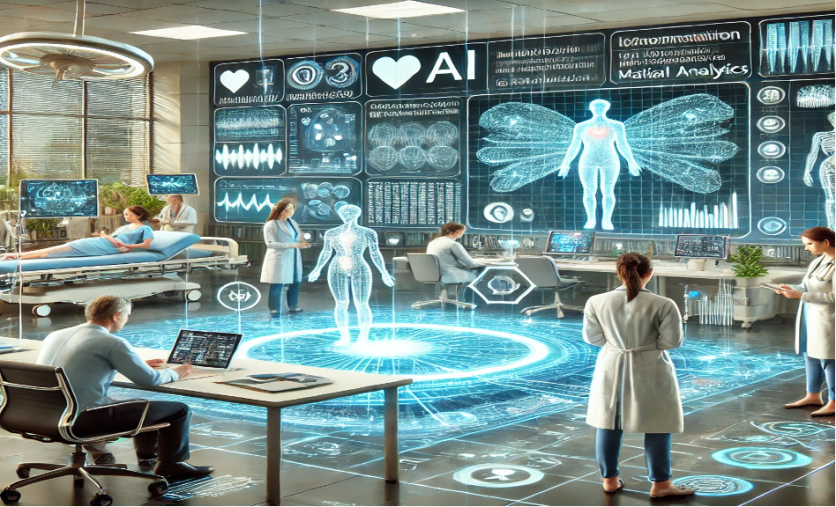
Artificial intelligence (AI) has transformed various sectors, and its potential in healthcare is nothing short of revolutionary. Despite its imperfections, AI in healthcare offers potential benefits such as improved diagnostics, personalized medicine, and enhanced patient care.
Nonetheless, there is a significant need for a system to guide the integration and application of AI technologies. This article explores the current challenges, reluctance to adopt, and the technical intricacies of AI, including large language models (LLMs), providing future guidance for effectively implementing AI in healthcare.
The Imperative for a Standard Framework
The diverse applications of AI in healthcare, from diagnostics to personalized medicine, signal the critical and urgent need for a standardized framework. Such a framework results in consistent outcomes, inefficiencies, and potential risks to patient safety.
A standardized framework would ensure consistency in data collection, algorithm development, validation processes, and ethical considerations. This would bolster the reliability and trustworthiness of AI applications and facilitate their seamless integration into healthcare systems.
The Imperfections of Current AI Systems
Despite the advancements in AI, the technology is far from perfect. One of the primary challenges is the quality and diversity of data. Artificial intelligence systems, particularly those based on machine learning (ML) and LLMs, require vast amounts of high-quality data to function effectively. However, healthcare data is often fragmented, inconsistent, and biased.
This can lead to inaccurate predictions and perpetuate existing healthcare disparities. Acknowledging these challenges is the first step towards overcoming them, making the demographic feel understood and validated in their concerns.
Reluctance in Adoption
Healthcare professionals understandably hesitate to trust AI systems when they cannot fully understand or explain how the decisions are made. They are trained to rely on evidence-based practices and clinical judgment, and the opacity of AI algorithms makes it difficult for them to place their trust in these systems.
Regulatory hurdles, as well, contribute to the slow adoption of AI. The healthcare sector is heavily regulated, and introducing new technologies requires extensive validation and approval processes. Without explicit regulatory guidelines specific to AI, healthcare organizations are reluctant to invest in and deploy these technologies.
Technical Challenges: Large Language Models (LLMs)
Large language models (LLMs) like GPT-4 have shown great promise in various applications, including natural language processing (NLP) and predictive analytics. However, their application in healthcare is fraught with challenges. LLMs require extensive computational resources and large datasets to train effectively. Acquiring such datasets can be difficult in healthcare due to privacy concerns and the need for patient consent.
Moreover, LLMs can inadvertently learn and propagate biases present in the training data. This can have profound implications in healthcare, leading to biased treatment recommendations and perpetuating health inequities. Ensuring that LLMs are trained on diverse and representative datasets mitigates these risks.
Future Guidance and Recommendations
The current U.S. Administration has also released the first-ever guidance to federal agencies on the responsible use of AI. This highlights the importance of regulating AI processes and the need to standardize practices.
Develop and Implement a Standard Framework
To overcome the current challenges, developing and implementing a standardized framework for AI in healthcare is imperative. This framework should encompass guidelines for data collection, algorithm development, validation, and ethical considerations. It should be developed collaboratively by industry experts, regulatory bodies, and healthcare professionals to ensure comprehensiveness and applicability.
Enhance Data Quality and Diversity
Improving the quality and diversity of healthcare data is essential for the effective functioning of AI systems. Organizations should invest in robust data governance practices, including standardized data formats, rigorous data validation processes, and continuous data quality monitoring mechanisms.
Promote Transparency and Explainability
Transparency and explainability are crucial for building trust in AI systems. Developers should prioritize the creation of explainable AI models that allow healthcare professionals to understand and interpret the underlying decision-making processes.
Foster Collaboration and Education
Collaboration between healthcare providers, technologists, and regulatory bodies is essential for successfully integrating AI. Regular communication and knowledge-sharing can facilitate the identification of innovative applications and the resolution of potential challenges.
Address Ethical and Regulatory Challenges
Addressing ethical and regulatory challenges is essential for the responsible use of AI in healthcare. Clear regulatory guidelines specific to AI should be developed to streamline the validation and approval processes. Ethical oversight committees should be established to monitor AI applications and ensure they align with ethical standards and patient rights.
Focus on Scalability and Integration
Developing AI solutions that are scalable and seamlessly integrated into existing healthcare systems is crucial for widespread adoption. Efforts should be made to ensure compatibility with existing electronic health record (EHR) systems and other healthcare technologies.
Prioritize Equity and Access
Ensuring that AI technologies are accessible to all patients, regardless of socioeconomic status or geographic location, is essential for reducing healthcare disparities. AI tools should be designed to be culturally sensitive and adaptable to diverse patient populations.
Continuous Monitoring and Evaluation
Continuous monitoring and evaluation are essential to ensure that AI systems perform as intended and deliver the desired outcomes. Healthcare organizations should establish mechanisms to regularly assess AI tools, including performance metrics, user feedback, and clinical outcomes. This ongoing evaluation helps identify areas for improvement and ensures that AI technologies remain practical and relevant.
The integration of AI in healthcare holds immense potential to transform the patient care system. However, achieving this potential requires healthcare organizations to collaborate, promote transparency, and prioritize equity. As the industry continues to refine these technologies, the future of AI in healthcare has the potential not only to revolutionize patient care but also to improve outcomes for all.
Author Bio
Amit Srivastava is an information technology expert with over a decade of experience integrating AI and advanced technologies into healthcare systems. He has been pivotal in developing and implementing innovative AI-driven solutions that significantly enhance system efficiency and operational effectiveness.