The production of perovskite solar cells, known for their efficiency in converting sunlight into electricity, is undergoing a transformation through the integration of artificial intelligence (AI) and machine learning.
Tandem solar cells utilizing perovskite semiconductors have demonstrated superior performance to traditional silicon solar cells. However, improvements in stability and manufacturing processes are essential to bring this technology to the market.
Researchers from the Karlsruhe Institute of Technology (KIT), in collaboration with Helmholtz Imaging at the German Cancer Research Center (DKFZ) and Helmholtz AI, have made significant progress in predicting and enhancing the quality of perovskite layers during the manufacturing process.
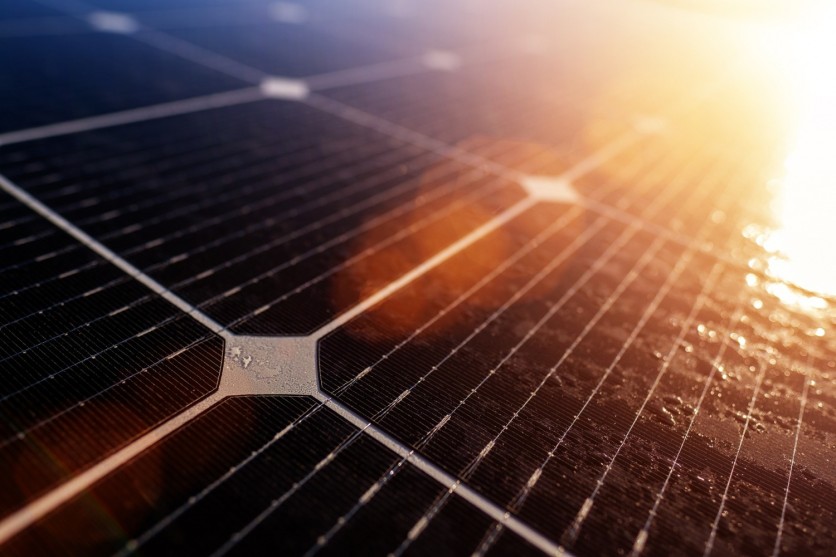
Next-Gen Solar Cell Tech
By harnessing the power of machine learning and innovative AI methods, they can assess the quality of these layers by analyzing variations in light emission during production.
The perovskite tandem solar cells combine a perovskite solar cell with a conventional silicon-based solar cell, marking them as a next-generation technology.
With an efficiency exceeding 33%, significantly higher than traditional silicon solar cells, and the use of cost-effective raw materials, these cells hold promise for widespread adoption, according to the researchers.
Manufacturing these advanced solar cells involves creating high-grade, multi-crystalline thin layers without deficiencies or holes using scalable and cost-effective methods. Overcoming this challenge is crucial for the industrial-scale production of highly efficient solar cells necessary for the ongoing energy transition.
A collaborative effort was initiated by KIT's perovskite solar cell experts and specialists in Machine Learning and Explainable Artificial Intelligence (XAI) from Helmholtz Imaging and Helmholtz AI to address the complexities of the manufacturing process.
The researchers employed AI methods to train and analyze neural networks using a vast dataset, including video recordings that captured the photoluminescence of thin perovskite layers during manufacturing.
Photoluminescence, the radiant emission of semiconductor layers excited by an external light source, provided critical insights that were not discernible to human experts.
The researchers pioneered using a machine learning AI system to detect hidden signs of coating quality from the extensive dataset, a departure from conventional approaches.
The researchers employed Explainable Artificial Intelligence methods to sift through the varied indications generated by the AI system, allowing them to gain insights into the factors influencing coating quality.
Paradigm Shift
The team experimentally observed variations in photoluminescence during production, highlighting its impact on coating quality. According to researchers, the innovative use of AI, particularly XAI, is a paradigm shift in materials science, offering systematic insights that go beyond traditional approaches.
By leveraging AI, the researchers gained a comprehensive understanding of the factors influencing the production of high-grade solar cells, enabling a more targeted and strategic approach to experiments.
The AI system, trained to recognize patterns in photoluminescence variations, proved instrumental in predicting the efficiency levels of each solar cell based on the observed light emission during the manufacturing process.
"These are extremely exciting results," said Ulrich W. Paetzold from the Institute of Microstructure Technology and the Light Engineering Institute at KIT.
"Thanks to the combined use of AI, we have a solid clue and know which parameters need to be changed in the first place to improve production. Now we are able to conduct our experiments in a more targeted way and are no longer forced to look blindfolded for the needle in a haystack. This is a blueprint for follow-up research that also applies to many other aspects of energy research and materials science," he added.
The findings of the study were published in the journal Advanced Materials.
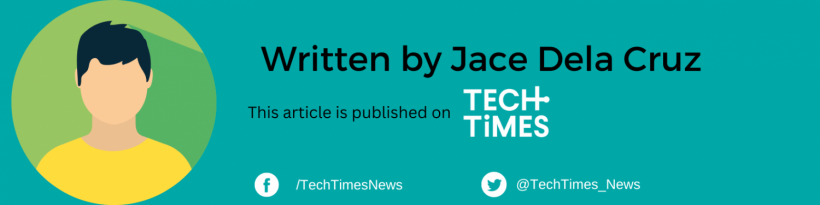