A new AI tool harnessing human perception to refine audio quality has emerged as a breakthrough solution for real-world scenarios where clarity is paramount.
This deep learning model, developed by researchers from the Ohio State University with a keen focus on leveraging subjective assessments of sound quality, promises significant advancements in speech enhancement by minimizing unwanted background noise, a persistent challenge in audio processing.
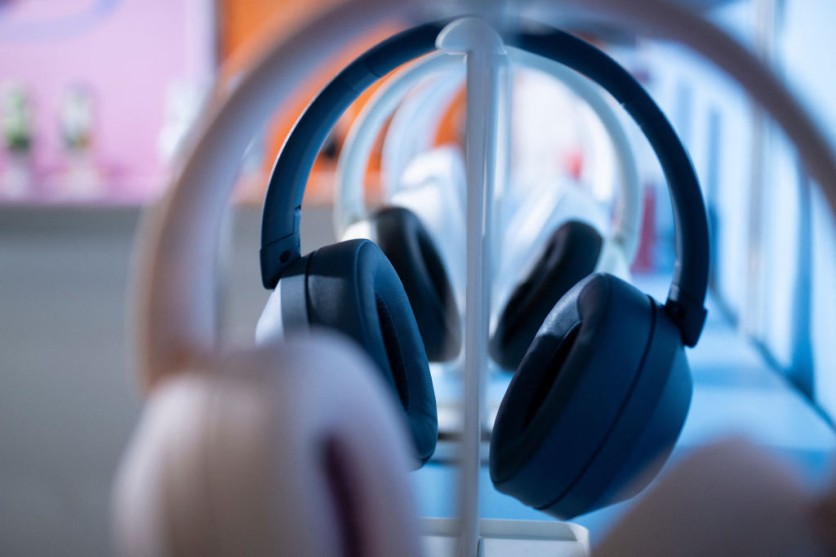
AI Uses Human Perception
Unlike conventional approaches that rely solely on objective algorithms, this innovative model integrates human perception into its training process.
By incorporating subjective judgments of sound quality, it gains valuable insights that contribute to its ability to eliminate noise effectively while preserving speech intelligibility.
Donald Williamson, co-author of the study and associate professor in computer science and engineering at the Ohio State University, underscores the significance of incorporating perception into the model's training process.
"What distinguishes this study from others is that we're trying to use perception to train the model to remove unwanted sounds. If something about the signal in terms of its quality can be perceived by people, then our model can use that as additional information to learn and better remove noise," Williamson said in a statement.
This research conducted training for the novel model using two datasets from prior studies, which contained audio recordings of individuals conversing. Some recordings included background disturbances such as television or music, potentially hindering clear communication. Participants evaluated the speech clarity of each recording using a rating scale ranging from 1 to 100.
Read Also : More Than 70% of Musicians Worried About the Impact of AI on Music Industry, New Study Finds
Collaborative Learning Technique
The performance of this team's model stems from a collaborative learning technique, merging a specialized speech enhancement language module with a predictive model capable of estimating the perceived quality score humans would assign to a noisy signal.
The findings indicated that their innovative approach surpassed alternative models, yielding superior speech quality according to objective metrics like perceptual quality, intelligibility, and human evaluations. However, Williamson noted that relying on human judgment for assessing sound quality presents inherent challenges.
He explained that the complexity of assessing noisy audio stems from its highly subjective nature, which hinges on individual hearing capabilities and past auditory encounters. Additionally, he noted that utilizing a hearing aid or cochlear implant contributes to altering the average person's perception of the sound environment.
Given the paramount importance of enhancing the quality of noisy speech for the advancement of hearing aids, speech recognition programs, speaker verification applications, and hands-free communication systems, the team noted that it becomes imperative to ensure that any disparities in perception are minimized to avoid making noisy audio less user-friendly.
The study, titled "Attention-Based Speech Enhancement Using Human Quality Perception Modeling," was published in the journal IEEE/ACM Transactions on Audio, Speech, and Language Processing.
Related Article : CD Projekt Red Wants to Use AI for Future Game Development-Will We See It for New Witcher, Cyberpunk Games?
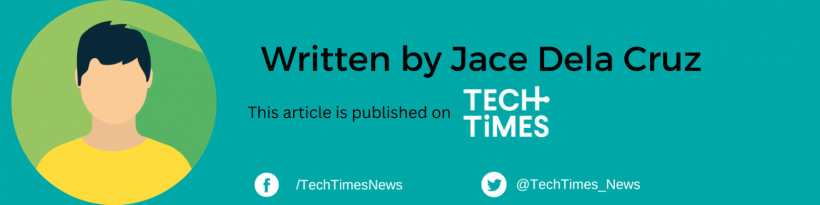
ⓒ 2025 TECHTIMES.com All rights reserved. Do not reproduce without permission.