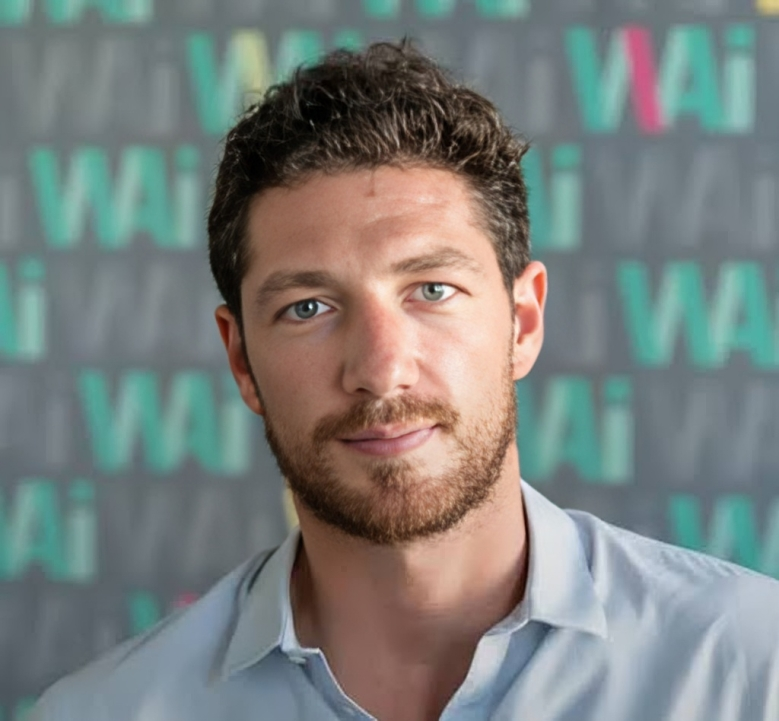
Semiconductor manufacturing, as it currently stands, faces a critical challenge: the lack of immediate, comprehensive feedback on the quality of its outputs. Traditionally, manufacturers have been limited to assessing only a small portion of their products, with test results delayed for hours or even days.
Lynceus.ai is pioneering a groundbreaking approach to semiconductor manufacturing, targeting the heart of its complexity: the real-time optimization of essential processes. In this interview with its founder, David Meyer, we're focusing on the impact and immediate benefits of AI implementation on production lines as well as regulatory peculiarities and common misconceptions about AI in the manufacturing industry.
What inspired you to integrate AI within the realm of semiconductor manufacturing? What have you achieved so far?
The concept of integrating AI into semiconductor manufacturing emerged from our desire to revolutionize high-value manufacturing processes. This aspiration led to the creation of Lynceus in 2020 alongside my co-founder, Guglielmo Montone, who brought extensive experience from a 15-year career in AI research. His expertise in developing methods for AI model training on fluctuating and inconsistent data, a prevalent issue in manufacturing, laid the groundwork for our venture.
At the heart of Lynceus was the ambition to fully automate the semiconductor manufacturing process. Our objective was to employ AI to oversee and optimize every phase of production, utilizing algorithms to continuously determine and apply the optimal machine settings for achieving superior product quality.
One of our most notable accomplishments to date is the successful deployment of our inaugural AI model in our clients' fabrication plants, marking our entry into the traditionally cautious semiconductor industry within just three years. The real hurdle was establishing our credibility, especially when engaging in discussions with professionals who hold PhDs and have dedicated two decades to mastering a single machine.
How do you market your product to manufacturing companies, and what outcomes do you assure?
Consider the dilemma faced by manufacturers aiming to enhance product quality through increased testing, which inversely affects production output. Our approach offers a solution to this predicament by refining the testing process to optimize efficiency. In factories committed to extensive testing, we've identified that up to 30% of processing steps are essentially non-value-adding, with about 70% of tested units proving to be satisfactory.
Our predictive model enables us to identify units that don't require testing, suggesting these can be bypassed. From our experience working with clients, we've managed to eliminate up to 70% of unnecessary measurements annually. This optimization significantly influences the following key areas.
Firstly, it reduces the cycle time-the duration it takes for a unit to complete the production process. By minimizing the number of units needing tests, we've successfully cut down the overall process time by approximately 10%, marking a substantial efficiency gain.
Secondly, it enhances the production capacity of the equipment. Many tested units are essentially control samples that don't contribute to sales. Reducing these tests frees up capacity for marketable units, leading to a 5 to 6% increase in tool capacity without the need for capital expenditure (CapEx) or additional labor.
Thirdly, for the testing tools themselves-not the processing equipment but the devices used for quality checks-we've optimized usage by about 25%. By forgoing certain tests, these tools are either utilized more effectively or less frequently, thereby reducing the CapEx required for these instruments. This is particularly significant given the substantial investment these tools represent and their footprint within the manufacturing site, which could otherwise be allocated to revenue-generating machinery.
This case study exemplifies the transformative impact our model can have on manufacturing operations, offering a glimpse into the potential efficiencies and savings achievable without the need for additional CapEx or workforce expansion.
What are some of the misconceptions you encounter about AI in manufacturing that you'd like to address?
This industry is extremely conservative. You have to understand that one factory costs between five and ten billion dollars of CapEx. Each machine is worth tens of millions of dollars. On average, almost everyone in the industry has been working for their company for more than ten years doing the same thing.
It's a very innovative industry, creating the most advanced chips that power smartphones and everything. But at the same time, it's really conservative because the financial stakes and the complexity of the process that they're doing is very high.
And so we came in saying that we can build a single software that will be able to deliver the same type of optimization across a wide variety of types of machines.
So even if you work on the same machine, there are hundreds of different types of products that are being run on one machine, depending on whether it's just after maintenance or just before it's gonna produce very different data. This seems impossible. They are saying that you cannot have a single model that's going to adapt to all those different situations.
It's also a closed industry where people have worked for a long time. If you don't come from semiconductor manufacturing, you're not going to be able to convince people on the production floor that this is working. It's something that I think we overturned.
When I meet with my customers' executives, they say that what makes us stand out is our experience deploying AI in semiconductor factories, which is our specialty. Even if you have people who have done semiconductor manufacturing for the past 30 years, deploying AI in a semiconductor factory is a completely different business. And even though we are only three and a half years old, I think we are the most experienced team out there in how to successfully implement artificial intelligence in this type of factory.
How do you view AI's impact on the manufacturing and chip-making industry in terms of overtaking people's jobs? Will it complement humans, or will it eliminate some work in some areas?
I think the most advanced tasks today are almost automated or close to being automated. Samsung announced recently that they want to automate their entire chip production by 2030. So it's not specifically AI; it's a type of industry where the main people who use our tool are called process engineers and actually define production processes. And they are constantly looking at ways to optimize how their machines are running and how to produce more at the best potential quality.
What we are giving them is a tool to manage their processes in real-time. When you think about how it works, usually, the unit comes into a machine, the machine processes it, then it goes outside of the machine, and it gets sent to another testing machine, which is somewhere else in the factory; the test happens, the result becomes available. And in the meantime, you don't stop the first machine. So you could have been producing for six to seven or eight hours in there. If the result of the test is not good, you might have to change some parameters on the machine, and it's extremely likely that you've been producing for six or eight hours with the wrong parametering of the machine.
And so those process engineers are, in a way, looking at the past, and what we enable them to do is look at data that was being produced right now and increase their reactivity. So it's more of a tool that augments what they're already doing rather than something that would disrupt them or take them out of the factory.
Are there any regulatory hurdles for AI startups in manufacturing?
The US is trying to prevent China from developing a chip industry and getting some specific types of technologies, which are sensitive, even though they're not American. Even at our level, we see interest from Chinese manufacturers and Chinese investment funds who try to gain access to this type of technology. So, rather than a regulatory thing, it's more of an impact of geopolitics on the strategic market.
Another thing is that a lot of those components are dual-use components, so they can be used to power a smartphone, but they can also be used to power some kind of military device. You have some of the products that we predict which are used in military applications as well. And especially if you start going towards very specialized semiconductor manufacturers, sometimes you need different levels of clearance to just be able to enter the facility.