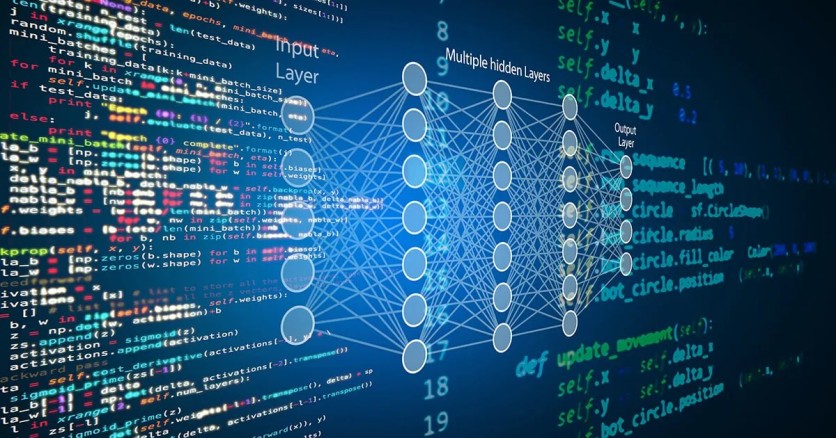
Database design and management are essential pillars of success in the financial sector, where efficient data handling and adherence to regulatory standards are critical. The global market for database management systems is projected to expand from approximately $63 billion in 2021 to over $100 billion by 2026. This growth is primarily driven by the financial services industry, as organizations strive to leverage data for insights and improve operational efficiency. Effective database management allows financial institutions to process vast amounts of information, enhance customer experiences, and maintain compliance, ultimately supporting strategic decision-making and business growth.
In this fast-evolving landscape, integrating advanced technologies and innovative methodologies is vital for addressing fraud—a persistent challenge for financial institutions worldwide. One researcher making significant strides in this area is Navya K. Alapati, whose work on Graph-based Semi-Supervised Learning for fraud detection presents promising solutions for identifying fraudulent activities within complex financial systems.
The Challenge of Fraud Detection
Fraud in the financial sector poses substantial risks, costing organizations billions of dollars annually. Traditional fraud detection methods often struggle to keep pace with the sophisticated tactics fraudsters employ. According to the Association of Certified Fraud Examiners (ACFE), organizations lose an estimated 5% of their revenues to fraud each year. As financial transactions become increasingly digital, the volume and complexity of data have surged, complicating the detection of fraudulent patterns. This has created a pressing need for innovative approaches that can enhance detection capabilities while reducing false positives.
A Research Breakthrough:
Navya's recent research has introduced an innovative approach to enhancing fraud detection mechanisms in finance through Graph-based Semi-Supervised Learning. This methodology merges the strengths of graph theory and machine learning, allowing for a more nuanced analysis of relationships and patterns in financial data that traditional methods might miss. By utilizing graph structures, this approach models complex interactions between entities, including customers, transactions, and accounts, thereby providing deeper insights into potential fraudulent activities.
A significant advancement of this research is its semi-supervised learning aspect, which enables the model to learn from both labelled and unlabelled data. In the finance sector, where labelled data—transactions identified as fraudulent or legitimate—can be scarce, this technique allows organizations to leverage the vast amounts of unlabelled data available. This enhances the model's accuracy and effectiveness in identifying anomalies.
The implications for financial institutions are substantial. By adopting these techniques, they can significantly improve their ability to detect suspicious activities, reducing false positives that can erode customer trust. Moreover, the capacity for real-time data processing allows for rapid responses to potential fraud, minimizing losses. As transaction volumes increase, this scalable solution ensures organizations maintain high-quality detection without compromising performance.
Besides this Another important contribution is her research on integrating genetic programming and clustering for intrusion detection in cybersecurity, which enhances the detection of potential threats and emphasizes the role of machine learning in security strategies.
A Step Toward Innovation
The transformative impact of Navya K. Alapati's work beyond immediate fraud detection. By advancing semi-supervised learning and graph-based analysis, this work enhances the fight against fraud while paving the way for future advancements in data analytics and machine learning within finance. In today's data-driven landscape, leveraging such innovative technologies is essential. Financial institutions that adopt these methodologies are better equipped to navigate the complexities of modern fraud detection, fostering greater trust and confidence among their clients.
The Broader Impact on Financial Services
As the financial sector continues to evolve, the significance of innovative research cannot be overstated. Alapati's contributions exemplify how academic inquiry can lead to practical solutions that address real-world challenges. Financial institutions that embrace these advancements not only improve their fraud detection capabilities but also enhance their overall data management practices.
The integration of cutting-edge research like Alapati's will likely influence regulatory frameworks as well, prompting regulators to adapt to new technologies and methodologies. As fraudsters become more sophisticated, regulatory bodies must also evolve their strategies to ensure that financial institutions can effectively combat fraud while protecting consumer interests.
The Path Forward
Navya's work on Graph-based Semi-Supervised Learning for fraud detection marks a noteworthy development in the financial sector's efforts to combat fraud. By improving the accuracy and efficiency of detection systems, this work supports safer financial transactions. As the industry increasingly adopts innovative solutions, the contributions from this research will be crucial in advancing fraud detection and data management. With ongoing technological advancements, the financial sector is better positioned to mitigate fraud-related risks effectively.