Researchers have unveiled a new artificial intelligence (AI) model named "life2vec" that uses sequences of life events to predict various aspects of an individual's life, including death.
All About Life2vec
Developed using transformer models similar to those powering large language models like ChatGPT, life2vec was trained on a dataset sourced from the entire population of Denmark, comprising six million people.
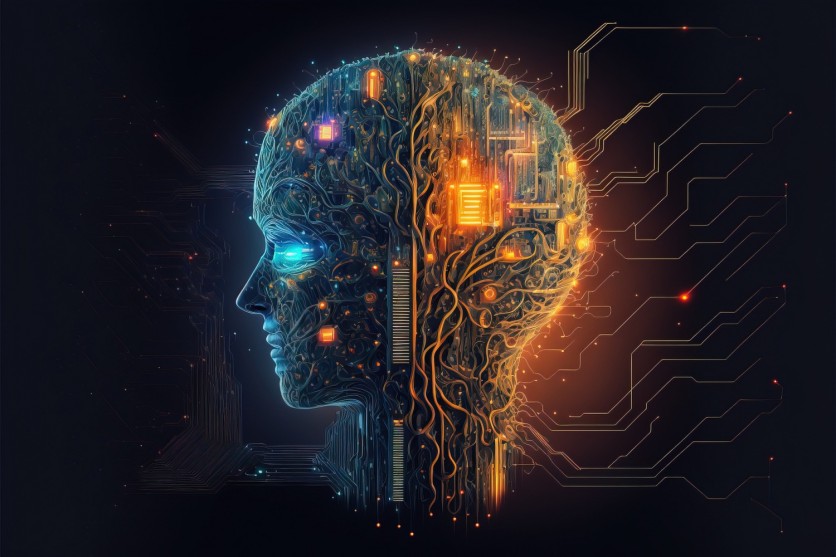
The tool demonstrated a capacity to predict future outcomes, including the lifespan of individuals, with a reported accuracy surpassing state-of-the-art models.
However, the research team emphasized that its primary utility lies as a foundation for future research rather than a tool for real-world predictions of individuals.
Tina Eliassi-Rad, Professor of Computer Science at Northeastern University, noted that while prediction is used to assess the model's effectiveness, it shouldn't be applied directly to real individuals.
She clarified that the tool is based on a specific dataset of a particular population and cautioned against its direct use for predicting outcomes for real people.
Eliassi-Rad, who contributed AI ethics expertise to the project, highlighted the broader societal implications of such tools, describing them as a means to "see into your society in a different way: the policies you have, the rules and regulations you have."
The tool essentially scans societal dynamics, shedding light on existing policies and regulations. To maintain a human-centered approach to AI development, the research team involved social scientists in the process.
Sune Lehmann, an author of the paper, emphasized that life2vec provides a more comprehensive reflection of human life, capturing the complexity of experiences and events that shape an individual.
The model relies on a massive dataset held by Statistics Denmark, offering a detailed registry of every Danish citizen's life events, encompassing health, education, and income.
The researchers transformed this wealth of data into long patterns of recurring life events, adapting the transformer model approach commonly used for training large language models.
Read Also : AI Study Shows Famous Raphael Painting Was Not Completely Created by the Italian Renaissance Master
Millions of Life Event Sequences
Life2vec creates vector representations in embedding spaces based on the information gleaned from millions of life event sequences. These embedding spaces serve as the foundation for the model's predictions, which extend to various aspects, including mortality probabilities and individual responses to personality questionnaires.
While the model's predictive capabilities are noteworthy, the researchers emphasize that these predictions are rooted in correlations specific to cultural and societal contexts and may carry biases inherent in the dataset.
Eliassi-Rad and Lehmann view life2vec not as a final product but as the beginning of a larger conversation. They envision their work fostering a more transparent understanding of predictive algorithms, enabling public discourse on their capabilities, applications, and ethical considerations.
"When we visualize the space that the model uses to make predictions, it looks like a long cylinder that takes you from low probability of death to high probability of death," Lehmann said in a statement.
"Then we can show that in the end where there's high probability of death, a lot of those people actually died, and in the end where there's low probability of dying, the causes of death are something that we couldn't predict, like car accidents," he added.
The team's findings were published in two papers in Nature Computational Science. You can access the first paper here and the second here.
Related Article : CaliExpress: World's First Fully Autonomous AI-Powered Restaurant Is Set to Open in Southern California
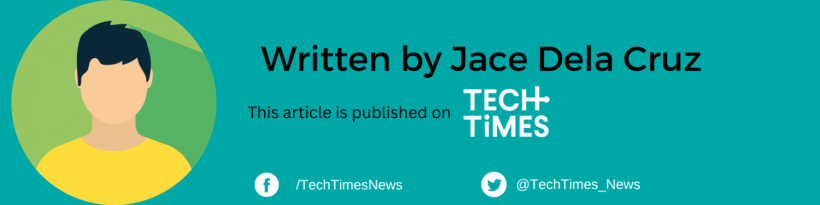