (AI) becomes an indispensable tool in enterprise financial operations, businesses are swiftly adopting automated solutions for processing invoices, detecting fraud, and managing complex cost allocations. However, despite remarkable advancements in efficiency, AI's pervasive integration into financial workflows has brought about one critical concern: a lack of transparency in decision-making processes. Explainable Artificial Intelligence (XAI) is rapidly emerging as a crucial advancement, poised to tackle this pressing issue by providing clarity and justification for decisions made by AI algorithms.
The Transparency Problem: AI's Black Box
Traditional AI models—particularly those driven by deep learning—often function as "black boxes," making critical financial decisions without providing meaningful insight into their internal decision-making processes. In regulated industries such as finance, this lack of transparency poses severe risks, complicating audit trails, regulatory compliance, and overall financial governance.
Enterprises that rely heavily on AI for critical decisions, such as approving high-value invoices or identifying fraudulent transactions, increasingly require explainability to justify automated decisions to auditors, regulatory bodies, and stakeholders. Without this clarity, enterprises risk costly compliance violations, regulatory fines, and reputational harm.
What Is Explainable AI (XAI)?
Explainable AI, commonly known as XAI, addresses these concerns by embedding transparency directly into the decision-making process of AI models. Unlike traditional opaque systems, XAI clearly communicates the rationale behind each decision, enabling finance teams and auditors to understand exactly how conclusions were reached.
This transparency is particularly critical for financial automation, where decisions can have significant fiscal consequences. Through XAI, enterprises can confidently adopt AI-driven systems, assured that they comply with regulatory demands and standards of accountability.
Applications of XAI in Financial Document Processing
XAI is increasingly integrated into financial document processing workflows, profoundly enhancing transparency in critical areas, such as:
1. Invoice Approvals
In invoice processing, AI algorithms are trained to approve or flag transactions based on complex patterns in historical data. With XAI, these models provide detailed explanations for invoice approval or rejection decisions. For example, if an invoice is flagged due to an unusually high amount of atypical vendor behavior, the AI will explicitly present these factors, ensuring auditors and managers clearly understand and validate each automated decision.
2. Fraud Detection
Detecting financial fraud has always been challenging due to sophisticated schemes and patterns of behavior. AI has vastly improved detection capabilities, but without transparency, these models can raise suspicion or confusion. XAI resolves this by providing explicit reasoning behind each fraud alert—such as highlighting specific anomalies or transaction characteristics—enabling finance teams to rapidly, investigate, and address fraudulent activities rapidly with clarity and confidence.
3. Cost Allocation Decisions
Enterprise cost allocation often involves intricate decisions about how to distribute shared expenses across departments or business units. XAI clarifies automated allocation decisions by explicitly stating factors that influence distribution percentages, ensuring fairness, consistency, and ease of validation during audits.
Key Benefits: Regulatory Compliance, Trust, and Improved Governance
XAI adoption in enterprise financial automation delivers three critical benefits:
- Enhanced Regulatory Compliance: Regulators increasingly demand clear justifications for automated decisions. XAI solutions offer documented, audit-ready trails demonstrating exactly how financial decisions are made, significantly reducing regulatory risks.
- Building Trust and Accountability: Transparent AI decisions build greater trust among employees, executives, stakeholders, and external auditors. Enterprises adopting XAI can confidently rely on automation, knowing every decision can be thoroughly understood and justified.
- Improved Financial Governance: Clear decision-making processes greatly enhance financial governance, enabling more informed oversight, proactive management, and streamlined auditing procedures. This transparency translates into better-managed operations, more accurate financial forecasting, and enhanced internal controls.
Challenges and Considerations for Implementing XAI
While the promise of XAI is compelling, enterprises face practical challenges during implementation. Issues such as data quality, model complexity, and the technical expertise required to interpret explanations pose significant hurdles. Companies must invest strategically in training, infrastructure, and tools capable of effectively leveraging XAI capabilities.
Despite these challenges, industry experts agree that the long-term advantages far outweigh the initial hurdles, given the profound improvements in transparency and compliance.
Future Trends: Advancing Toward Explainability as a Norm
The future trajectory of XAI in financial automation is clear: explainability is swiftly transitioning from a beneficial add-on to an operational requirement. Analysts forecast that regulatory environments will soon mandate explainable decision-making for financial transactions, fundamentally reshaping industry standards.
Emerging innovations in XAI research—including advanced interpretable models, interactive explanations, and intuitive visualization tools—are set to further empower finance teams. Enterprises that proactively integrate XAI into their financial systems today will undoubtedly hold a strategic advantage, already meeting the forthcoming regulatory and operational demands of tomorrow.
Embracing Transparency in Financial Automation
Explainable AI represents a transformative milestone in enterprise financial automation. As financial processes continue to grow in complexity and volume, organizations must increasingly rely on AI-driven decision-making. However, this reliance demands transparency, compliance, and accountability—precisely the solutions provided by XAI.
Through strategic adoption of Explainable AI, enterprises not only ensure operational efficiency but also significantly strengthen governance frameworks, foster stakeholder trust, and proactively navigate regulatory environments. Indeed, the future of enterprise finance lies not merely in automation but in explainable, accountable automation—making XAI an indispensable component in the evolution of intelligent financial operations.
Ranadheer Reddy Charabuddi continues to lead innovations in AI-driven financial systems, promoting transparency, trust, and regulatory compliance through the pioneering implementation of Explainable AI solutions.
ⓒ 2025 TECHTIMES.com All rights reserved. Do not reproduce without permission.