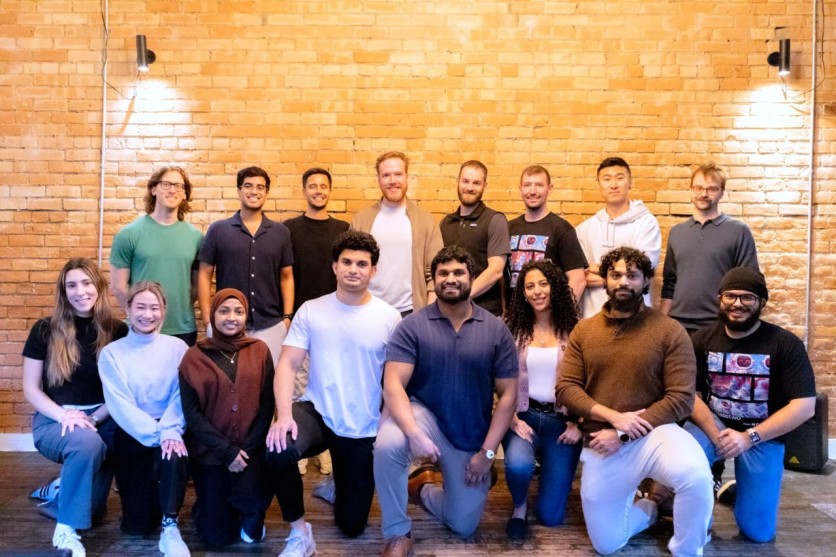
Fresh off an impressive $11.5M Series A funding round, Basetwo is poised to revolutionize manufacturing optimization with its innovative Physics AI platform. Combining chemical engineering principles with cutting-edge artificial intelligence, Basetwo offers a groundbreaking approach to solving complex industrial challenges, from scaling pharmaceutical production to reducing resource consumption.
In this exclusive interview, Basetwo's founder, Thouheed Abdul Gaffoor, shares how the recent funding will fuel expansion into key markets such as the US, Japan, Europe, and the Middle East while advancing their AutoPilot technology for autonomous manufacturing control. We also explore how their platform has already driven significant improvements in efficiency, sustainability, and product quality for global clients, as well as their vision for reshaping the future of manufacturing.
Congratulations on your recent $11.5M Series A funding! How will this investment help accelerate Basetwo's growth, particularly with the expansion into the US, Japan, Europe, and the Middle East?
This funding will allow us to invest in the necessary talent to build and deploy our autonomous control capabilities (AutoPilot), allowing us to deliver significantly more value for our existing and prospective customers and capture this value as revenue.
The funding will also enable us to expand our business development and customer support footprint in our core target market of chemical manufacturing—specifically pharmaceutical, consumer goods, and gas processing. The US, Japan, the EU, and the Middle East are the top global exporters of these product segments.
Basetwo's Physics AI combines chemical engineering principles with artificial intelligence. How does this approach differ from traditional machine learning models used in manufacturing optimization?
Traditional ML models learn from large amounts of accumulated historical data. We call them "black boxes" since they only learn input/output relationships but lack a physical understanding of the process they are learning about. In other words, they learn from correlation, not causal relationships. This is okay for problems such as classifying cats or dogs in images. Still, this would be highly problematic when simulating engineering problems such as chemical reactions in tanks, combustion in engines, or distillation in columns. This is because engineers need to be able to understand "how" these systems work to be able to control, troubleshoot, and optimize them.
As such, engineers have accumulated years of experience and knowledge about the underlying physics, chemistry, and thermodynamics of these processes that are not captured in the data. This knowledge is often captured in engineering handbooks and chemical/thermodynamics property tables.
At Basetwo, we believe it would be shortsighted to leave out the decades of physics/chemistry expertise that engineers have accumulated, as well as the vast amount of understanding about how engineering systems function. This is why we use Physics AI.
Basetwo's Physics AI technology combines the underlying physics of the process with the available historical data. We use engineering handbooks and chemical/thermodynamics property tables to build models that:
- Are rooted in a causal understanding of the physics/chemistry of the process
- Are explainable/interpretable by engineers and regulators (not black boxes)
- Can extrapolate to conditions where there is highly limited historical training data (i.e., when testing new compounds/drugs or new system designs)
This is extremely important because, in actual industrial processes, training data is often limited/narrow in terms of diversity since operators tend to operate the plant the same way historically or lack critical sensors.
Pharmaceutical and chemical manufacturers often face significant challenges when scaling production from lab to commercial levels. Can you explain how Basetwo's platform addresses these scaling issues and improves batch quality?
Scaling pharmaceutical and chemical manufacturing from lab to commercial production is complex and costly, often requiring significant manual/physical trial-and-error experimentation. This manual effort can take months or years, driving up costs and delaying time-to-market. A key technical challenge is that scaling up isn't a linear process—changes in geometry, scale, and chemical compound interactions can lead to unexpected shifts in chemical and thermodynamic behavior, making it difficult to ensure consistent performance at larger scales.
Basetwo's AI-powered platform addresses these scale-up challenges by providing:
- Predictive Scale-Up: Our platform enables manufacturers to virtually simulate the desired scale-up in advance, accounting for shifts in process dynamics due to changes in equipment size, material properties, and operating conditions.
- Physics AI for Process Understanding: Combining first-principles equipment knowledge, our database of over 2000+ chemicals, and machine learning algorithms, Basetwo helps manufacturers gain deeper insights into how process parameters and chemical compounds interact at different scales, ensuring a more reliable and efficient transition.
- Real-Time Insights and Adjustments: Our AI continuously monitors key process variables during scale-up, providing actionable insights and allowing teams to make data-driven adjustments, ensuring smooth and predictable production ramp-up.
By leveraging AI-driven modeling, Basetwo minimizes the need for extensive trial-and-error experimentation, significantly accelerating the scale-up process while reducing resource expenditure.
Your platform has demonstrated up to a 40% improvement in cycle times and raw material usage, alongside a 25% enhancement in product quality. Can you share a specific success story where Basetwo's technology significantly impacted a client's operations?
Using the Basetwo platform, one of our customers, a Top 5 Pharmaceutical manufacturer, deployed Basetwo to optimize cycle times, minimize material consumption, and streamline energy usage using AI-powered recommendations.
Basetwo provides real-time decision support for operators and engineers, providing dynamic insights into adjusting process parameters to maximize efficiency and product quality while respecting existing constraints and regulatory requirements set by authorities such as the FDA or EMA.
The pharmaceutical company successfully leveraged the Basetwo platform across its pilot and commercial plants. They reduced time to market by 50%, significantly decreased resource consumption, and improved energy efficiency, contributing to their ambitious net-zero targets. Overall, Basetwo facilitated a substantial 44% reduction in operational costs compared to previous batch runs, maintaining both product quality and operational efficiency.
With the funding to advance your AutoPilot technology for real-time autonomous manufacturing control, what are the key milestones you're focusing on for this innovation?
Our initial AutoPilot deployments will focus on rigorous testing in controlled environments, such as research and pilot facilities, to validate the system's control recommendations for process safety, cybersecurity, and product quality. System performance under highly dynamic feed/input conditions will be continuously monitored to ensure the AutoPilot is highly responsive to sudden changes in conditions.
Once the technology has been proven in these controlled settings, we will transition to semi-autonomous deployments in commercial environments, where human validation will still be required.
After successful testing in R&D and semi-autonomous commercial environments, we will proceed to cGMP deployments in full-scale commercial plants. During this phase, the AutoPilot controllers will be carefully constrained to ensure recommendations remain within a tightly controlled safety envelope.
You've partnered with major investors like AXA Venture Partners and Glasswing Ventures. How do these collaborations support Basetwo's mission, and what role does being part of the Hub71 community play in your ongoing development?
Our partnerships with AVP and Glasswing support our mission to empower manufacturers with AI-driven process optimization. These collaborations provide not only financial backing but also strategic guidance, industry expertise, and access to extensive networks that help accelerate our growth and innovation. Their support enables us to enhance our platform's capabilities and expand into new markets effectively.
The UAE serves as a critical gateway to the Middle East and broader Asian markets. Under initiatives such as Operation 300Bn and the UAE National AI 2031 Strategy, the UAE has a strong commitment to rapidly grow its manufacturing/industrial sector and invest in AI and digital transformation. Being part of the Hub71 ecosystem allows us to be a part of these key initiatives.
As Basetwo continues to grow, how do you see Physics AI reshaping the future of manufacturing, and what long-term goals do you have for the platform regarding sustainability and efficiency?
With our growth, our Physics AI technology will become more impactful for manufacturers, enabling more precise and efficient process optimization. Integrating deep scientific principles with AI allows us to simulate, predict, and enhance complex manufacturing processes in real time, driving greater productivity, enhancing quality, and reducing waste.
One of the most transformative aspects of Physics AI is its ability to preserve and formalize engineering knowledge across an organization. As industries face challenges such as an aging workforce and skill gaps, our technology ensures that critical process knowledge is retained and easily accessible. With a low-code, user-friendly interface, Basetwo empowers new engineers by equipping them with AI-driven insights, accelerating their learning curve, and upskilling teams to make data-driven decisions with confidence.
We envision a future where manufacturers operate lights-out facilities—highly autonomous, self-optimizing production environments powered by AI. Basetwo aims to serve as the operating system for manufacturers to deploy autonomous control across their operations, ensuring seamless integration of AI into everyday decision-making for improved efficiency and sustainability.
Regarding sustainability, our platform will help manufacturers optimize energy usage, reduce carbon emissions, and minimize material waste, all while improving product quality. The long-term goal for Basetwo is to establish our AI solutions as the industry standard for intelligent manufacturing, where every decision is informed by real-time data and advanced simulations, ensuring that operations are efficient and sustainable.
ⓒ 2025 TECHTIMES.com All rights reserved. Do not reproduce without permission.