Adobe researchers have created a new AI training method called FairDeDup in collaboration with an Oregon State University doctoral student. This approach aims to mitigate social biases inherent in AI systems by refining training datasets through a process termed fair deduplication.
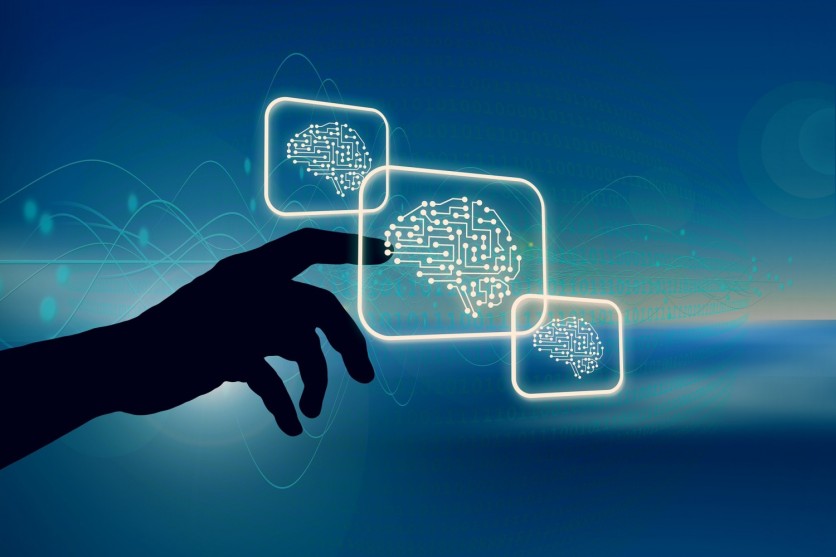
About FairDeDup of Oregon State University Student, Adobe Researchers
FairDeDup aims to address the challenge of biases in datasets sourced from the internet. The new technique ensures that AI systems are trained on diverse and representative data, thus enhancing accuracy while promoting fairness.
The research team, led by Eric Slyman from the university's College of Engineering, explained that FairDeDup utilizes a pruning process to selectively refine datasets of image captions, ensuring that only a subset of data that accurately represents the entire dataset is used for training.
This method streamlines the training process by reducing redundant data and integrates considerations of fairness by incorporating diverse dimensions defined by human context.
Researchers noted that datasets sourced from the internet frequently reflect societal biases. When these biases become embedded in trained AI models, they can reinforce unfair ideologies and behaviors.
Hence, understanding the impact of deduplication on bias prevalence enables mitigating adverse outcomes. For instance, it can prevent scenarios where an AI system defaults to showing images of only white men when asked to depict a CEO, doctor, or other roles, thereby failing to represent diversity as intended, according to the researchers.
That explains why FairDeDup is embedded with pruning to streamline datasets of image captions collected from the web. Pruning involves selecting a subset of data that represents the entire dataset. When done in a content-aware manner, it enables informed decisions about which data segments remain and which are discarded.
According to Slyman, FairDeDup eliminates redundant data while integrating controllable, human-defined dimensions of diversity to mitigate biases. This approach not only ensures cost-effective and accurate AI training but also promotes fairness.
Addressing Biases in AI Systems
Slyman emphasized that AI systems could uphold social justice by addressing biases during dataset pruning. This approach aims not to impose a singular notion of fairness on AI but to establish a framework to encourage fair behavior within specific contexts and user bases where AI is deployed.
Slyman pointed out that the team allows individuals to define fairness within their own settings rather than letting the internet or large-scale datasets dictate standards.
"By addressing biases during dataset pruning, we can create AI systems that are more socially just," Slyman said in a press release. "Our work doesn't force AI into following our own prescribed notion of fairness but rather creates a pathway to nudge AI to act fairly when contextualized within some settings and user bases in which it's deployed."
The research team's findings were presented at the IEEE/CVF Conference on Computer Vision and Pattern Recognition.
Related Article : Luma's AI Video Tool Dream Machine Under Scrutiny After Altered Version of Disney Character Appears in Its Trailer
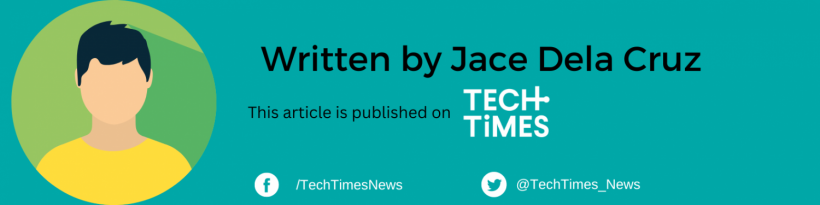