MIT researchers have introduced a new algorithm designed to facilitate the creation of shape-shifting slime robots using a machine learning technique.
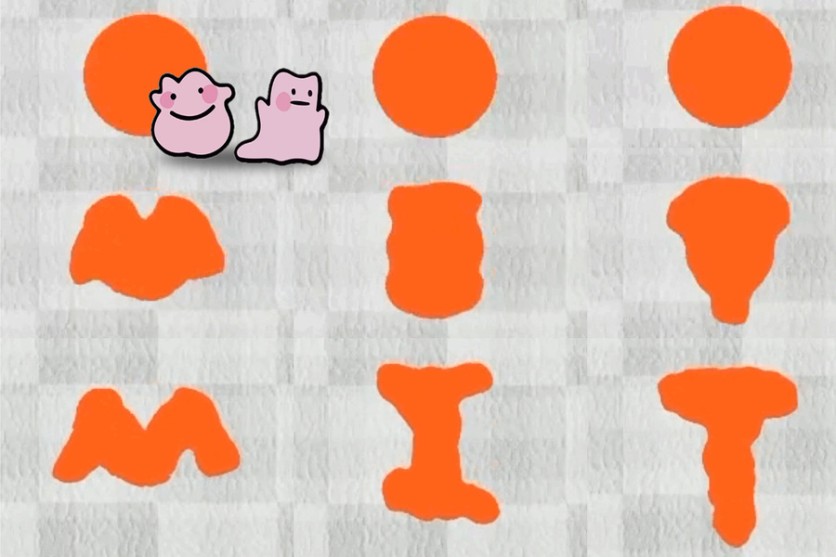
Shape-Shifting Slime Robots
Picture a robot capable of changing its shape on demand, squishing, bending, or stretching to perform various tasks like navigating tight spaces or retrieving objects.
While this may sound like something out of science fiction, MIT researchers are actively pursuing this concept by developing reconfigurable soft robots with potential applications in healthcare, wearable devices, and industrial settings.
The main challenge lies in controlling robots without traditional joints or limbs that can undergo drastic shape changes. MIT researchers have addressed this challenge by developing an advanced control algorithm capable of autonomously learning how to move, stretch, and shape a reconfigurable robot to accomplish specific tasks.
This algorithm enables the robot to morph multiple times during a task, adapting its form to suit different scenarios.
To evaluate the effectiveness of their approach, MIT researchers created a simulator to assess control algorithms for deformable soft robots across a series of complex, shape-changing tasks.
Boyuan Chen, an electrical engineering and computer science graduate student at MIT and co-author of the research paper, describes their robot as resembling "slime" due to its morphing capability.
Chen collaborated with lead author Suning Huang, an undergraduate student from Tsinghua University, along with Huazhe Xu and Vincent Sitzmann, who are also part of the MIT team behind this work. Their research is set to be presented at the International Conference on Learning Representations.
Read also: MIT Takes Strides Towards Greener AI: Addressing the Environmental Impact of Energy-Intensive Models
How MIT Tackled Shape-Shifting Robots
In the realm of robotics, traditional machine-learning methods like reinforcement learning are typically applied to robots with well-defined moving parts, such as grippers with articulated fingers. However, shape-shifting robots, which can deform dynamically, pose unique control challenges.
MIT researchers adopted a unique strategy to tackle these challenges. Instead of controlling individual muscle-like components, their reinforcement learning algorithm starts by controlling groups of adjacent muscles that collaborate.
This coarse-to-fine approach allows the algorithm to explore a wide range of actions before refining its strategy to optimize task completion.
Sitzmann explains that the algorithm treats the robot's action space as an image, employing machine learning to generate a 2D representation that encompasses the robot and its environment. This method exploits correlations between nearby action points, similar to the relationships between pixels in an image.
To evaluate their algorithm, MIT researchers developed a simulation environment called DittoGym, featuring tasks that assess a reconfigurable robot's ability to change shape dynamically.
While the practical application of shape-shifting robots may be years away, Chen and his colleagues are hopeful that their research will stimulate future advancements in reconfigurable soft robotics and complex control problems.
The findings of the research team were published in arXiv.
Related Article : PIGINet: MIT's New AI to Enhance Robots' Planning, Problem Solving, and MORE by as much as 80 Percent
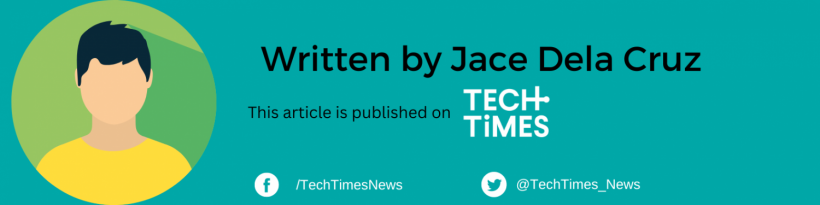