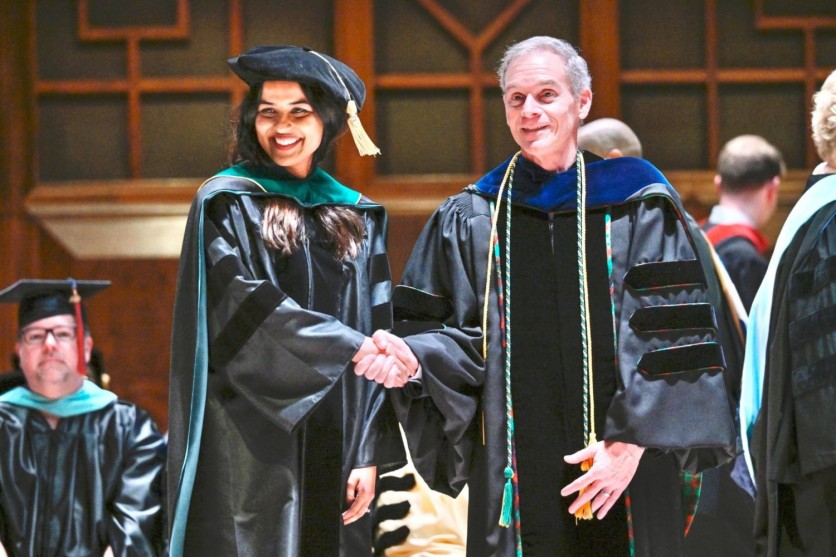
Data scientist Dr. Pranita Patil's curiosity and interest in technology ignited her pursuit of two master's degrees and a PhD in Data Science. This article delves into her research and explores how she develops algorithms to extract patterns and relationships in databases to recognize health conditions.
Q: Dr. Patil, thank you for joining us today. Could you share your journey into the world of machine learning and deep learning?
Dr. Pranita Patil: Since I was a teenager, I've been fascinated by machine learning and how deep learning models could be used to extract something meaningful from raw data. I successfully obtained my first master's degree in engineering, with a specific emphasis on machine learning, before achieving my second master's degree in Analytics and PhD in data science at Harrisburg University of Science and Technology. During my research, I delved into a variety of machine-learning techniques and crafted algorithms to uncover intricate patterns and connections within databases.
Q: Could you tell us about any specific projects you worked on during your undergraduate days?
Dr. Patil: Certainly! Back then, I collaborated on projects that involved converting speech to text using neural network methodologies and identifying fingerprints. These experiences fueled my passion for exploring the unknowns hidden within data.
Q: Impressive! And your journey continued with a Master of Science degree at Oklahoma State University. What did you work on there?
Dr. Patil: At Oklahoma State University, I had the opportunity to develop the very first Matlab toolbox for training and implementing deep networks. At the time of my master's degree research back in 2012, there was no tool available to experiment with deep architectures. This toolbox made it very easy for researchers to test their new ideas and design new architectures without having to write their own code and led to four publications. Since that time, the field has exploded, and there are now many frameworks for experimenting with deep neural networks.
Q: How did this project impact your career trajectory?
Dr. Patil: This project set the foundation for my professional career. After graduating, I joined AUM Cardiovascular as a Senior DSP Engineer. I explored and implemented machine learning and deep learning techniques on pre-processed data using digital signal processing techniques. One project I'm particularly proud of is developing machine learning algorithms to detect coronary artery disease at AUM Cardiovascular. I led the development of a machine learning algorithm for a noninvasive handheld device that collects heart sound data and performs acoustic analysis to rule out coronary artery disease. This project not only resulted in a patent but also has the potential to save lives by providing early diagnosis.
Q: Can you share more about your Ph.D. research and its impact?
Dr. Patil: My Ph.D. research focused on eliminating bias problems in deep learning models. I introduced novel techniques for Parkinson's disease recognition using rs-fMRI data for bias mitigation and for integrating the Granger causality framework in the deep learning domain. This research has significantly contributed to the field by improving the reliability of deep learning models and advancing bias mitigation techniques, making AI applications more trustworthy and effective.
Q: What inspired you to pursue a career in machine learning and deep learning?
Dr. Patil: The main reason I pursued this career was to explore the unknowns and unseen to the human eye. During my school days, my grandmother passed away due to a brain hemorrhage that went undetected. This personal experience made me question if technology could have detected the issue earlier. This curiosity led me to explore how data could reveal hidden patterns, which ultimately led me to my passion for machine learning and deep learning.
Q: So, to finish, your work benefits society by recognizing patterns in data that help identify and diagnose health conditions.
Dr. Patil: Yes, throughout my career, I have developed tools to improve diagnostic medicine, such as the detection of coronary artery disease, developing a new architecture of deep learning to perform breast cancer detection on mammograms, and using rs-fMRI data to detect Parkinson's disease. These tools help medical professionals make more accurate diagnoses and, in the end, ultimately save lives. My work in bias mitigation has helped to create fairer AI systems, which is so important for their ethical deployment.