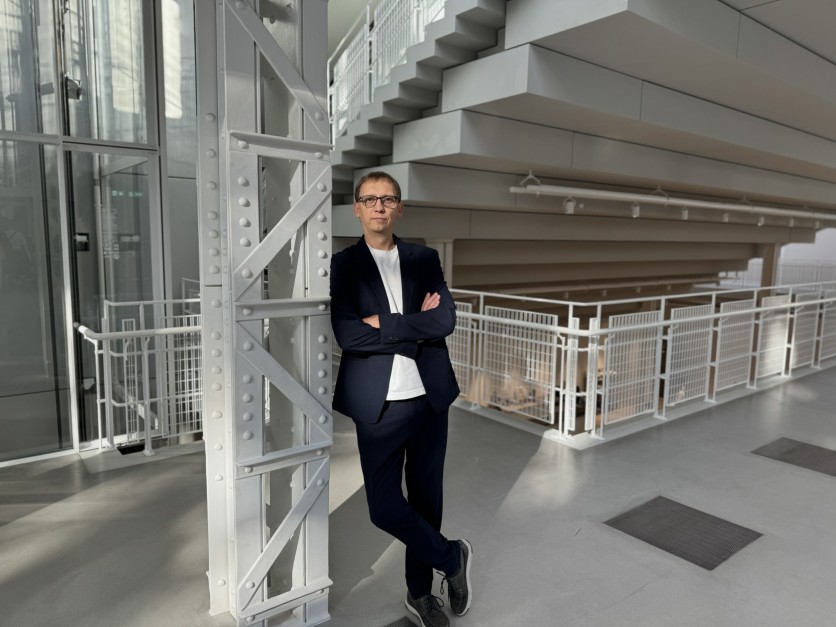
Data analytics is becoming the foundation for making business decisions, creating innovative products, and improving the quality of services. According to a report by Fortune Business Insights, the global data analytics market will reach $745.15 billion by 2030, growing at a compound annual growth rate of 13.5% during the forecast period.
The analysis and interpretation of large volumes of data are made possible by AI and machine learning-based solutions. This field is advancing rapidly, driven by the efforts of experts like Roman Styatyugin, director of the VK Predict Analytics Services Center. In this interview, he discussed the current development of technological solutions for data analytics and ML, the reasons behind their creation, and the innovations we can expect in the future.
Roman, you have 25 years of experience in product management within the IT sector. 9 years ago, you built a division from the ground up to develop IT products for corporate clients in the field of data analysis and machine learning. What do you think enabled you to successfully take on such a challenging task?
By that time, I had a great deal of experience in creating and developing IT products. For about 12 years, I worked at Diasoft, one of the largest developers and suppliers of IT solutions for the financial sector in the CIS. I started in a role that today would likely be called a "product manager," where I interacted with clients, analyzed their needs, managed priorities of development, acted as an analyst, and tested the product. In the next stage, I was entrusted with developing a whole portfolio of products for retail banking and managing a team of 300 people.
Later, I was appointed Director of the Business Development Department, where I shifted focus to strategy development, marketing, sales, and building relationships with key partners. During my time in this position, the company was ranked first and second in terms of core-banking solution sales to new clients, according to the IBS publishing rating.
In the early 2010s, my partners and I recognized the potential of a data-driven approach, particularly in big data analytics and machine learning, which were starting to gain traction at that time. We decided to create a startup focused on developing a data-driven product in the field of digital marketing and customer communications. Our solution was a data management platform that allowed us to accumulate information from various touchpoints between a company and its current and potential customers. For example, it could collect data from targeted marketing campaigns, product inquiries, website engagement, customer feedback, and call center interactions. Using machine learning techniques, the platform was able to analyze this data, extract valuable insights, and formulate recommendations.
At the same time, we were developing our Partner Solutions business. We partnered with two major companies, IBM and Teradata, and began implementing their software products in Russia. Through ongoing communication with the market and key vendors, we were able to test the vision and positioning of our own product. This approach paid off, and in the first year, we were able to deliver several successful projects.
By the time I finished working at the startup, I had a solid understanding of how successful data products are created, along with extensive experience in applying IT solutions in the banking sector.
When I moved to my current position, I was drawn to the opportunity to leverage my expertise in understanding the needs of large corporate clients in data analysis and machine learning. I saw the potential to align those needs with our company's technological capabilities and quickly drive new initiatives to commercial success. Within five months, we secured our first contracts and began generating revenue.
Which industries are most interested in smart data analytics solutions today? Which of these industries do you find most challenging for ML service developers to work with?
The most mature industries in terms of smart data analytics are banking, telecom, retail, and e-com. We have worked with all these industries. But perhaps the most rewarding experience has been in the financial sector. The ML services developed by my team are now used by a large number of banks in Russia.
Companies in the industries I mentioned have been working with data for quite a long time, so they have a clear understanding of what they need. They choose their technology partners carefully, ask the right questions, and set ambitious goals. Collaborating with them can be a significant challenge, but it's also where we see the most rewarding projects.
Firstly, experienced companies know which metric needs to be optimized and can clearly define the objectives, which is crucial for machine learning projects. Secondly, they can quickly integrate the development into their infrastructure, test it, and provide feedback. Thirdly, they have a good understanding of the economic impact they expect and the methods for measuring it.
Other industries are now actively investing in analytical products based on machine learning, including property developers, energy companies, manufacturers, and public authorities. With the help of ML services, they can solve a wide range of tasks—from common tasks like predicting customer behavior or selecting favorable business locations for business to more industry-specific tasks.
Your team recently won the Artificial Intelligence Alliance Award for a project with a client in the energy sector. What made this project unique?
Together with specialists from a federal energy company, we developed an ML service aimed at reducing power losses. The project was recognized by the jury of the National AI Russia Awards in the "Revenue Growth" category.
Our client, like other energy companies, faces the challenge of non-technical power losses. Typically, they are monitored by a team of analysts who rely on their experience to create a set of rules for identifying such situations. However, this approach is labor-intensive and doesn't always allow for analysis across the company's vast geography. Additionally, the number of parameters taken into account in the analysis is often limited.
By developing a mathematical model, we not only improved the quality of the forecast but also significantly increased the speed of data processing and reduced the labor intensity of calculations. In fact, this was one of the first data analytics projects using machine learning to solve such problems in the energy sector. After its successful implementation, we were able to scale our solution to other companies.
You mentioned property developers are investing in data analytics solutions. Your project in this industry was named AI Project of the Year by CNews. What do you think led to this recognition?
We won the CNews AWARDS 2022 award for the best AI project of the year in the property market. The idea originated during a brainstorming session with one of our clients—we realized that high-quality forecasting in the early stages can significantly affect the success of a new residential complex project. I initiated the creation of an MVP, and having confirmed the hypothesis, we began scaling the product.
We applied innovative approaches that had not been used before for similar tasks. For instance, we collected a unique dataset for model training, combining historical apartment sales data, geodata on infrastructure facilities, open statistics, and anonymous data on potential buyer profiles. We also focused on the user interface to ensure it was intuitive and user-friendly for the developer's business analysts.
As a result, we became the first to develop and offer Russian companies a service that solves a wide range of problems through the analysis of industry data. The service has already been successfully implemented by our client, allowing them to determine the optimal housing estate class and pricing based on sales rates, and to predict the apartment configurations in demand at a given time and location. Similar solutions from other players only emerged two or three years after we launched our service.
Data analytics solutions can greatly enhance business efficiency. How do you see the potential of using ML services to improve people's quality of life?
Our team has been with Russian regions for about two years, gaining a deep understanding of the challenges they face. Today, I can confidently say that analytical services are capable of providing a significant social effect.
One area where data-driven solutions can be applied is in landscaping. Recently, we launched a pilot project with the Ministry of Improvement of one of Russia's major regions to assess how efforts to improve public spaces and parks have influenced attendance and other key metrics. By leveraging geoanalytics services, we can identify the most popular attractions, understand the demographics and needs of the population, and optimize development strategies for these areas. For example, geoanalytics can predict that a particular park may become a popular spot for family recreation, signaling the need to upgrade playgrounds and focus on safety improvements in that location.
The tourism sector holds great potential for the use of ML services. Many regions are now working on increasing their tourist appeal, and to invest effectively in project development and positioning, it's crucial to understand the profile of visitors—who they are, what interests them, and which locations attract them and why. In addition, regional authorities can analyze how tourists prefer to reach specific attractions, enabling them to prioritize road repairs along key routes, organize public transportation options, and plan convenient stops, cafes, and souvenir shops.
Of course, ML services also make it possible to solve digital inequality issues. By analyzing communication quality in different locations, it's possible to identify areas where network infrastructure needs to be prioritized for development. These services not only help improve communication on a day-to-day basis but also ensure public safety during emergencies. For example, in one region, during floods, ML services could monitor and predict communication disruptions due to flooding of network equipment. This would allow authorities to take proactive measures, ensuring continuous informing flow and enabling feedback from residents in flooded areas.
As the head of a large team of developers, data scientists, analysts, and managers, how involved are you in projects?
When we enter a new industry, begin working with a new customer, or do something we've never done before, I always get personally involved in the project. This was especially true for the development of solutions for the energy sector and property developers, as we discussed earlier.
The responsibility of any good manager is to build and motivate the team, helping employees overcome obstacles. Just imagine how difficult it can be for specialists with several years of experience in, say, advertising technologies to switch to an entirely unfamiliar field like the energy sector. In some cases, my involvement in customer negotiations and supporting the team in building relations with partners becomes essential.
You can always complete a one-off project and move on, or you can create a service that will be in demand for years and build solutions for an entire industry. I am a strong believer in the second approach. As a manager, what excites me more is not just delivering a one-time service for profit but building a sustainable business that will generate revenue long-term. That's why I'm always personally involved in new projects. Once the project is on track, we set performance indicators and define the development strategy, after which most of the operational work is handed over to the product managers.
You led a team of around 100 employees. How did you build such a strong team, and how do you keep them together in a competitive job market?
When we started, I had to build the team from scratch. Initially, only a few people were working on the first projects to help develop the new direction. Over time, I expanded the team and established a system for training and professional growth, particularly for young, promising talent. We now hire interns, many of whom remain on our staff. Creating a strong and engaged team is an ongoing process. We are constantly developing our employees, helping them acquire new skills and stay updated on the latest technologies.
To ensure our specialists deliver strong results, I've established a system with a clear division of roles. Line managers play a key role in team development, staying closely connected to their teams, gathering feedback, and making necessary adjustments. We also offer horizontal and vertical growth opportunities. If a developer wants to explore new technologies or take on different challenges, we move them to a new project to keep them engaged. Horizontally, we have product leaders who act as mini-CEOs for their products, overseeing market positioning and backlog prioritization. Delivery managers coordinate the work of the technical teams, while our project office handles non-product-related tasks, ensuring that projects meet deadlines and commitments.
I place a strong emphasis on eliminating any grey areas in our work. Everyone on the team understands what they are doing when they are doing it, and for whom—the work of the departments is synchronized, with employees involved in annual planning. Product roadmaps are broken down into sprints, and each specialist has a clear set of tasks with deadlines.
Another focus is fostering internal communication. Teams regularly share updates on the products they're working on, the results they've achieved, and new technologies. Specialists are encouraged to stay engaged in the process—it's important for them to feel connected to the outcomes and see that their work contributes to something valuable that benefits customers, the company, or society. This involvement helps keep the team motivated and supports staff retention.
Professionals at your level are often engaged as experts. What projects have you been involved in, and why do you allocate time for such activities?
Indeed, I am invited to participate in various competitions and conferences as an expert—I think it is important to support and develop talented specialists and share my experience in creating AI-based products and services with them.
Among the latest projects, I can mention my participation as an expert at the international hackathon of the project 'Digital Breakthrough. Season: Artificial Intelligence.' I also regularly participate in the expert review of projects involving the application of artificial intelligence technologies within the framework of the national Digital Economy programme (Data Economy Russia).
How do you see the market for artificial intelligence and machine learning solutions evolving? What are the three key trends you foresee?
First and foremost, it's the search for effective scenarios for applying generative AI to solve business problems. Today, large language models (LLMs) from various developers are actively used for code generation, enterprise search, text summarization, copywriting and image generation, and development of support chatbots. However, for more complex tasks, such as optimizing technological processes of industrial enterprises, the best practices of GenAI use are still being developed.
The second trend gaining attention is the democratization of machine learning. New products and no-code tools are emerging, allowing users without specialized education to work with these technologies. Whereas data analysis using ML was once limited to data scientists, AutoML tools are now empowering business analysts to discover how these technologies can address company challenges.
The third trend is confidential computing, which enables the generation of necessary data sets and training of AI models without transmitting or disclosing sensitive information from various participants. This includes technologies like federated learning and the creation of secure crypto-enclaves. Once these solutions are fully productized in the market, businesses will receive additional developmental opportunities. We, too, are also actively moving forward with developing such innovative products.