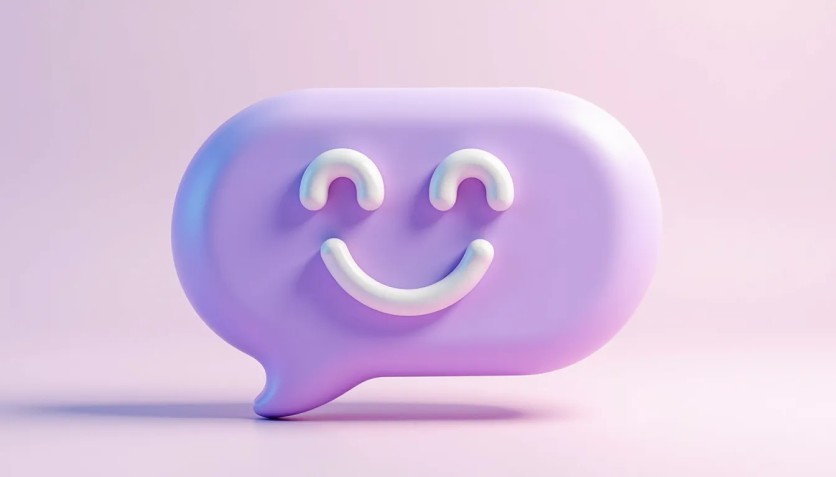
The customer service industry has benefited greatly from Artificial Intelligence (AI) integration, which automates customer service, enhances efficiency, and personalizes customer interactions.
AI helps answer questions and resolve issues quickly but can run into problems with fundamental technical limitations. For example, while a chatbot can respond quickly to customer inquiries and provide immediate assistance while reducing wait times, it may struggle to understand nuanced or complex queries, which can lead to incorrect responses.
Challenges with Traditional AI Solutions
Most AI assistants use "RAG" (Retrieval-Augmented Generation) to answer questions by retrieving the most relevant information from a knowledge base and injecting it into the context window of the Large Language Model (LLM). When the AI searches for the most relevant information for a given situation, instead of referencing the entire knowledge base, which would be very costly and slow, the general practice is to take the user's question and semantically search the knowledge base for the most similar information related to that question. However, the processes that platforms are powering this whole process, called their "RAG pipeline," are often not tailored to a business's use cases, which increases the risk of errors in information retrieval and leads to bad customer experiences.
Some examples of parameters that make up a RAG pipeline are the number of similar 'documents' to take from the knowledge base, the vectorization model, what chunking method should be used, etc. Inaccurate responses from a chatbot can be attributed to a lack of fine-tuning the parameters in this RAG pipeline to a specific use case.
Furthermore, AI platforms generally don't give transparency on why the bot answered the way it did. The raw request sent to the LLM is not available to businesses that are building AI assistants, which makes it even harder to debug inaccurate answers and create great customer experiences.
Haseab, founder and CEO of automatic.chat, recognized these shortcomings in customer service AI, and began working on a solution.
Haseab and 'Automatic Chat'
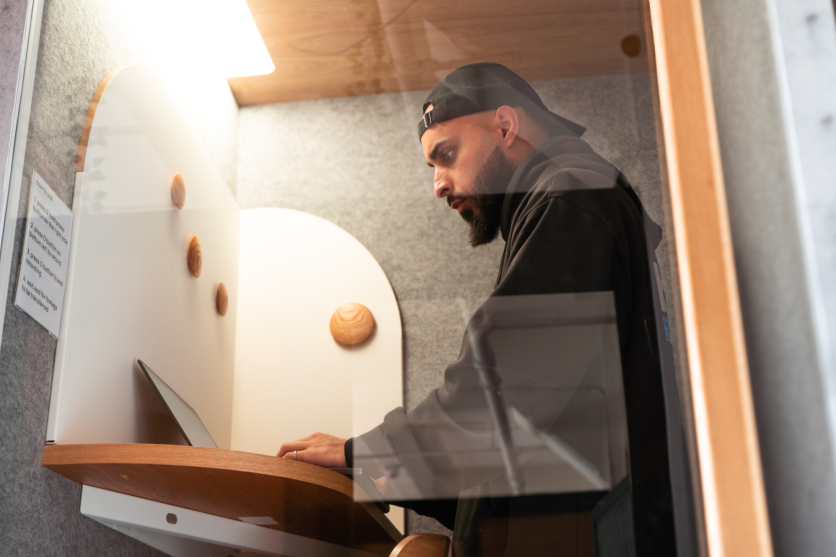
Haseab has a rich and experienced history working with AI. Before working on automatic.chat, he co-founded micropay.ai (2022–2023) and llm.report (2023–2024), both of which emphasized AI accessibility and found success in niche markets.
For instance, llm.report, one of the first AI observability tools of its kind, attracted significant interest from top venture capital firms and was adopted by numerous unicorn startups for cost monitoring. Haseab applied for the Vercel AI Accelerator and was one of just 40 companies to be accepted out of 1,400 applicants. Of those 40, llm.report won "best individual project" and was awarded $120,000 in prize credits.
Using the insights on LLM observability he gleaned from llm.report, Haseab is bringing his vision of developing observable and debuggable AI to life through automatic.chat. He has partnered with several major businesses, one of which was Heroic Signatures, a subsidiary of the large Chinese conglomerate Tencent. Together, automatic.chat and Heroic Signatures successfully launched a Text to Adventure AI chat game under the Conan Exiles Universe that garnered over 50,000 AI messages in just one week.
As the platform grows, Haseab has continued to focus on allowing observable and customizable RAG pipelines for businesses with no IT departments to build robust AI assistants. There are thousands of companies already building on the automatic.chat platform that Haseab developed.
How Haseab & Automatic Chat Are Changing the AI Support Game
The automatic.chat platform aims to provide non-technical businesses with the resources they need to create robust AI assistants by making the technology more observable and customizable.
Observability and debugging are key innovations of automatic.chat, providing businesses with a complete understanding of their AI assistant and where it can be improved. Businesses can debug a given response automatically by observing the raw request sent to the LLM and the LLM's responses to specific prompts. This allows the company to improve the AI's responses.
Furthermore, automatic.chat allows businesses to custom-tune their RAG pipeline parameters to their own use case and gives them a frontend user interface to see the vector database and edit the documents inside of it. This gives them power that previously only a developer would have.
Industries That Debuggable AI Helps
Haseab serves three notable industries where accurate answers could be crucial: wealth management, law firms, and non-profit communities.
With wealth management firms, automatic.chat provides internal support for a firm's clients,
helping them navigate their finances and answer queries like how to get in touch with their advisor, what financial instruments might exist, or any questions they have about their account. Wealth management businesses need high-accuracy AI support in order to provide a great service while not soliciting any financial advice in the process.
Law firms, on the other hand, are using automatic.chat on their website as an intake bot, where the chatbot greets the user on their website, provides support, and collects information about prospective clients without soliciting legal advice. Users explain their personal situation and ask what they should do, and the AI needs to carefully provide an answer to the user without responding with an opinion. If the AI were to give legal advice accidentally, then the company could be held liable.
Non-profit communities are another area where the use of a debuggable AI Assistant Platform like automatic.chat is crucial. These volunteer-based communities suffer from a range of issues that AI assistants can solve, such as inefficient employee onboarding, lack of member support, limited governance visibility issues, inefficient member recruiting, limited member engagement, and limited knowledge of institutional memory. Automatic.chat has notably helped non-profit housing communities tackle these challenges.
An AI Future in Customer Service
As the AI landscape continues to evolve, debuggable AI solutions like automatic.chat are emerging as essential tools for businesses across multiple industries. The platform tackles one of the biggest challenges facing AI today: ensuring accuracy, transparency, and customization in AI-driven interactions. By empowering businesses, even those with no technical expertise, to create highly customizable and accurate AI assistants, automatic.chat is poised to transform how companies engage with customers, clients, and internal teams.
Looking ahead, automatic.chat and similar debuggable AI technologies represent the future of customer service and operational support. As the demand for more personalized, accurate, and reliable AI interactions grows, platforms like automatic.chat are leading the charge in setting new industry standards.
Haseab's automatic.chat is not just a tool for automation; it is a dynamic solution that empowers businesses to shape their AI in real time, adapting to the changing needs of both their industry and their customers.