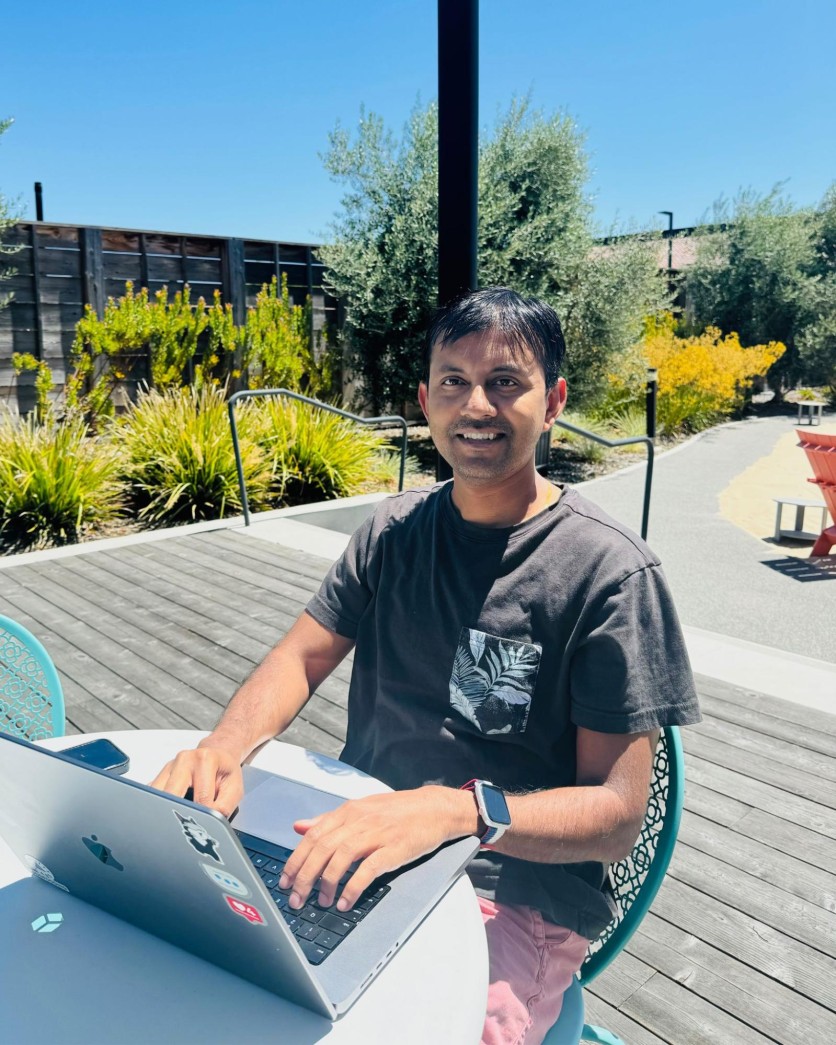
These days, it's all too easy for anyone to manipulate digital content and share it with millions of unsuspecting users. Whether it's used to spread misinformation or carry out scams, digital fraud has become a widespread issue, leaving people increasingly distrustful of what they see online.
Software engineer Vineet Dhanawat is addressing this problem with a fraud detection system that can detect fake images with higher accuracy than historically possible. His work is making it possible to improve online security and establish a safer, more reliable digital environment.
Learn more about Dhanawat and how he's mitigating the impacts of digital fraud.
Building a Career in Industry-Leading Tech Companies
After getting his bachelor's in computer science at the Birla Institute of Technology and Science and his master's from the University of Texas at Dallas, Vineet Dhanawat soon found work as a software developer for widely known companies such as Oracle and IBM.
Early in his career, Dhanawat became interested in machine learning—advanced AI algorithms that learn from large datasets in order to perform tasks, solve complex problems, and offer statistical insights. The challenge, he found, was finding a good use case for the tech.
Dhanawat's Work with Fraud Detection
The answer came to Dhanawat in 2023 after he experienced a series of fraud alerts while traveling abroad. "My credit card was constantly getting flagged for fraudulent transactions," he explains. "And while my bank eventually managed to help me out, I kept thinking how there was so much more they could do to address these situations."
After this, Dhanawat began to explore the mechanics of digital fraud in hopes of creating a way to fight it. Specifically, he focused on finding ways to detect image manipulation, a particularly prevalent and powerful tactic. While it's not terribly difficult to identify an image that's been spliced together from multiple sources, Dhanawat realized that it's not quite as simple to detect what's known as a copy-move forgery: the copying and pasting of data within the same image. Since the fraudulent area comes from the same graphic and is therefore consistent with the rest of the image, many traditional fraud detection models struggle to pick up on it—let alone the human eye to notice.
Dhanawat's solution is based on graph convolutional networks (GCNs), an advanced AI technique that specializes in analyzing complex structures. Instead of dividing an image into pixels or chunks like most systems do, Dhanawat's model converts it into a graph of interconnected data points for better processing and analysis. This allows the model to scrutinize better the image's details and visual patterns (like its tone, lighting, and shapes) and automatically detect any areas that break from these patterns—thereby identifying any digital manipulation.
This technology is more effective than traditional detection methods, and Dhanawat notes that it has significant potential across multiple industries. For example, financial institutions can use it to spot-check fraud, courts can identify the tampering of evidence, and social media sites can detect and flag falsified images before they go viral.
Promoting Safe AI Solutions
Shortly after finishing his research, Dhanawat detailed his findings in the academic paper "Copy-Move Forgery Detection Technique Using Graph Convolutional Networks Feature Extraction," which was recently published in the Institute of Electrical and Electronics Engineers' scientific journal. He's continuing his studies into machine learning algorithms, with similar articles to be published later this year.
Currently, Dhanawat is working at FAANG, where he works to enhance the integrity of platforms that affect millions of users. "With AI becoming more accessible than ever," he says, "I want to make sure this technology is being used to provide safe, quality services that people can trust."
To learn more about Vineet Dhanawat's research and work with AI, visit his Google Scholar profile.