"I'm not afraid of storms, for I'm learning how to sail my ship." — Louisa May Alcott
Introduction
The rise of artificial intelligence (AI), or generative AI, to be specific, has sparked obvious concerns and fears within the ART world, with many believing that AI will replace human artistic expression. To fight back, artists have filed class action lawsuits in the US against Midjourney, Stable Diffusion, and DeviantArt [1], organized protests, and boycotted online services like ArtStation that allowed image-generated content on their platforms. They continue to raise awareness about the impact of image generators on their profession and communities [2], [3]. These concerns are valid and understandable, as AI is undoubtedly a transformative technology that could profoundly disrupt the way we understand ART today.
There is no doubt that [4] generative AI is a new medium that will fundamentally alter the creative processes of artists, but it is not necessarily the "end of art," as some may fear. Generative AI will require artists to find new ways to exert their artistic intention and rigor, such as in selecting training data, crafting prompts, and using AI-generated artifacts for downstream applications. There are concerns regarding how the aesthetics and biases embedded in generative AI models will affect the diversity and range of artistic outputs. Generative AI is positioned to upend many sectors of the creative industry, threatening existing jobs and labor models in the short term while ultimately enabling new roles, genres, and aesthetics of art.
If we look at the history of technological advancements, it was often met with initial fear and resistance before ultimately benefiting society. For instance, the introduction of the printing press, the industrial revolution, and even the internet were all met with apprehension and skepticism at first, but they eventually became catalysts for progress, knowledge propagation, and economic growth. The same is true for the ART industry as well. For instance, the invention of photography and then digital ART was initially met with skepticism and resistance from traditional artists[5], but these technologies have now become integral tools in the artistic process, enabling new forms of expression and creativity. AI has the potential to enhance and complement artistic expression in novel ways. By automating repetitive tasks, AI can free up human ingenuity for more creative and fulfilling endeavors, ultimately enhancing the quality of work within the realm of ART.
The concerns about the exploitation of artists, such as through the training of AI models on their artwork without permission, displacement of artists, and the shifting of profits away from individual creators to the owners of the generative AI models, are all valid. And we as a community should not remain oblivious of impending danger. The AI undoubtedly poses risks if misused or developed without proper safeguards. However, there are concerted efforts underway to ensure its responsible development and governance. Ethical principles, such as transparency, accountability, and respect for human rights, are being integrated into AI systems to mitigate potential negative consequences.
It is crucial to approach AI with a balanced and thoughtful perspective, acknowledging both its risks and opportunities. Rather than succumbing to fear, we should embrace the responsible development and governance of AI, ensuring that it remains a tool for human betterment and not a source of harm.
History of ART — How ART and Artists Evolved with Technology
Throughout history, technological advancements have significantly impacted the art world. [6] For instance, the development of new pigments and materials has enabled artists to explore new techniques and styles, while scientific studies of light, color, and perception have informed artistic theories and practices. The invention of oil paints in the 15th century allowed artists to achieve greater depth and realism in their paintings. The development of photography in the 19th century challenged the traditional role of art in representing reality and led to the emergence of new artistic movements like Impressionism. Figure Fig1 lists the inventions from past to current that have helped shape ART the way it is today.
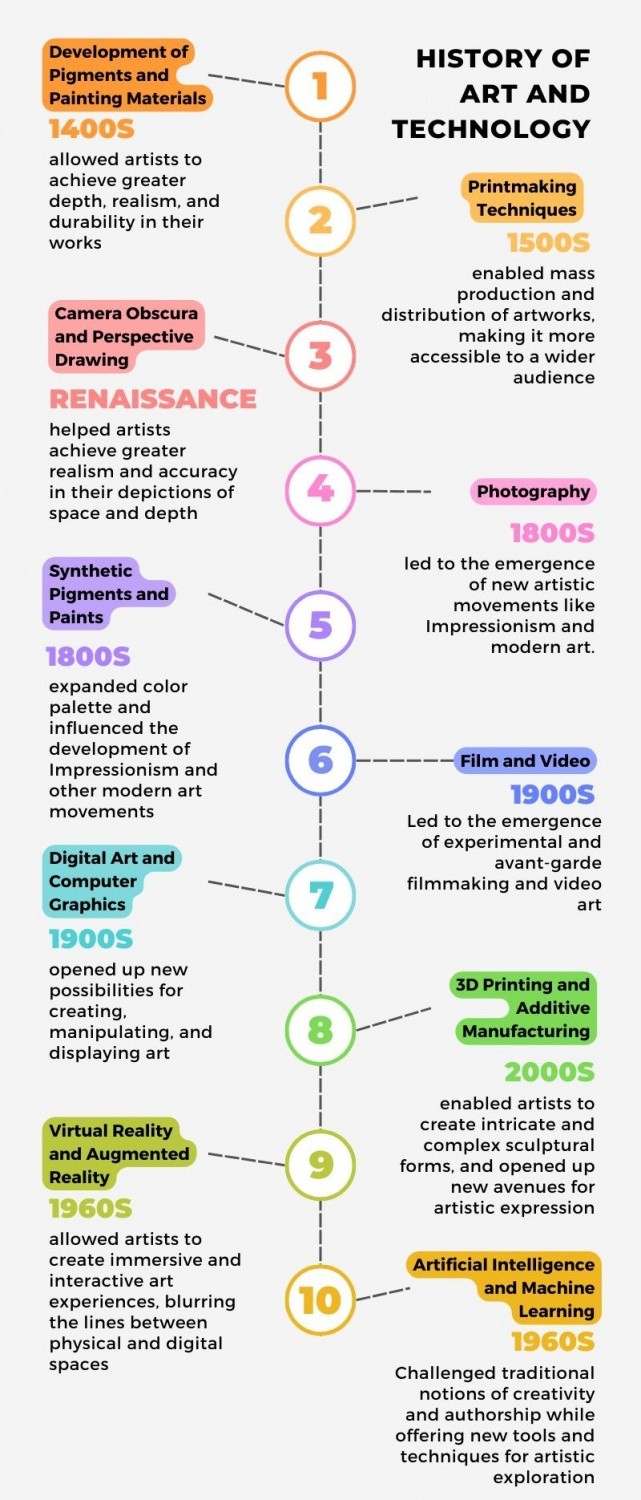
Similar to these past innovations, AI-generated art has the potential to reshape the artistic process and our understanding of creativity. Like the introduction of new tools or materials, AI systems provide artists with a novel medium to explore and express themselves.
However, AI-generated art differs from past technological advancements in its ability to create artworks autonomously without direct human input. And hence, raises questions about the role of the artist when the AI system plays a significant part in the creative process. This challenges the traditional notion of art as a purely human endeavor and raises debates about authorship, originality, and the nature of creativity. I will talk more about this in the section "Perception vs Reality." For now, let's first delve into the techniques behind some of the most popular Generative AI frameworks and models. Having some understanding of how these models work is essential to evaluate their strengths and limitations and identify potential biases or ethical concerns that may arise from the training data or algorithms used.
GenAI Techniques and Models for Artistic Expression
Majority of the AI-ART-generator tools that exist in the market today are based on three main generative AI technologies [7], [8], [9], [10], [11]. These are:
Neural Style Transfer (NST)
Neural Style Transfer (NST) leverages convolutional neural networks to separate the "content" and "style" representations of images and then recombines them to generate a new stylized image. That is, with this technique, you can combine the content of one image with the style of another image, creating a new image that resembles the content of the first image but with the artistic style of the second image. For example, [11] taking the content of a photograph (e.g., a landscape scene) and combining it with the artistic style of a famous painting (e.g., Van Gogh's Starry Night) and thus creating a new image that blends the two.
Generative Adversarial Networks (GANs)
Generative Adversarial Networks (GANs) are a prominent class of machine learning framework for generative AI. It consists of two neural networks—a generator and a discriminator—that are trained in a competitive manner. The generator tries to produce realistic-looking images, while the discriminator tries to distinguish the generated images from real ones. The discriminator's job is to tell how "realistic" the input seems, and the generator's job is to fool the discriminator. This enables the model to learn in an unsupervised manner. The generator-discriminator combo can together generate an output that would seem authentic to human eyes based on the number of realistic characters it has.
AICAN (Artificial Intelligence Creative Adversarial Network)
Artificial Intelligence Creative Adversarial Network (AICAN) is an evolved version of the GAN-based approach that can generate creative art by maximizing the deviation from established artistic styles/patterns and minimizing deviation from the overall art distribution. That is, generate novel artworks but at the same time "not too novel." GAN, on the other hand, would simply emulate the previous data distribution, showing limited creativity.
The effectiveness of AICAN was assessed by having human evaluators regularly confuse the generated art with human-created art. [Cite?]
In summary, AICAN is an example of a GAN-based system that was specifically designed to generate creative, novel artworks by balancing originality and adherence to broader artistic norms. It demonstrates how the GAN framework can be adapted and extended to tackle the challenge of computational creativity.
These three generative AI technologies together have given birth to the rise of the AI Art movement. While NST methods are more focused on automated image manipulation, GANs, and AICAN aim to push the boundaries of machine creativity and generate truly novel artworks.
Role of AI in the Creative World
Artificial Intelligence (AI), specifically generative AI, can analyze huge amounts of data, spot patterns, and generate original outputs using machine learning algorithms. Generative AI can provide inspiration for artists by generating unique concepts, color palettes, textures, and compositions and even help with producing an initial draft, which artists can further refine and personalize. AI-powered tools can be used to automate mundane routine tasks such as image processing, color correction, or background removal, allowing artists to spend more time on the creative process that they enjoy most. Based on research by [12], where they analyzed 53,000 artists and 5,800 known AI adopters on one of the largest art-sharing platforms, it was found that upon AI adoption, artists experienced a 50% increase in productivity on average, which doubled in the subsequent month. For an average user, that means approximately 7 additional artifacts published in the adoption month and 15 artifacts in the following month.
AI algorithms can produce or analyze techniques that are impossible or difficult for humans. For example, AI can help artists, students of art, and researchers to understand the brushwork, symmetry, balance, etc., in classic paintings of artists of the past. An understanding of these classic art techniques will deepen our appreciation of historical works and enable new-age artists and historians to discover intricate layers of visual art.
AI can increase the overall revenue from sales performance, both in terms of the number of sales and average sales price. Based on research by [13], they found that consumers are willing to pay higher prices for AI-generated content, which could be a valuable revenue stream for creators, especially those in the early stages of their careers. [12] found that the creative artifacts produced with the help of generative AI were evaluated 50% more favorably by peers over time.
AI-powered tools will be helpful to those groups of artists who are lesser known and have limited resources by making advanced techniques and resources available for their pursuit, thus helping to democratize art. [13] suggests that less productive and experienced creators benefit more from AI adoption, as it helps enhance their productivity and make up for their lack of experience.
In short, generative AI can be a very powerful tool when oriented towards the goal of enhancing human creativity rather than attempting to supplant it. One such example is the work of artist Anna Ridler, who created a piece called Mosaic Virus in 2019, generating her own training data by taking photos of 10,000 Tulips, which itself is a work of art that she titled Myriad (Tulips). She then trained a GAN-based image generator with this data, creating a video in which the appearance of a tulip is controlled by the price of bitcoin [14].
Perception vs Reality
While there is a perception that AI is an autonomous technology that can operate independently, the reality is that current AI systems are not truly as autonomous as the media claims them to be. We need to separate fact from fiction. AI technologies that exist today still require significant human involvement and oversight in most applications. While researchers are actively working on developing more autonomous and self-improving AI systems, the current state of the technology still relies heavily on human expertise, oversight, and collaboration. As AI continues to advance, the balance between human and machine autonomy will likely shift, but it is unlikely that AI will become truly autonomous and independent of human involvement in the near future.
While the field has made remarkable progress in areas such as machine learning, natural language processing, and computer vision, the current state of AI is still narrow and specialized. AI systems excel at specific tasks but lack the general intelligence and autonomy that many people envision. Even in areas where AI has made significant progress, such as computer vision, natural language processing, and decision-making, human involvement is still essential. For example, AI systems often require large datasets curated and labeled by humans for training. Humans are needed to define the objectives, constraints, and ethical guidelines for AI decision-making. Human oversight is necessary to monitor AI systems for potential biases, errors, or unintended consequences. AI systems may struggle with edge cases or novel situations that require human intervention or retraining.
Based on the interesting research done by [12] on the art-sharing platform, they have observed that the average content and visual novelty diminished over time, but the peak content novelty increased, the novelty being a measure of creativity (focal objects, object relationships, pixel-level stylistic elements). This implies that while the adoption of generative AI could expand the universe of creative possibilities, their widespread adoption could lead to a long-run equilibrium where many artifacts converge to the same types of content or visual features, potentially leading to a proliferation of generic content.
People's perception of AI is also a very complex and multifaceted topic. There is a general preference for human-created art over AI-generated art. [15] Studies have found an "algorithm aversion" phenomenon, where people tend to underestimate the aesthetic quality of AI-generated art. This bias against AI art exists even among younger generations, who are more accepting of the technology.
[12], [15] Both have found in their experiments that while text-to-image AI can enhance human creativity and lead to more favorable evaluations of the resulting artworks, there is a consistent reduction in the average visual and content novelty over time. [15] notes that while AI-generated images can be highly realistic and difficult for humans to distinguish from human-made art, there are still certain defects or cues that can allow people to identify the AI origin. Image generators have no understanding of the perspective of the audience or the experience that the output is intended to communicate to this audience [16]. The output from image generators is aesthetic, meaning they can be appreciated or enjoyed, but they are not artistic. To be artistic, a work must also be esthetic—that is, framed for enjoyed receptive perception. [17].
[11] AI tools show promise, but the true artistic value lies more in how the human orchestrates and designs the latent space in which the AI system operates. An image generator is trained to generate images from prompts by mapping images and texts into a lower dimensional representation in a latent space [18], [19], [20]. This latent space is learned during the model's training process. Once the model is trained, this latent space is fixed and can only change through training from scratch or finetuning on additional examples of image-text pairs [21]. Human creativity, in contrast, varies continuously with new experiences, and a human's lived experiences evolve over time. Most importantly, these experiences are not dependent on training on an input of images. That is, while generative AI can enhance human creativity in certain ways, it also has limitations in terms of maintaining consistent novelty and originality. The human's role in ideation, filtering, and orchestrating the AI's creative process appears to be crucial in determining the artistic merit of the final output. [12] also suggest that artists who can successfully explore novel ideas and curate AI-generated outputs are able to produce artworks that are evaluated more favorably by their peers.
Ethical Concerns and Challenges Surrounding AI-Generated Art
Amidst all the productivity, automation, opportunities, and new possibilities that AI brings to the ART world, it also raises several ethical concerns. There are questions about who owns the intellectual property rights for AI-generated artworks, as the AI system is essentially "borrowing" from existing works in its training data. Like many AI systems, the algorithms used for art generation can perpetuate biases present in their training data. This could result in a lack of diversity and representation in the generated artworks, potentially reinforcing existing societal biases. AI generators have been shown to appropriate and distort the identities of groups, encode biases, and reinforce stereotypes [22], [23], [24]. Some artists and creatives express concerns that AI-generated art could devalue human creativity, potentially leading to job displacement or making it harder for human artists to earn a living from their work. There are also questions about the transparency, explainability, and accountability of AI art generation systems, particularly when it comes to understanding how they arrive at certain outputs or addressing potential harmful or unethical content.
Students who anticipate image generators replacing artists have become demoralized and dissuaded from developing their craft and style [2]. Not only that, but existing artists are becoming increasingly reluctant to share their works and perspectives in an attempt to protect themselves from the mass scraping and training of their life's works [2], [3]. Independent artists share their work on social media platforms and crowdfunding campaigns and sell tutorials, tools, and resources to other artists on various sites or at art-centric trade shows. They do these in an attempt to gain visibility among the competition. It takes them years to build an audience and fanbase to sell their work. Fearing AI, they now choose not to share their work, which impacts their ability to receive compensation. This could also limit the large-scale adaptation of AI-generated ART, as described in the section on "Future Possibilities for ART."
Role of Legal Frameworks and Intellectual Property Rights Policies
In order to prevent harm to human society and ART, it is important that we explore new frameworks for intellectual property rights, ensure diverse and unbiased training data, protect the livelihoods of human artists, and establish transparency and accountability measures for AI systems. Ultimately, as with any emerging technology, it is important to strike a balance between fostering innovation and addressing potential ethical challenges to ensure responsible development and deployment.
There have already been several proposals put forth by artists, the art community, researchers, and lawmakers. One example is the proposed regulation by Arte es Ética that calls for legislation that requires the explicit consent of content creators before their material is used for generative AI models [25]. They suggest having detection and filtering algorithms to ensure that uploaded content belongs to creators who have consented to their work being licensed or opted-in for use as training data. They also suggest having a metric to quantify the amount of human interaction with an image generator to determine whether or not a generated image is copyrightable, with a 25% or less interaction level being uncopyrightable. [26] recommends ensuring artists are fairly compensated when their works are used to train generative AI systems or provide protections against their displacement.
In a letter to members of The Costume Designers Guild, Local 892, a union of professional costume designers, assistant costume designers, and illustrators working in film, television, commercials, etc., suggested legislation to allow "using AI derived imagery strictly for reference purposes and making it unacceptable to hand over a fully AI generated work as a finished concept" [27].
There are existing lawsuits that are filed against Stability AI over its Stable Diffusion image generator[26]. The outcomes of these cases could set important legal precedents and help clarify how copyright law applies to generative AI systems and the use of artists' works.
Even in the past, disruptive technologies have been a challenge for the prevailing copyright laws, leading to legal battles, court rulings, and sometimes legislative updates to adapt the legal framework. [26] notes that the law and policy often evolve much slower than the rapid pace of technological change. [26] Technologies like player pianos, cable TV, photocopiers, and MP3 players have faced copyright industry challenges in the past. While many of these legal challenges failed in court, Congress sometimes later extended protections in the aftermath. Courts typically try to balance the legitimate interests of copyright owners with the needs of developers and follow-on creators when new technologies pose unforeseen copyright questions. This could involve finding the right balance between preventing the misappropriation of artists' works while also allowing some "breathing space" for innovative technologies like generative AI to develop.
Future Possibilities for ART
Looking to the future, the one thing that is guaranteed is a significant disruption in the way we see and understand ART. This will be done by introducing new perspectives, challenging traditional boundaries, and offering novel ways of creating, analyzing, and experiencing art. Now, whether the impact would be positive or painful will depend on how artists, curators, policymakers, technologists, and the broader art community choose to embrace and integrate these technologies.
The future can be a bright one where we see AI becoming a powerful tool for artists, assisting in the creative process by generating ideas, exploring new styles, and even collaborating with human artists. It is used by organizers of art exhibitions and galleries, who select and arrange artworks based on various criteria, such as themes, styles, and emotional impact. Where it is used to create immersive and interactive art experiences where the artwork adapts and responds to the viewer's presence, movements, and emotions. Where it provides deeper insights into artworks, analyzing not only the visual elements but also the underlying meanings, cultural contexts, and emotional resonance.
However, it could also become one where Artists' reluctance to share their work and teach others reduces the ability of prospective artists to learn from experienced ones, limiting the creativity of humans as a whole. Similar to the feedback loop created by the next generations of large language models trained on the outputs of previous ones [28], if we, as humanity, rely solely on AI-generated works to provide us with the media we consume, the words we read, the art we see, we would be heading towards an ouroboros where nothing new is truly created, just a stale perpetuation of the past. In [16], the authors warn against a similar issue with future generations of large language models trained on outputs of prior ones and static data that do not reflect social change.
The future may see a shift in the focal skills required for artists, where ideation proficiency and the ability to curate and filter AI-generated content become more important than pure mechanical skill [12]. This "generative synesthesia"—the harmonization of human exploration and AI exploitation—may open new opportunities for creative exploration, where artists can focus more on the ideas they represent rather than the technical execution.
While concerns about automation exist, the future may also see a more democratized and inclusive creative domain where generative AI empowers a wider range of artists.
The authors of [10] believe that deep learning approaches will continue to evolve rapidly, paving the way for computer systems to analyze and understand fine arts in the future autonomously.
In summary, AI will definitely play a prominent role in the art world, with the potential to fundamentally alter how art is created, analyzed, and understood. The future of art during the generative AI era will possibly be one of both challenges and opportunities. Adapting to the new medium, addressing issues of exploitation and displacement, and finding ways to leverage the strengths of human-AI collaboration will be crucial for the continued evolution and flourishing of art in the years to come. Technology firms and policymakers must be sensitive to the potential consequences of generative AI in creative fields and society more broadly.
Conclusion
In conclusion, while the concerns about AI are understandable, history has shown that technological advancements, when approached responsibly and ethically, can ultimately benefit humanity. By fostering a collaborative and inclusive approach to AI development, we can harness its potential while mitigating its risks, paving the way for a future where humans and AI coexist harmoniously.
Generative AI possesses the capability to become an excellent tool for art when the data they are trained on is not created using someone's unpaid effort and when their production is not meant to replace jobs. The true value of AI for ART is its ability to empower and complement human artists in expanding the bounds of creativity. When viewed as a powerful tool to augment an artist's vision and skills, there is no limit to what we can achieve. [5] says that as long as artificial intelligence enables artists to do more interesting work without completely displacing them, it is generally a force for good.
References
[1] "AI art tools Stable Diffusion and Midjourney targeted with copyright lawsuit — The Verge." Accessed: Jul. 07, 2024. [Online]. Available: https://www.theverge.com/2023/1/16/23557098/generative-ai-art-copyright-legal-lawsuit-stable-diffusion-midjourney-deviantart
[2] The problem with AI-generated art | Steven Zapata | TEDxBerkeley, (Oct. 12, 2023). Accessed: Jul. 07, 2024. [Online Video]. Available: https://www.youtube.com/watch?v=exuogrLHyxQ
[3] "Why AI Models are not inspired like humans.," KOrtiz Blog. Accessed: Jul. 07, 2024. [Online]. Available: https://www.kortizblog.com/blog/why-ai-models-are-not-inspired-like-humans
[4] Z. Epstein et al., "Art and the science of generative AI," Science, vol. 380, no. 6650, pp. 1110–1111, Jun. 2023, doi: 10.1126/science.adh4451.
[5] A. Ghosh and G. Fossas, "Can There be Art Without an Artist?" arXiv, 2022. doi: 10.48550/ARXIV.2209.07667.
[6] The Cover Design, ART, TECHNOLOGY, AND SCIENCE: NOTES ON THEIR HISTORICAL INTERACTION, and CYRIL STANLEY SMITH, "ART, TECHNOLOGY, AND SCIENCE: NOTES ON THEIR HISTORICAL INTERACTION."
[7] N. Derevyanko and O. Zalevska, "Comparative analysis of neural networks Midjourney, Stable Diffusion, and DALL-E and ways of their implementation in the educational process of students of design specialities," Sci. Bull. Mukachevo State Univ. Ser. Pedagogy Psychol., vol. 9, no. 3, pp. 36–44, Jul. 2023, doi: 10.52534/msu-pp3.2023.36.
[8] D. Hanna, "The Use of Artificial Intelligence Art Generator 'Midjourney' in Artistic and Advertising Creativity," J. Des. Sci. Appl. Arts, vol. 4, no. 2, pp. 42–58, Jun. 2023, doi: 10.21608/jdsaa.2023.169144.1231.
[9] J. Ploennigs and M. Berger, "Analysing the usage of AI art tools for architecture," presented at the 2023 European Conference on Computing in Construction and the 40th International CIB W78 Conference, Jul. 2023. doi: 10.35490/EC3.2023.253.
[10] G. Castellano and G. Vessio, "Deep learning approaches to pattern extraction and recognition in paintings and drawings: an overview," Neural Comput. Appl., vol. 33, no. 19, pp. 12263–12282, Oct. 2021, doi: 10.1007/s00521-021-05893-z.
[11] E. Cetinic and J. She, "Understanding and Creating Art with AI: Review and Outlook," ACM Trans. Multimed. Comput. Commun. Appl., vol. 18, no. 2, pp. 1–22, May 2022, doi: 10.1145/3475799.
[12] E. Zhou and D. Lee, "Generative AI, Human Creativity, and Art," SSRN Electron. J., 2023, doi: 10.2139/ssrn.4594824.
[13] P. Xie and X. Li, "When AI Meets Art: the Algorithm Appreciation Effect in Creative Content," SSRN Electron. J., 2024, doi: 10.2139/ssrn.4814916.
[14] "Anna Ridler uses AI to turn 10,000 tulips into a video controlled by bitcoin." Accessed: Jul. 07, 2024. [Online]. Available: https://www.itsnicethat.com/articles/anna-ridler-digital-art-280619
[15] J. Oppenlaender, J. Silvennoinen, V. Paananen, and A. Visuri, "Perceptions and Realities of Text-to-Image Generation," in 26th International Academic Mindtrek Conference, Tampere Finland: ACM, Oct. 2023, pp. 279–288. doi: 10.1145/3616961.3616978.
[16] H. H. Jiang et al., "AI Art and its Impact on Artists," in Proceedings of the 2023 AAAI/ACM Conference on AI, Ethics, and Society, Montr'{e}al QC Canada: ACM, Aug. 2023, pp. 363–374. doi: 10.1145/3600211.3604681.
[17] "Art as Experience in: The Richness of Art Education." Accessed: Jul. 07, 2024. [Online]. Available: https://brill.com/display/book/9789087906092/BP000003.xml
[18] I. J. Goodfellow et al., "Generative Adversarial Networks." arXiv, 2014. doi: 10.48550/ARXIV.1406.2661.
[19] D. P. Kingma and M. Welling, "Auto-Encoding Variational Bayes." arXiv, 2013. doi: 10.48550/ARXIV.1312.6114.
[20] R. Rombach, A. Blattmann, D. Lorenz, P. Esser, and B. Ommer, "High-Resolution Image Synthesis with Latent Diffusion Models." arXiv, 2021. doi: 10.48550/ARXIV.2112.10752.
[21] I. Goodfellow, Y. Bengio, and A. Courville, Deep Learning. MIT Press, 2016.
[22] L. Nicoletti and D. B. T. + Equality, "Humans Are Biased. Generative AI Is Even Worse," Bloomberg.com, Jul. 02, 2024. Accessed: Jul. 07, 2024. [Online]. Available: https://www.bloomberg.com/graphics/2023-generative-ai-bias/
[23] R. Qadri, R. Shelby, C. L. Bennett, and E. Denton, "AI's Regimes of Representation: A Community-centered Study of Text-to-Image Models in South Asia," in 2023 ACM Conference on Fairness, Accountability, and Transparency, Chicago IL USA: ACM, Jun. 2023, pp. 506–517. doi: 10.1145/3593013.3594016.
[24] R. Srinivasan and K. Uchino, "Biases in Generative Art — A Causal Look from the Lens of Art History." arXiv, Feb. 16, 2021. Accessed: Jul. 07, 2024. [Online]. Available: https://arxiv.org/abs/2010.13266
[25] "Art is Ethics — Artivism and Authors of Hispana Speak." Accessed: Jul. 07, 2024. [Online]. Available: https://arteesetica.org/
[26] P. Samuelson, "Generative AI meets copyright," Science, vol. 381, no. 6654, pp. 158–161, Jul. 2023, doi: 10.1126/science.adi0656.
[27] "The Costume Designers Guild, Local 892. 2022. A Letter to Membership. (2022)."
[28] E. M. Bender, T. Gebru, A. McMillan-Major, and S. Shmitchell, "On the Dangers of Stochastic Parrots: Can Language Models Be Too Big? ," in Proceedings of the 2021 ACM Conference on Fairness, Accountability, and Transparency, Virtual Event Canada: ACM, Mar. 2021, pp. 610–623. doi: 10.1145/3442188.3445922.