Scientists from the Technical University of Denmark (DTU), the University of Copenhagen, and Caltech have advanced the capabilities of artificially intelligent algorithms by adding a new dimension - taste. Wine apps like Vivino, Hello Vino, and Wine Searcher already employ AI algorithms to assist users in selecting wines based on labels and reviews.
Now, the researchers have demonstrated that integrating people's flavor impressions into these algorithms enhances their precision in predicting individual taste preferences, Tech Xplore reported.
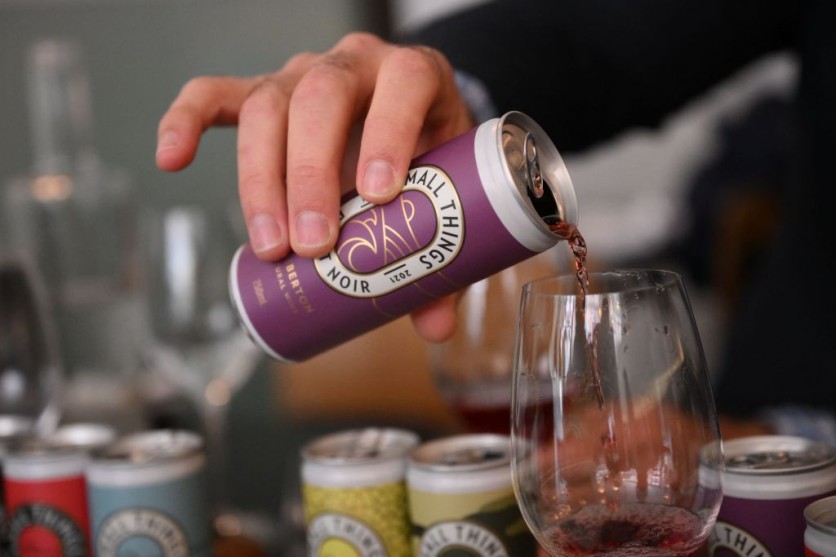
How An Algorithm Acquired 'Taste'
The study involved 256 participants in wine tastings, arranging shot-sized cups of different wines based on perceived similarities. The researchers digitized the tasting data, combining it with hundreds of thousands of wine labels and user reviews from Vivino.
Using this extensive dataset, an algorithm was developed to incorporate the dimension of flavor, enabling users to find wines similar in taste or both taste and price to their favorites.
Thoranna Bender, a graduate student at DTU, highlighted the algorithm's potential, saying, "I can stand with my favorite bottle of wine and say, I would like to know which wine is most similar to it in taste-or both in taste and price."
The study's co-author and professor Serge Belongie from the University of Copenhagen emphasized the significance of using human sensory experiences in machine learning, noting that it results in better algorithms for users. Incorporating taste as a data source in AI is novel and holds promise, particularly in the food sector.
Read Also : What Happens When AI Is Asked to Create a Bomb? Study Reveals LLMs' Susceptibility to 'Jailbreaks'
Food Science and AI
Belongie anticipated increased research at the intersection of food science and AI, emphasizing the importance of understanding taste for achieving healthy, sustainable food production.
While the use of AI in this context is in its early stages, the study showcases the potential of human-based inputs in enhancing AI capabilities. Bender suggested that the method can be applied to other types of food and beverages, such as beer and coffee.
The approach holds the potential for recommending products and food recipes and even tailoring meals for patients in the healthcare sector based on taste profiles.
The researchers have made their data publicly available for further exploration and development, encouraging collaboration and expanding the dataset for broader applications.
"We can see that when the algorithm combines the data from wine labels and reviews with the data from the wine tastings, it makes more accurate predictions of people's wine preferences than when it only uses the traditional types of data in the form of images and text. So, teaching machines to use human sensory experiences results in better algorithms that benefit the user," Belongie noted.
The findings of the research team were published in arXiv.
Related Article : Teachers, Students May Soon Use ChatGPT, OpenAI Explores Educational Applications for Classrooms
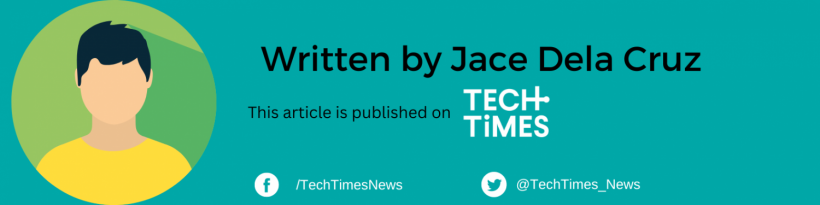