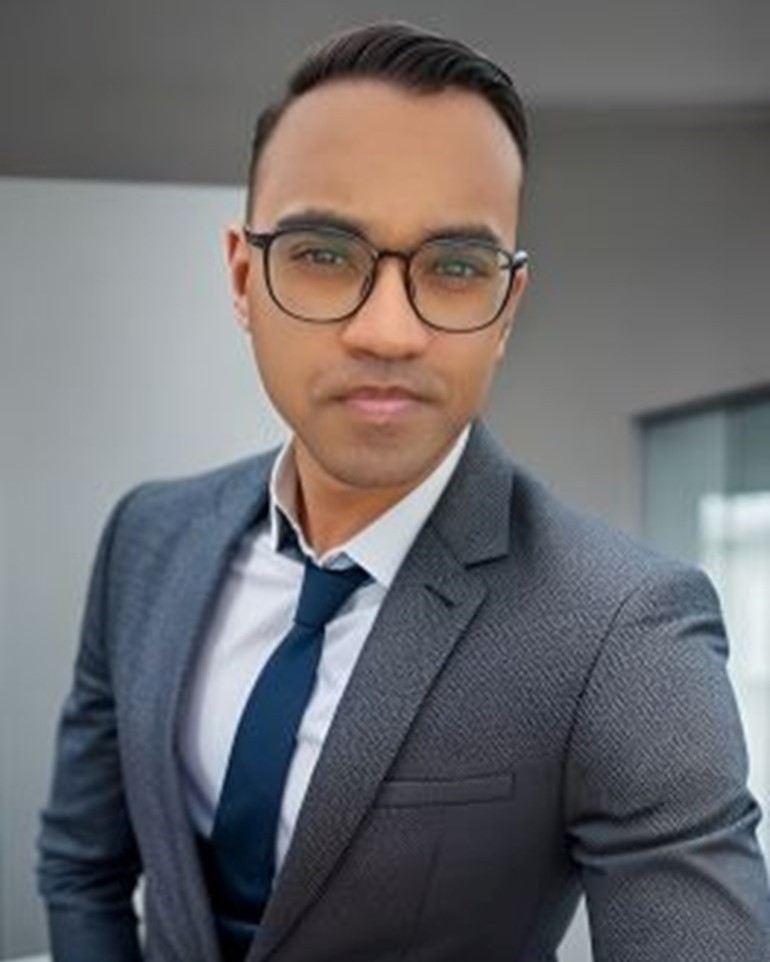
Maria Anurag Reddy Basani, a seasoned expert in data engineering and analytics, has made significant strides in the field over the past decade. With experience spanning industries such as insurance, finance, government, and technology, Mr. Basani currently serves as a Senior Data Engineer at Meta. His work focuses on data engineering, data warehousing, business intelligence, and big data, with a keen interest in developing scalable data solutions that provide actionable insights. His initiatives have optimized data processes, built robust data infrastructures, and implemented innovative solutions that enhance organizational success.
Mr. Basani's contributions have been recognized with awards like the Global Recognition Award, the Platinum Titan Award in Big Data, and the Claro Gold Award in Data Analytics. He is also a Senior IEEE member, Threws Fellow member, and a full member of Sigma Xi, reflecting his commitment to advancing data engineering and analytics.
Presentation at IEEE UV 2024 Conference
At the IEEE UV 2024 conference, Mr. Basani presented his research paper, "Optimizing ETL Pipelines with AI." This paper introduces a comprehensive framework for enhancing Extract, Transform, Load (ETL) pipelines using AI techniques such as reinforcement learning and incremental learning. The AI-driven ETL pipeline dynamically adjusts data extraction, transformation, and loading processes, resulting in significant improvements in data integration performance.
Overview of the Paper
The paper addresses a critical challenge in the era of big data: the efficient management, integration, and processing of vast amounts of information. Traditional ETL pipelines, foundational to data integration systems, often struggle with manual configurations, rigid workflows, and scalability constraints. Mr. Basani's research proposes an AI-driven framework to overcome these limitations, enabling more intelligent and adaptable data integration processes.
Key Contributions
- AI-Driven ETL Framework: The framework leverages AI techniques, including reinforcement learning and incremental learning, to optimize ETL pipelines. This approach dynamically adjusts data processes, leading to significant performance improvements.
- Experimental Validation: Experiments using a financial transactions dataset demonstrate the framework's effectiveness, achieving a 48% reduction in latency, a 78% increase in throughput, and enhanced data quality compared to traditional ETL systems.
- Machine Learning Integration: The AI-driven pipeline not only improves ETL processes but also enhances the accuracy of machine learning models trained on the processed data, with a 5–6% improvement in accuracy.
Methodology
The proposed framework is structured around several key components:
- Dynamic Data Extraction: AI models automate the detection and prediction of relevant data sources, reducing the need for manual intervention and ensuring data is gathered from the most pertinent sources.
- AI-Driven Transformation: During the transformation phase, AI models optimize transformation rules by learning from historical patterns. This improves data cleaning, normalization, and enrichment tasks, creating a self-correcting system with minimal human oversight.
- Intelligent Data Loading: The framework enhances the load phase by dynamically adjusting database structures and optimizing query performance in real time, ensuring efficient data storage and availability.
- Continuous Learning: The system incorporates incremental learning techniques, allowing it to adapt to new data patterns and requirements, maintaining agility and efficiency as data sources evolve.
Impact and Implications
Mr. Basani's research highlights the transformative potential of AI in ETL processes. By integrating AI-driven techniques, organizations can achieve scalable, efficient, and accurate data integration solutions. This approach not only improves the performance of ETL pipelines but also enhances the quality of insights derived from data, enabling more informed decision-making.
The AI-driven ETL framework offers several advantages over traditional systems. It reduces the need for manual configurations, allowing for more flexible and responsive data integration. The use of AI techniques such as reinforcement learning and incremental learning ensures that the system can adapt to changing data environments, maintaining high performance and data quality.
The paper concludes with a discussion on the future of AI in data integration, emphasizing the need for continued research and development to refine further and expand the capabilities of AI-driven ETL systems. Mr. Basani's work sets a new standard for data integration, paving the way for more intelligent and responsive data management solutions in the big data landscape.
ⓒ 2025 TECHTIMES.com All rights reserved. Do not reproduce without permission.