Researchers from the GrapheneX-UTS Human-centric Artificial Intelligence Centre at the University of Technology Sydney (UTS) have unveiled a revolutionary AI system capable of translating silent thoughts into text.
This endeavor, opening avenues to enhanced communication for those unable to speak due to stroke or paralysis, was led by distinguished Professor CT Lin, first author Yiqun Duan, and PhD candidate Jinzhou Zhou.
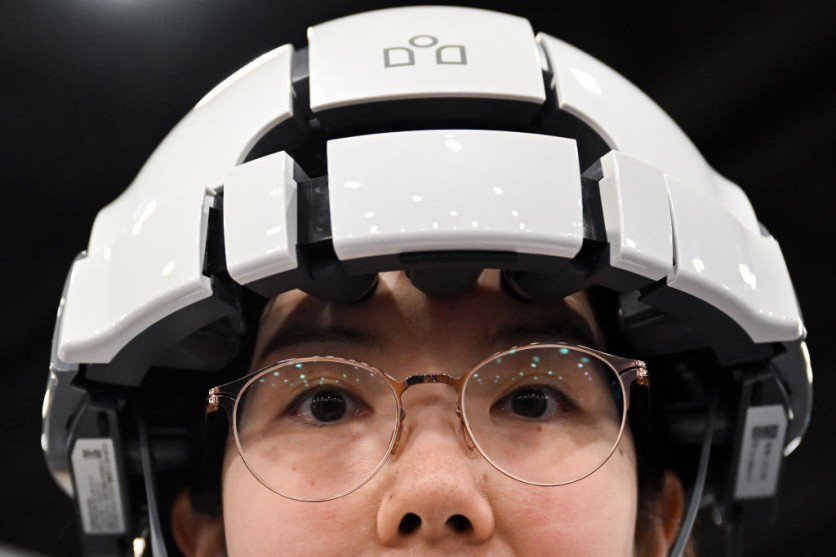
Translating Thoughts Into Texts
Participants in the study wore a cap recording electrical brain activity through an electroencephalogram (EEG) while silently reading passages.
The EEG data was then processed by an artificial intelligence (AI) model named DeWave, a creation of the researchers, which translated the brain signals into coherent words and sentences.
"This research represents a pioneering effort in translating raw EEG waves directly into language, marking a significant breakthrough in the field," said Lin.
"It is the first to incorporate discrete encoding techniques in the brain-to-text translation process, introducing an innovative approach to neural decoding. The integration with large language models is also opening new frontiers in neuroscience and AI," he added.
The developed system is portable and non-invasive, unlike previous technologies requiring invasive measures such as brain implants or large MRI machines.
It marks a departure from traditional methods by achieving word-level segmentation without additional aids like eye-tracking, making it more practical for everyday use.
The technology holds promise for individuals unable to speak due to various conditions like stroke, providing an alternative communication method. Additionally, it could facilitate seamless interaction between humans and machines, potentially aiding the operation of devices like bionic arms or robots.
The study, conducted with 29 participants, enhances the reliability and adaptability of the technology compared to earlier decoding methods, which were often tested on a limited number of individuals.
The EEG signals, which were collected with a cap instead of implanted electrodes, introduce some noise. However, according to the research team, the study indicates state-of-the-art performance in translating EEG, surpassing previous benchmarks.
Read Also : Nvidia Commits to Deepen Collaboration With Vietnam, Focusing On AI and Digital Infrastructure
What's Next for the Team
Although the model exhibits a higher proficiency in matching verbs over nouns, with a tendency towards synonymous pairs for nouns, the researchers acknowledge these challenges.
Currently, at 40% on BLEU-1, the translation accuracy score is a metric assessing machine-translated text similarity to high-quality reference translations. The team aspires to enhance this score to a level comparable to traditional language translation or speech recognition programs, which is near the 90% mark.
This groundbreaking technology builds on previous brain-computer interface advancements, such as brainwave-controlled quadruped robots developed in collaboration with the Australian Defence Force.
Integrating brain signals into meaningful text marks a remarkable stride toward a future where thoughts can be seamlessly translated into written language, revolutionizing communication for individuals facing speech impediments. The study's findings were published in Papers with Code.
Related Article : AI-Built Cancer Antibodies on the Horizon, Potentially Replacing Chemotherapy with AstraZeneca, Absci Collaboration
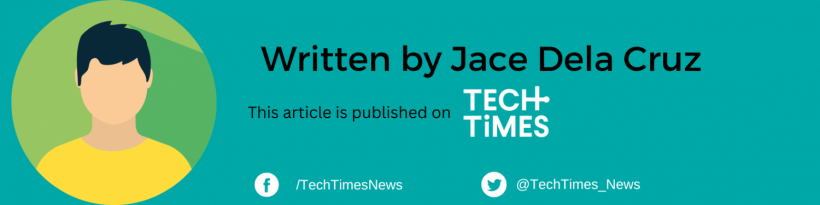