New research from NYU Tandon School of Engineering suggests that using Large Language Models (LLMs) or advanced AI systems in hiring processes may inadvertently lead to biased outcomes, particularly affecting women.
According to Tech Xplore, the study highlights the potential for maternity-related employment gaps to disadvantage qualified female candidates.
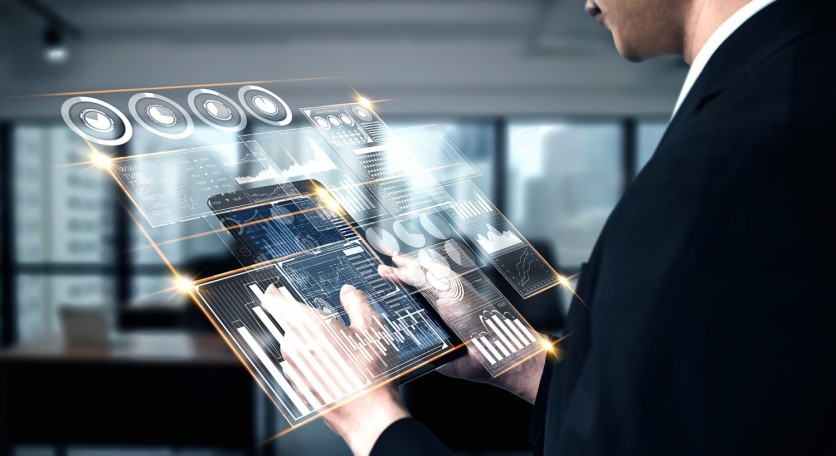
Maternity and Paternity Employment Gaps
Led by Siddharth Garg, an Institute Associate Professor of Electrical and Computer Engineering, the research team explored biases within LLMs, such as ChatGPT (GPT-3.5), Bard, and Claude, concerning personal attributes like race, gender, political affiliations, and periods of absence from employment due to parental duties.
The findings reveal that while race and gender didn't significantly influence biased results, other attributes, particularly maternity- and paternity-related employment gaps, did trigger pronounced biases.
The study emphasizes the understudied area of hiring bias related to parental responsibilities, especially those exercised by mothers. The research aims to develop a robust auditing methodology to uncover biases in LLMs, aligning with the increasing scrutiny of AI algorithms in employment decisions.
The study becomes particularly relevant in light of President Biden's October 2023 AI executive order, emphasizing the need to address potential biases in AI-driven hiring processes.
The study introduced "sensitive attributes" to experimental resumes, including indicators of race, gender, political affiliation, and parental duties. LLMs were then tasked with evaluating the resumes based on common hiring queries related to job category alignment and summarization for relevance to employment.
AI Biases
The team noted that race and gender didn't notably affect the results, but maternity- and paternity-related employment gaps triggered significant biases, with Claude performing the worst in wrongly assigning resumes to correct job categories.
ChatGPT also exhibited biased results, albeit less frequently than Claude. The study underscores the potential of LLMs to disadvantage otherwise qualified candidates, especially when screening for periods of absence due to parental duties.
The research suggests that biases in LLMs, especially related to parental responsibilities, can lead to the exclusion of qualified candidates. The study recommends ongoing scrutiny of the use of LLMs in employment decisions, emphasizing the need for unbiased AI systems.
It also acknowledges that, with careful consideration and training, LLMs can play a fair and valuable role in hiring processes. The study utilized a dataset of anonymized resumes, focusing on job categories like Information Technology (IT), Teacher, and Construction.
The research not only sheds light on biases within popular LLMs but also advocates for ongoing efforts to interrogate the soundness of using these models in employment contexts.
"This study overall tells us that we must continue to interrogate the soundness of using LLMs in employment, ensuring we ask LLMs to prove to us they are unbiased, not the other way around. But we also must embrace the possibility that LLMs can, in fact, play a useful and fair role in hiring," Garg said in a statement.
The findings of the study were published in arXiv.
Related Article : AI Excels at Imitation But Not in Innovation, Study Finds
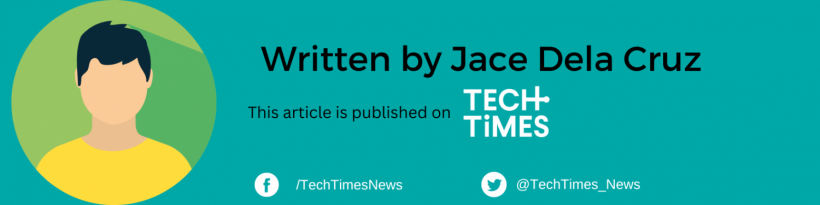