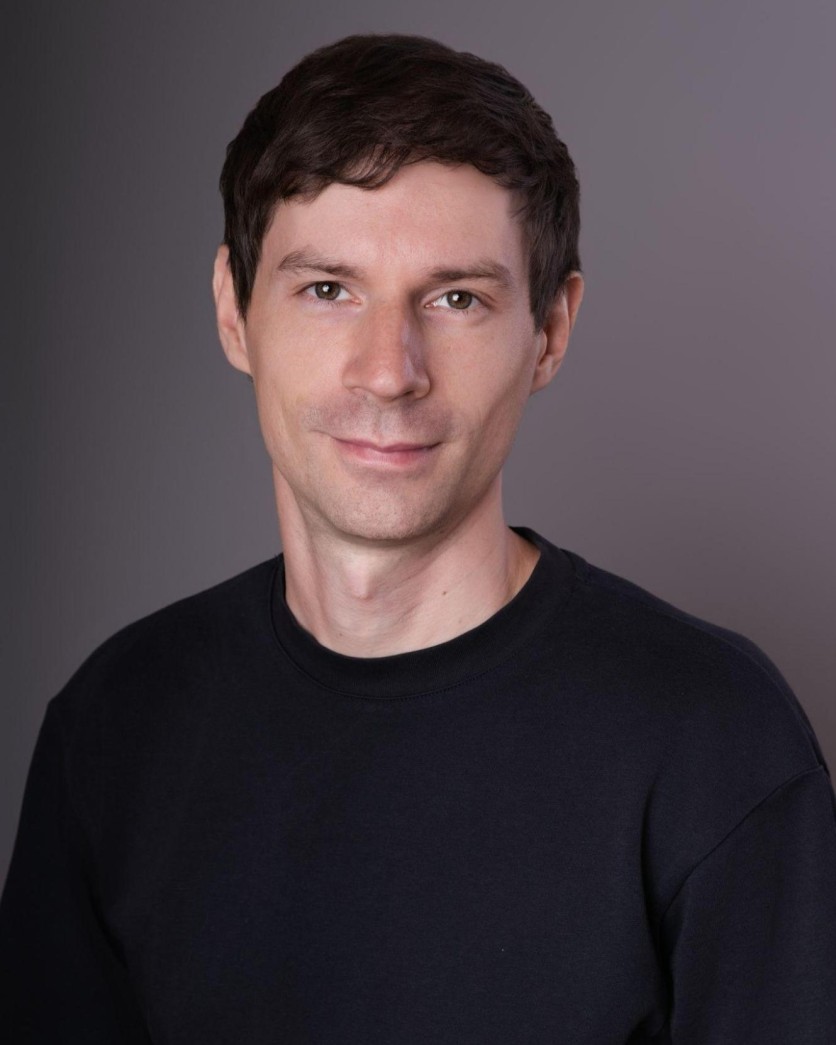
The market has faced a few major shocks in recent years, such as the 2008 financial crisis and the COVID-19 pandemic. These events, commonly referred to as "black swan" events, rocked global markets to their core and threw economies into turmoil. During the 2008 recession, for example, the U.S. government had to intervene to stabilize the market and implement new regulations that would mitigate the crisis and prevent similar situations. However, finance is always evolving, which means there's always the potential for another unprecedented black swan threat to shake things up.
Mathieu Tancrez, an expert in financial engineering and risk management, is tackling the unpredictable nature of black swan events with artificial intelligence. He created StressGen to create AI-driven scenarios based on past global crises, current economic/political context, and hypothetical future events to predict their impact on the economy. In doing so, it efficiently generates risk assessments without needing a large team of experts to manually sift through data, identify risks, and develop and calibrate scenarios. By automating these tasks, StressGen helps financial institutions stay ahead of the curve and better handle unexpected challenges in the future.
Learn more about Mathieu Tancrez's AI-driven approach to managing the risks of black swan events.
The Impact of Black Swan Events on Global Markets and the Challenges of Predicting Them
There are several good examples of black swan events that have had a significant impact on global markets.
One is the 2008 financial crisis, which was sparked by banks offering risky subprime mortgages to borrowers with poor credit. When borrowers defaulted, it caused a housing market crash. This sharp decline in property values caused massive losses for financial institutions, sparking bank failures and a financial meltdown that spiraled into a global recession.
Another example is the 1997 Asian financial crisis, which began when Thailand's currency (the baht) collapsed after the Thai government ran out of money to keep its value pegged to the U.S. dollar. This devaluation triggered a financial crisis that spread rapidly to other countries in the region, with stock markets crashing and banks and corporations failing under the weight of their debt. The International Monetary Fund (IMF) had to step in with emergency loans for the hardest-hit countries, and even though disaster started in Asia, the effects of the crisis were felt globally.
Similarly, the COVID-19 pandemic had far-reaching impacts on jobs, banks, and everyday people. With the global supply chain in chaos and lockdown-imposed business closures, the world's economy took a major hit, leading to widespread job losses, reduced consumer spending, and a slowdown in production. The result was a global recession that forced governments to step in with stimulus packages to try to stabilize their economies.
Understandably so, these crises were nearly impossible to predict.
That's not to say problematic variance is unpredictable—regular market fluctuations caused by economic cycles, inflation, or interest rate changes can be predicted because they happen frequently enough for us to understand their causes. Traditional risk metrics, like value at risk (VaR) and expected shortfall (ES), are commonly used to assess these risks, but most of the time, they rely primarily on historical data. As such, they work well when the current economic context is aligned with past scenarios, but they fall short when it comes to rare, extreme events like black swans.
Cognitive biases can also play a role. People often underestimate the likelihood of extreme events simply because they haven't experienced them and are prone to assume that things will continue as they always have—making it difficult to acknowledge the very real risks that pose a grave threat to the markets. Over time, people often forget the lessons learned from past crises (or they're lost to previous generations), and as the memory of previous events fades, complacency can quickly take root.
The Power of AI in Anticipating the Unexpected
Mathieu's solution to tackling these challenges is StressGen, an AI-powered tool built with OpenAI's LLM and Python.
Traditionally, financial institutions rely on teams of experts to create "what-if" scenarios—like a sudden market crash, geopolitical conflict, or massive corporate bankruptcies—to predict potential shocks. However, this manual process was slow, prone to human biases, and limited by the imagination of risk managers. But above all else, they were static and not updated with current economic contexts. For example, financial institutions may develop scenarios for upcoming U.S. elections, but they may not update the scenario every time a poll is published or after a candidate releases statements on policy.
But StressGen takes this a step further by automating the entire process and implementing real-time data in addition to historical data, allowing it to develop scenarios that are more comprehensive, imaginative, and efficient than traditional models. This way, risk managers can better prepare for and measure the impact of "market shocks" like interest rate spikes or significant stock price fluctuation.
StressGen offers a mix of qualitative and quantitative analysis, which shows expected changes in financial values like stock prices and interest rates and provides context on how different industries or markets could potentially react. Risk managers can then use these analyses to assess a variety of potential threats and adjust portfolios, improve strategies, or stress-test their existing financial models as needed. With faster scenario generation and fewer biases than manual methods, StressGen gives financial institutions a clearer view of their risks, helping them stay ahead of unforeseen events.
By comparing current market conditions with historical data, StressGen promises a more accurate and comprehensive financial analysis than traditional approaches.
In addition to what's already possible with StressGen, Mathieu is developing ways to compute the probability of each scenario, giving financial firms a clearer idea of what risks to prioritize by providing a clearer picture of potential losses. Higher probabilities may encourage a firm to take preemptive steps, such as adjusting their investments or increasing liquidity, while a scenario with an extremely low likelihood would allow the firm to reframe its focus on more probable risks.
StressGen is a powerful, real-world application for LLMs thanks to its ability to understand risk factors, fetch relevant data and news, mimic historical crises, and generate hypothetical scenarios—even black swan events.
Preparing for Financial Crises with AI
Mathieu's work on StressGen shows how artificial intelligence can change the way risk managers prepare for black swan events. By generating scenarios that go beyond historical data and traditional risk models, StressGen helps financial institutions build more resilient systems and processes that can make all the difference in how markets and institutions respond to future crises.
Curious about how StressGen can make your institution more resilient? Get in touch with Mathieu Tancrez to learn more.