In an age where predicting future occurrences is tough, Artificial Intelligence (AI) has transformed predictive modeling. Conventional forecasting approaches that were limited in catching complicated data patterns are now increasingly being surpassed by superior AI systems. This article helps you learn about Anusha Yella's transformative AI innovations in predictive modeling, emphasizing the profound impact of deep learning, predictive analytics, and ensemble learning on accuracy and foresight.
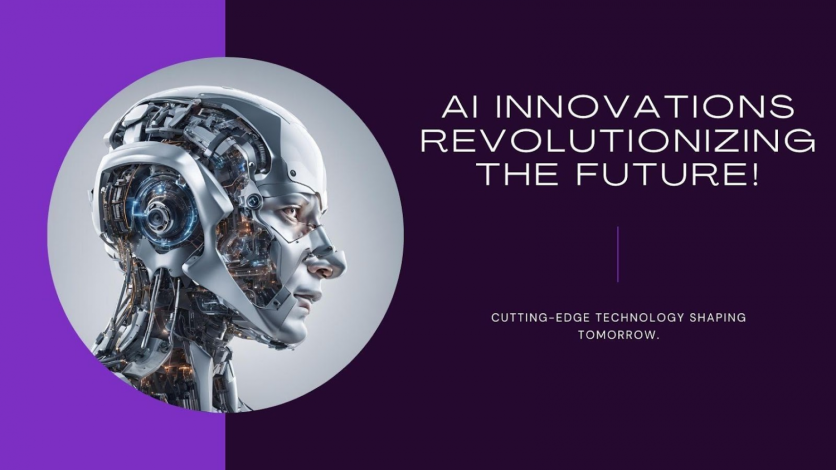
The Rise of Artificial Intelligence in Predictive Modeling
Artificial Intelligence has transformed predictive modeling by leveraging complex algorithms and vast datasets to forecast future events accurately. Traditional statistical methods often fail to capture intricate patterns in data, whereas AI excels in this area, enabling more accurate predictions across various domains.
Deep Learning: A Breakthrough in Pattern Recognition
Deep learning, a subset of AI, is key in predictive modeling. This technique uses neural networks that mimic the human brain's neural connections to identify and learn from complex patterns in data. Neural networks consist of interconnected nodes that process data through multiple layers, making them compellent for tasks such as image recognition, natural language processing, and time series forecasting.
In the financial sector, deep learning models have shown significant potential in predicting stock market trends. These models analyze historical data to forecast future stock prices, trading volumes, and market volatility. Anusha Yella's innovative approaches have resulted in more precise and reliable stock market predictions, significantly impacting investment strategies and risk management practices.
Predictive Analytics
Predictive analytics utilizes statistical algorithms to extract insights from historical and real-time data. Techniques such as regression models and decision trees enable AI systems to analyze patterns, recognize trends, and make well-informed predictions. Regression models establish connections between dependent and independent variables. They are valuable for predicting continuous values like sales figures or temperature readings.
Decision trees classify data based on a series of decisions or rules, making them robust for classification and regression problems. Ensemble techniques, such as random forests and gradient boosting, enhance the predictive power of decision trees. By combining multiple models, these techniques reduce overfitting and improve overall accuracy.
Ensemble Learning
Ensemble learning involves combining multiple models to enhance prediction accuracy and reliability. Anusha Yella's approach leverages the collective intelligence of diverse models, resulting in more dependable forecasts.
Bagging (Bootstrap Aggregating) trains several models on different subsets of the training data obtained through random sampling with replacement. The predictions from these models are combined through majority voting or averaging, reducing variance and overfitting. Algorithms like AdaBoost and Gradient Boosting Machines train a sequence of weak models, each focusing on instances misclassified by previous models, improving accuracy for complex and noisy data.
Stacking trains multiple base models on the same data and a meta-model to combine their predictions. The meta-model leverages the strengths of diverse base models, enhancing overall performance.
Real-World Applications and Impact
AI-driven predictive modeling has significant applications across various fields. In finance, deep learning models enhance stock market predictions, investment decisions, and risk management. Predictive analytics also improves fraud detection, portfolio optimization, and algorithmic trading.
In climate science, neural networks analyze extensive datasets from satellite imagery and weather stations to forecast climate variables like temperature and precipitation. These predictions are crucial for understanding and mitigating climate change impacts. In socio-economic analysis, AI models predict indicators like population growth and employment rates, guiding policymaking and resource allocation.
Challenges and Future Directions
Despite its impressive capabilities, AI-driven predictive modeling faces challenges such as data quality, model interpretability, and ethical considerations. Ensuring data quality and availability is crucial, as inaccuracies can significantly impact model performance, necessitating robust data preprocessing and augmentation techniques.
Model interpretability and transparency are critical, especially in sectors like healthcare and finance, to foster trust and accountability. Techniques like explainable AI (XAI) aim to develop models that offer comprehensible explanations for their predictions.
Ethical considerations, including algorithmic bias and privacy concerns, must be addressed to ensure responsible AI deployment. Proactive governance and regulation are necessary to mitigate risks and ensure that AI technologies are used ethically and transparently.
To wrap up, Artificial Intelligence is transforming predictive modeling, offering unprecedented accuracy and foresight. By combining deep learning, predictive analytics, and ensemble learning, AI systems can capture intricate patterns, model complex relationships, and provide valuable insights across various domains. As AI continues to evolve, its impact on predictive modeling will grow, unlocking new possibilities and transforming how we anticipate and navigate the future.
Anusha Yella's research focuses on the revolutionary potential of AI in predictive modeling, highlighting the importance of continual innovation and ethical deployment to reap its benefits.