Scientists at Umeå University have successfully developed a self-driving forest machine controlled by AI, making it a significant feat in autonomous technology for heavy machinery.
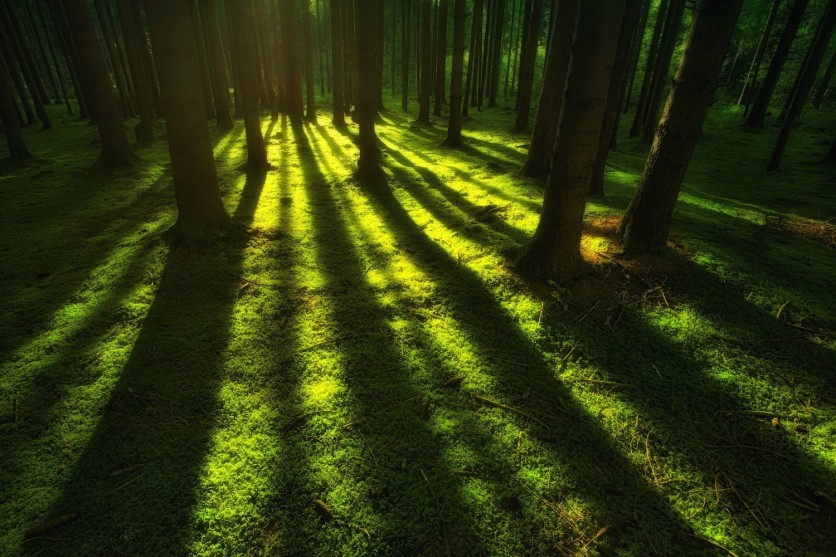
AI-Controlled Forest Machine
The breakthrough revolves around overcoming the complexities of transferring AI control to physical environments, particularly for large and intricate systems like heavy forest machinery.
Traditionally, AI requires extensive training data, which poses both financial and safety risks when applied to heavy equipment. The solution lies in preliminary training within simulated environments, although bridging the gap between simulation and reality remains a persistent challenge for researchers.
Trials at Umeå University's research facility and Skogforsk's testing ground in Jälla have demonstrated the feasibility of AI controlling a 16-ton forest machine autonomously.
This achievement rests on rigorous pre-training sessions conducted on Umeå University's supercomputers, comprising millions of training steps to prepare the AI for real-world tasks.
Viktor Wiberg, a researcher at Algoryx Simulation and Umeå University, underscores the significance of their work, affirming that autonomous control of such complex machinery through AI represents a first in the field.
The team leveraged "deep reinforcement learning," a method often used to manage intricate systems beyond human capabilities. However, the research team noted that its application has primarily been confined to digital systems or smaller robots until now.
Martin Servin, an associate professor of physics at Umeå University, highlights the inherent challenges in AI's adaptation to heavy machinery. Unlike lightweight robots with swift electric motors, forest machines operate with complex hydraulic systems and slow actuation.
This divergence necessitates tailored approaches in both simulation and real-world deployment to ensure seamless performance. The virtual training environments crucially mimic real-world dynamics, where AI models navigate diverse scenarios without physical risks or resource consumption.
This method enables rapid exploration of causal relationships between actions and outcomes, which is vital for refining AI capabilities in unpredictable environments.
Multibody Dynamics
Researchers emphasized the role of multibody dynamics and system identification in accurately simulating the forest machine's behavior. By integrating these techniques, they optimized AI policies to mitigate discrepancies between simulation and reality.
According to the researchers, techniques such as domain randomization, action delays, and penalties for erratic behavior contribute to narrowing the simulation-to-reality gap, which is crucial for reliable autonomous operations.
In their experiments, the AI-controlled forest machine demonstrated proficient performance on level ground and when navigating challenging terrains, such as ramps requiring active suspension management.
This successful transfer underscored the efficacy of their approach in aligning simulated behaviors with real-world applications, particularly in maintaining smooth motion trajectories and high-performance standards.
"The results show that it is possible to transfer AI control to a physical forest machine after first training it in a simulated environment," Wiberg noted.
The research team's findings were published in the journal Robotics and Autonomous Systems.
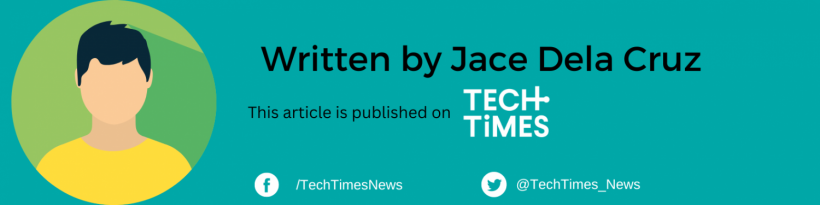