A collaborative work between Duke neuroscientists, neurosurgeons, and engineers has led to the development of a new speech prosthetic capable of translating brain signals into coherent speech.
This innovation holds potential for individuals facing communication challenges due to neurological disorders, offering a promising avenue via brain-computer interfaces.
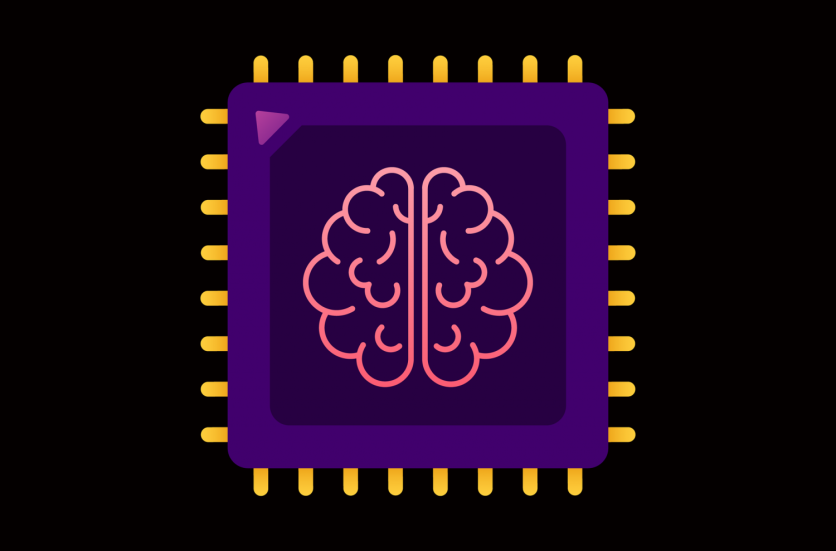
High-Density, Flexible Brain Sensors
Gregory Cogan, PhD, a neurology professor at Duke University's School of Medicine and a key figure in this venture, emphasized the importance of this technology for individuals dealing with conditions like ALS or locked-in syndrome.
He highlighted the current limitations of communication tools, which tend to be slow and unwieldy. Currently, speech decoding technology operates at a rate equivalent to half the speed of an audiobook, approximately 78 words per minute.
In contrast, the average human speech rate is around 150 words per minute. This lag stems partly from the limited number of brain activity sensors that can be integrated into an ultra-thin material placed on the brain's surface.
The scarcity of sensors results in less comprehensive information available for decoding, according to the researchers. To address this, Cogan collaborated with Jonathan Viventi, PhD, a fellow faculty member at the Duke Institute for Brain Sciences specializing in creating high-density, flexible brain sensors.
In this project, Viventi's team managed to incorporate an impressive 256 minuscule brain sensors onto a postage stamp-sized, medical-grade plastic substrate. This density of sensors allows for discernment of signals from neighboring brain cells, a crucial aspect for accurate predictions about intended speech.
The researchers then partnered with neurosurgeons from Duke University Hospital, including Derek Southwell, MD, PhD; Nandan Lad, MD, PhD; and Allan Friedman, MD, to conduct tests on four patients. These tests involved temporarily implanting the device in individuals undergoing brain surgery for other conditions.
Listen-and-Repeat Activity
Participants engaged in a listen-and-repeat activity, hearing and then speaking a series of nonsense words. The device recorded activity from the patients' speech motor cortex, which coordinates the movements of various muscles involved in speech production.
Subsequently, Suseendrakumar Duraivel, a biomedical engineering graduate student at Duke and lead author of the study, processed the neural and speech data using a machine learning algorithm. This algorithm aimed to predict the sounds produced based solely on the brain activity recordings.
Results demonstrated an 84% accuracy for certain sounds when they were the initial sound in a sequence of three forming a given nonsense word. However, accuracy diminished for sounds in the middle or end of a word, particularly when sounds were similar, such as /p/ and /b/.
In total, the decoder achieved 40% accuracy, an impressive feat considering it operated with only 90 seconds of spoken data from a 15-minute test. The team is currently pursuing a cordless version of the device with a $2.4 million grant from the National Institutes of Health.
While this technology shows significant potential, it is acknowledged that there is still progress to be made before it becomes widely accessible.
Viventi noted that the current speed of speech decoding remains slower than natural speech but envisions a trajectory toward improvement in the future. The findings of the study were published in the journal Nature Communications.
Related Article : New Study Delves Into World of Artificial Neural Networks, Unraveling How AI Brains Learn
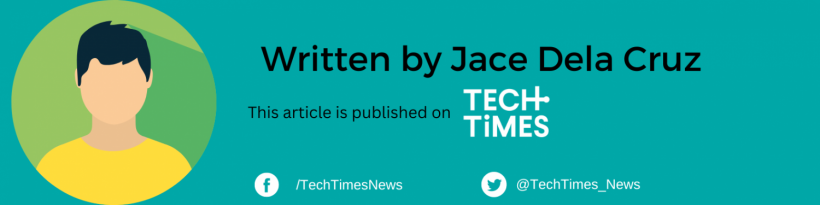