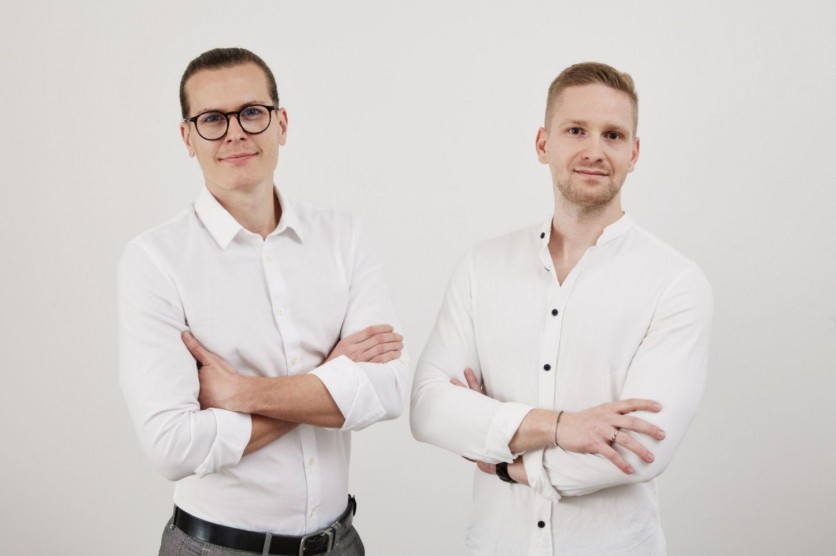
Imagine autonomous drones navigating the trickiest terrains, self-driving cars reaching Level 5 autonomy, and robots revolutionizing manufacturing. DiffuseDrive's technology makes these visions a reality, tackling the toughest AI data challenges with unparalleled photorealism and speed. Machines need vision to see and understand their environment, and their vision must be trained with examples: visual data. Solving the data layer for developers will enable machines to make human life fairer, more accessible, and more effective. DiffuseDrive's groundbreaking technology generates photorealistic visual data, empowering vision AI systems across diverse industries.
The cornerstone of effective AI development lies in access to high-quality, diverse, and abundant datasets. These datasets are crucial for training machine learning models in computer vision, where the ability to recognize and interpret visual information accurately is paramount. Collecting and annotating real-world data is fraught with challenges. Capturing data in various lighting conditions, weather scenarios, and diverse environments can be prohibitively expensive and time-consuming. Moreover, some situations, such as rare safety-critical events, are difficult to capture in sufficient quantity, limiting the robustness of the AI models trained on this data.
Furthermore, real-world data often suffers from noise, unavailability, and biases that can hinder model performance. Data availability is a significant challenge, particularly for niche applications where relevant data may be scarce or difficult to obtain. Biases in data can result from historical inequalities or unrepresentative sampling, which can perpetuate existing disparities and reduce the generalizability of AI systems.
Using real-world data also raises ethical and privacy issues, particularly when dealing with sensitive information. For example, collecting data from surveillance cameras or medical devices can infringe on individuals' privacy rights if not handled with care. Ensuring compliance with regulations such as the California Consumer Privacy Act (CCPA) or General Data Protection Regulation (GDPR) and maintaining the confidentiality of personal information are critical concerns that can complicate data collection efforts and are essential for protecting privacy and ethical considerations in AI development.
Revolutionizing AI with Synthetic Data
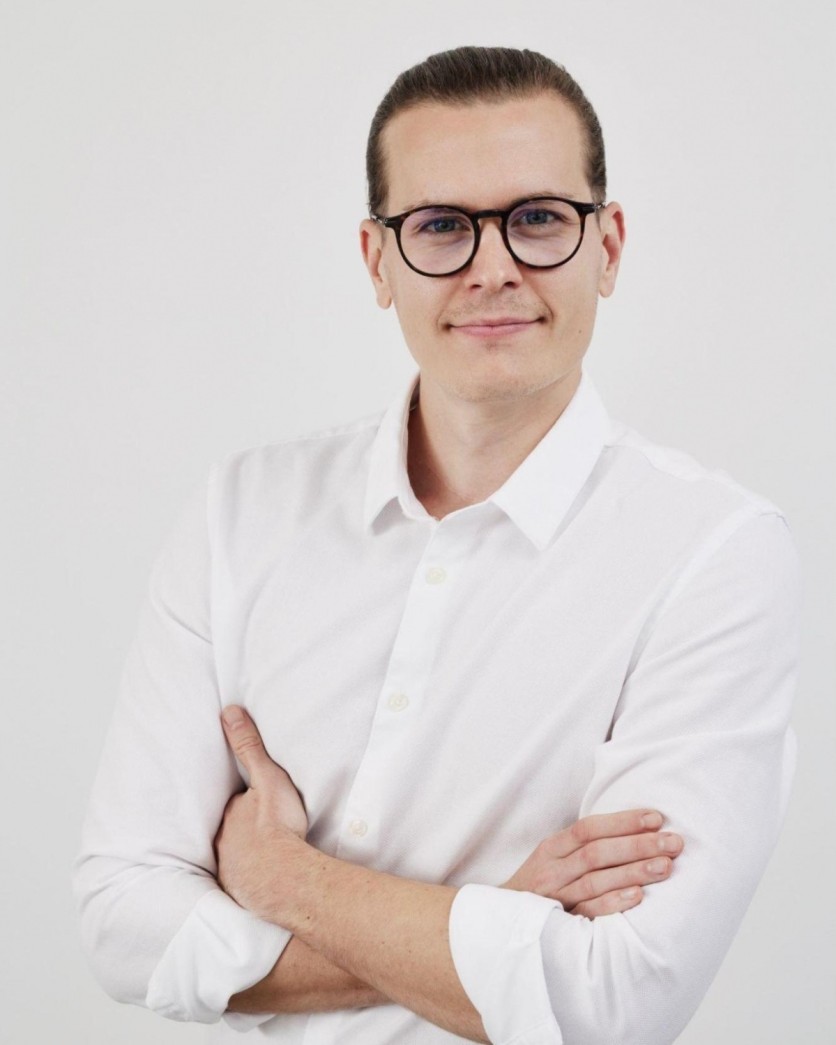
Balint Pasztor, CEO of DiffuseDrive, has a rich background in autonomous driving, having led an internal startup at Bosch. His experience has given him a unique perspective on the challenges faced in AI development and the potential of synthetic data to address these issues. He emphasizes the transformative potential of synthetic data. Synthetic data offers a scalable and flexible alternative, addressing many of these challenges. It is artificially generated data that mimics the real world and is used for training and testing AI models. Since synthetic data is inherently anonymized, containing no personally identifiable information (PII), it ensures high quality and diversity without ethical and privacy concerns.
Historically, existing synthetic data solutions have often relied on graphics engines that power video games. While these engines are excellent for rendering visually appealing scenes, they fail to encompass the complexities of the real world. They use approximations and finite equations to describe the infinite physical world, leaving out important details that encompass the intricacies of the real world. Consequently, AI models trained on such synthetic data may struggle to generalize to real-world applications.
Advancing AI with Diffusion Models
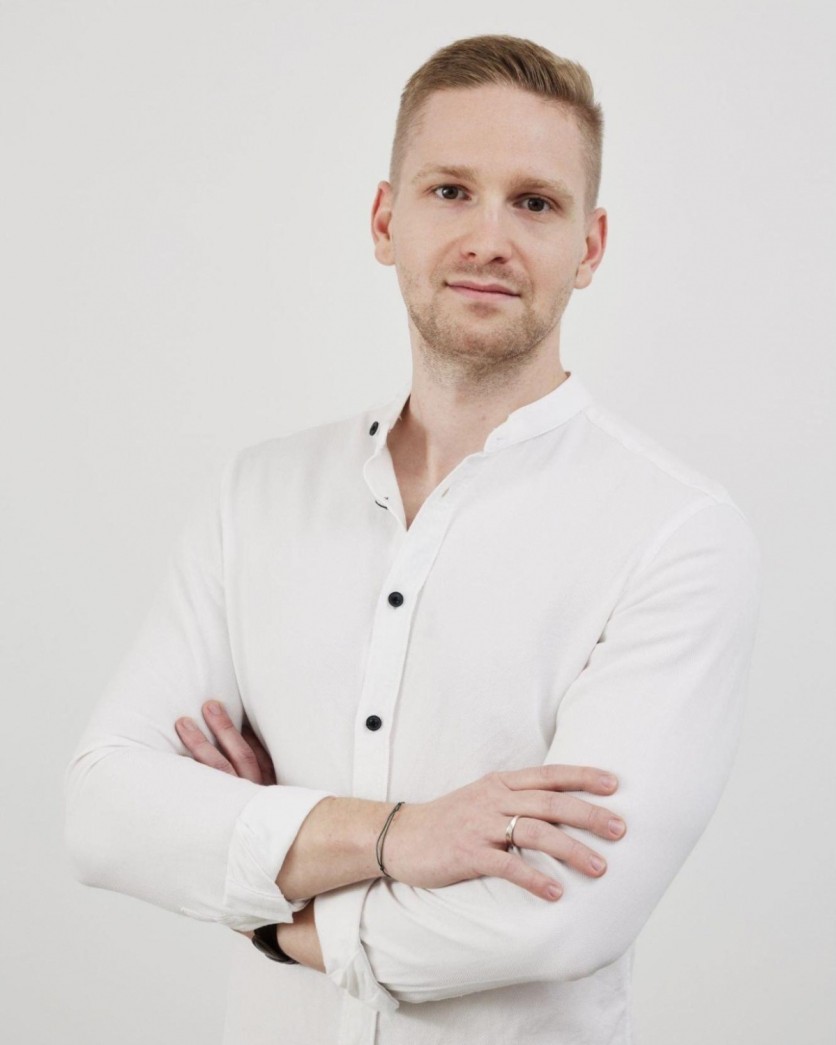
Roland Pinter, CTO of DiffuseDrive, brings a wealth of experience from his previous role as the AI/ML lead at Bosch and his work with one of Europe's best generative AI teams at Docler. His technical expertise drives the innovation behind DiffuseDrive's synthetic data generation tools. DiffuseDrive leverages diffusion models to generate synthetic data that is photorealistic and meets the specific needs of their customers. Diffusion models are a class of generative models that learn to create data by reversing a gradual noise process, producing highly realistic and varied samples. This approach allows DiffuseDrive to create synthetic data that is indistinguishable from real-world data in terms of appearance and complexity.
This technology creates highly detailed and accurate data that replicates the exact characteristics of the customer's hardware, such as camera position and characteristics. This ensures that the synthetic data produced is tailored to the specific sensors and devices used by DiffuseDrive's customers, eliminating the need for extensive data pre-processing and adaptation. The DiffuseDrive approach produces data tailored to specific applications, ensuring that the synthetic data aligns perfectly with the real-world conditions under which the vision AI models will operate.
Imagine developing AI software for an autonomous drone's recognition system. The drone's camera captures data from specific angles and positions. Using DiffuseDrive's technology, synthetic data is generated that mirrors these exact conditions, ensuring seamless integration into existing machine learning pipelines. This level of customization and precision allows developers to train AI models that are highly accurate and reliable, capable of performing complex tasks in diverse real-world environments.
The Impact of DiffuseDrive's Synthetic Data Technology
With DiffuseDrive, developers can identify what data they have and generate what they don't have—encompassing complex edge-case situations and scenarios that are frequently present—building complete and representative datasets for the use case. This technology allows autonomous drones to perform complex tasks with high precision, improving efficiency in delivery services and enhancing safety in defense missions. Autonomous vehicles benefit from safer, more efficient transportation systems, reducing traffic congestion and environmental impact.
Industrial robots can perform intricate tasks with remarkable accuracy, leading to more efficient manufacturing processes and quicker turnaround times for goods. In construction, autonomous vehicles and machinery can operate in hazardous environments, reducing the risk to human workers and lowering construction costs. Precision farming AI systems are optimized, ensuring high-quality food production and addressing global food security issues.
Advanced AI-driven surveillance systems, powered by DiffuseDrive's synthetic data, enhance public safety by enabling quicker and more accurate responses to potential threats. Smart city infrastructure is improved, resulting in more livable and sustainable urban environments. In healthcare, synthetic data aids in the accurate diagnosis and monitoring of medical conditions, democratizing access to high-quality healthcare.
The future of AI is intricately linked to the quality of the data that trains it. DiffuseDrive's synthetic data generation technology offers a scalable, high-quality solution that addresses current challenges in AI development. By leveraging diffusion models with their proprietary process, DiffuseDrive sets new standards in the industry.