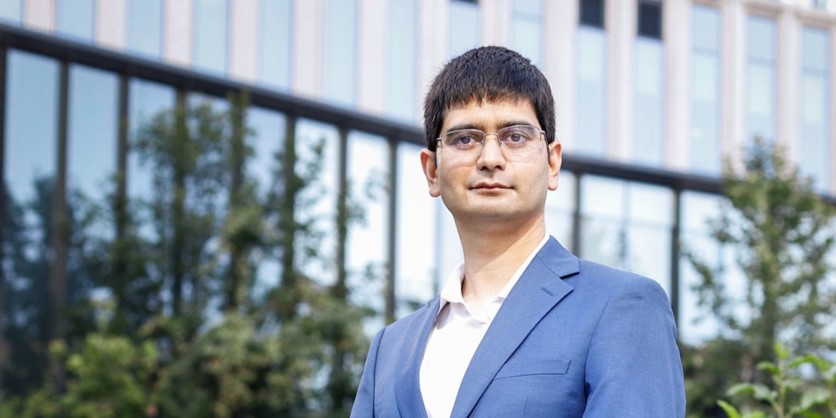
E-commerce giants are increasingly turning to machine learning (ML) techniques to ensure that their products reach the right audience at the right time, optimizing product discoverability and maximizing revenue. Aniket Sundriyal, a senior business intelligence engineer at Amazon's Woot.com, has become a leading figure in using ML to drive e-commerce success.
"It's not just about listing products anymore; it's about creating a personalized shopping journey for each customer," he explains. With over 14 years of experience in data analytics, Aniket Sundriyal's product visibility and customer lifecycle management projects increased Woot.com's engagement and profitability.
Beyond Woot, his strategies highlight the transformative potential of machine learning in tackling industry-wide challenges like discoverability and retention. By setting a standard for using data-driven insights to refine customer experiences, Sundriyal's work has inspired a shift in how e-commerce platforms approach growth, loyalty, and operational efficiency.
The Problem of Product Discoverability in E-Commerce
Product visibility is crucial in a fast-paced and fiercely competitive digital marketplace. Online platforms are challenged to make their extensive product inventories easily accessible to consumers. For Woot.com, which offers thousands of products across multiple categories like home, sports, and electronics, getting the right product in front of the right consumer can feel impossible. Without a strategic approach, customers may become overwhelmed by the sheer volume of items, leading to lost sales opportunities.
"The challenge is real," Aniket Sundriyal notes. "When you have such a vast inventory, there's always the risk of overwhelming the customer. They could get frustrated and leave without making a purchase." This problem is standard across the e-commerce sector, as businesses need help to balance variety with user experience.
This is where ML becomes essential. By predicting consumer behavior and ranking products based on their potential to sell, e-commerce platforms can create a more user-friendly experience while increasing revenue. Sundriyal's work is a prime example of this, as he has designed a highly effective curation algorithm that optimizes product listings by prioritizing the items most likely to drive sales.
Machine Learning: A Game Changer for E-Commerce
ML offers data-driven solutions to long-standing industry challenges. In Sundriyal's case, his curation algorithm for Woot.com leverages ML to predict each product's sales potential. The system considers hundreds of attributes to identify which products are likely to perform best.
"By applying machine learning, we're able to rank products based on their likelihood to sell, even before the event goes live," he says. This proactive approach helps prevent customer frustration by presenting high-potential products front and center, improving the overall shopping experience.
The benefits of this approach are manifold. Not only does it enhance product visibility, but it also provides real-time adjustments based on customer interactions during live sales events. The algorithm recalculates and updates the rankings as products are clicked on, added to carts, or purchased. This ensures that top-performing products remain highly visible, boosting engagement and driving sales throughout the event.
This system has proven highly effective for Woot.com, resulting in a notable increase in both customer engagement and sales. By using ML to optimize product discoverability, the platform has seen a significant boost in overall revenue. The algorithm also improved the visibility of top-selling products, ensuring they received priority during peak traffic periods.
How Machine Learning Enhances Product Discoverability
To fully understand the impact of ML on e-commerce, it's essential to delve deeper into how these algorithms function in practice. At Woot.com, Aniket Sundriyal's curation algorithm operates in two phases, each designed to optimize product visibility and sales.
The first phase begins before the product is even listed on the website. During this stage, the machine learning model analyzes historical data, such as previous sales performance, customer preferences, and seasonal influences. Using this data, the algorithm predicts which products will perform best and ranks them accordingly for the sales event. "Essentially, we're teaching the system to anticipate what the customer will want," he explains.
The second phase takes place during the sales event itself. As customers interact with the site and make purchases, the algorithm updates the product rankings in real time, adjusting based on actual sales performance.
This dynamic ranking system ensures that top-selling products remain prioritized while those not performing as well are gradually deprioritized. Woot.com can keep customers engaged and drive consistent sales throughout the event by continuously optimizing product placement based on real-time data. What sets this ranking system apart is its potential to reshape the e-commerce industry through its scalability and adaptability. By addressing Woot's specific challenges, it established a versatile framework that can be applied across other e-commerce platforms facing similar constraints.
The algorithm's innovative focus on ranking optimization, rather than relying on traditional personalization methods, provides a powerful solution for businesses seeking to enhance product discoverability in dynamic, high-volume environments. This approach has influenced the industry by demonstrating how advanced analytics can be leveraged to drive meaningful customer engagement and operational efficiency, setting a new standard for data-driven decision-making in e-commerce.
Beyond Sales: Improving Customer Retention and Experience
While ML's impact on product discoverability is clear, its benefits extend beyond sales figures. Sundriyal's work also demonstrates the potential of machine learning to improve customer retention and enhance overall satisfaction. One of his most notable achievements was developing a customer lifecycle management strategy. This strategy uses machine learning to predict high-risk customer segments and identify customer's product preferences.
By identifying high-risk segments for churn and their product preference, Woot.com was able to design personalized marketing campaigns targeted at these groups, encouraging repeat purchases and boosting customer loyalty. The results speak for themselves. As a direct result of these targeted efforts, Woot.com saw a considerable improvement in both customer retention and revenue.
Additionally, Sundriyal's ML models have enhanced Woot's ability to understand the voice of its customers. By applying Natural Language Processing to analyze customer feedback, he identified recurring themes in customer responses, allowing the company to make data-backed improvements to its customer service.
These strategies offer valuable insights not only for Woot but for the broader e-commerce landscape as well. Other companies and platforms can leverage similar ML-driven models to parse through vast amounts of data to understand customer preferences, identifying pain points and satisfaction drivers. By doing so, they can prioritize targeted improvements in customer experience, refine their service strategies, and increase loyalty—ultimately building stronger relationships with their customer base and gaining a competitive edge in the marketplace.
The Future of Machine Learning in E-Commerce
By 2030, experts predict that AI-driven personalization will become the standard in e-commerce, offering customers tailored shopping experiences anticipating their needs. Sundriyal believes ML will soon integrate with emerging technologies like augmented reality and voice search to create a truly immersive shopping experience. "Imagine walking through a virtual store where every product you see has been curated just for you," he says.
In this fast-paced environment, e-commerce businesses need every advantage to stay ahead of the competition. ML offers that advantage, providing platforms with the tools to optimize product discoverability, improve customer retention, and drive significant growth.
Through his innovative use of ML, Aniket Sundriyal is helping to influence the future of online retail, proving that with the right tools, businesses can thrive in even the most competitive environments.