A recent study conducted by University of Michigan researchers has examined the bias in OpenAI's CLIP, a model integral to the functioning of the popular DALL-E image generator.
The findings suggest that AI image generation tools favor images that portray higher-income and Western lifestyles, showing the power to perpetuate societal inequities and cultural prejudices.
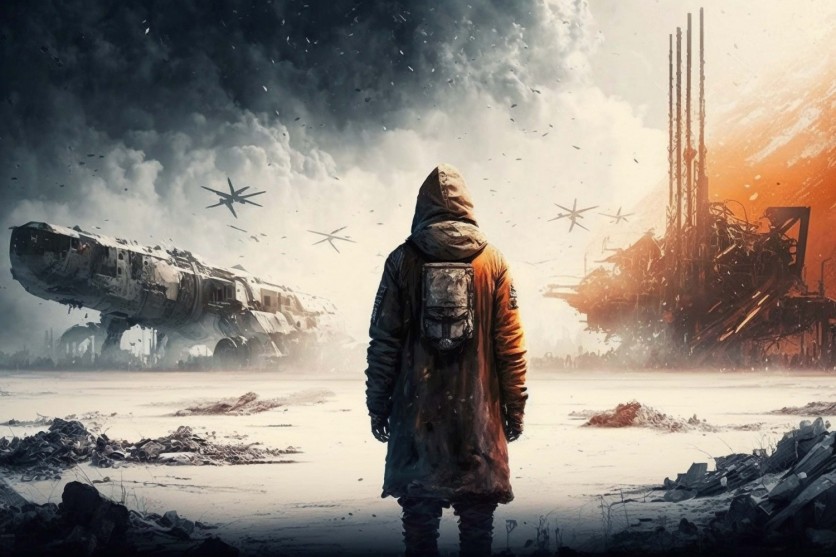
Evaluating CLIP
The study initiated and guided by Rada Mihalcea, the Janice M. Jenkins Collegiate Professor of Computer Science and Engineering, aimed to evaluate the performance of CLIP in handling images representing diverse socioeconomic backgrounds.
Mihalcea emphasized the importance of ensuring comprehensive representation in AI tools deployed globally to prevent the exacerbation of existing inequality gaps.
"During a time when AI tools are being deployed across the world, having everyone represented in these tools is critical. Yet, we see that a large fraction of the population is not reflected by these applications - not surprisingly, those from the lowest social incomes. This can quickly lead to even larger inequality gaps," said Rada Mihalcea, the Janice M. Jenkins Collegiate Professor of Computer Science and Engineering who initiated and advised the project.
Joan Nwatu, a doctoral student in computer science and engineering, led the research team alongside postdoctoral researcher Oana Ignat. CLIP is a model that combines text and images and generates a score indicating the perceived match between the provided text and an image.
This score is then used in downstream applications, such as image flagging and labeling. The researchers noted that OpenAI's DALL-E heavily relies on CLIP to evaluate performance and create a database of image captions.
The researchers evaluated CLIP's performance using Dollar Street, a globally diverse image dataset created by the Gapminder Foundation. This dataset includes over 38,000 images from households across Africa, the Americas, Asia, and Europe, with monthly incomes ranging from $26 to nearly $20,000.
The evaluation revealed a notable bias in CLIP's scoring, particularly favoring images from higher-income households. The correlation between CLIP scores and household income was evident, with images from wealthier families consistently receiving higher scores.
For instance, when assessing the topic "light source," CLIP scores were generally higher for electric lamps from wealthier households than kerosene lamps from lower-income families.
AI Geographic Bias
Geographic bias was also identified, as countries with lower scores were predominantly from low-income African regions. According to the study, this geographical bias raises concerns about the potential underrepresentation of diverse perspectives in large image datasets, particularly those relying on CLIP.
The researchers emphasized the need for AI developers to proactively address these biases and create more equitable AI models.
They proposed several steps, including investing in geographically diverse datasets, defining evaluation metrics that consider location and income, and transparently documenting the demographics of the data AI models are trained on.
Nwatu stressed the importance of transparency, stating that the public should be aware of the training data used in AI models to make informed decisions when utilizing these tools.
The study advocates bridging the performance gap across demographics to foster more inclusive and reliable AI models. The findings of the study were published in the arXiv.
Related Article : Bank of England to Assess the Risks AI Poses to UK Financial System
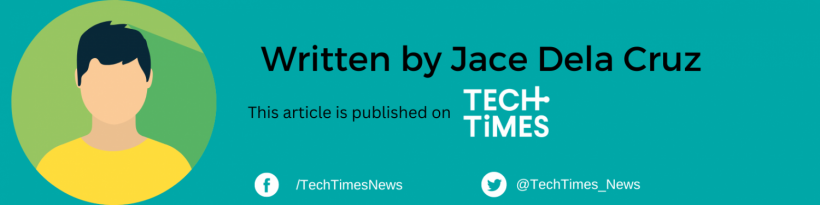