MIT's Computer Science and Artificial Intelligence Laboratory (CSAIL) has unveiled an innovative AI model that merges principles of diffusion and Poisson Flow.
According to MIT News, the researchers behind the work claim that the "Poisson Flow Generative Model ++" (PFGM++) surpasses existing models in image generation capabilities. This technology finds applications in various fields, including antibody and RNA sequence generation, audio production, and graph generation.
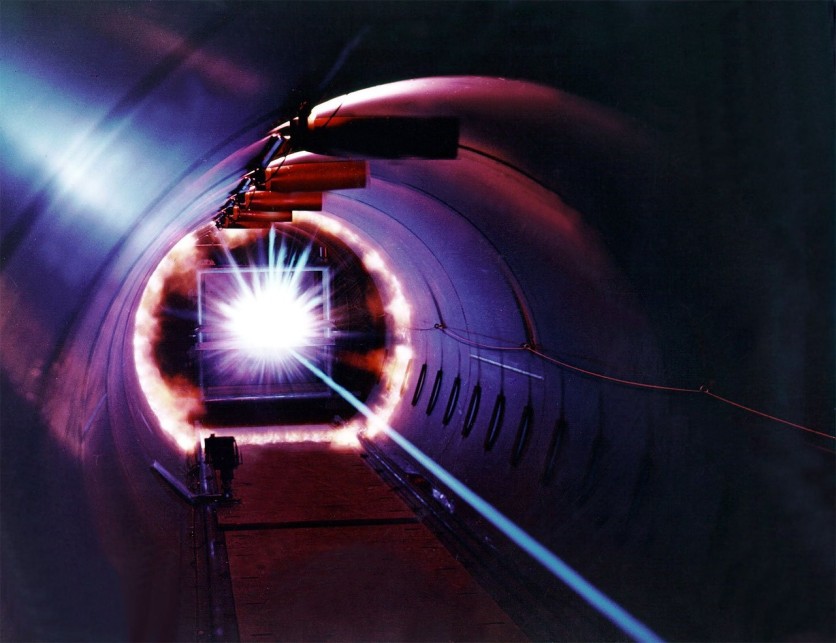
The Poisson Equation
PFGM++ builds upon the previous year's work on PFGM, drawing inspiration from the mathematical "Poisson" equation. The team added an extra dimension to the model, expanding its capabilities and allowing for a broader approach to data generation.
The Poisson equation is a fundamental partial differential equation in physics and mathematics. It describes how a physical quantity (such as electric potential, temperature, or gravitational potential) behaves in a region where the distribution of sources or charges is known.
In simple terms, it establishes a relationship between a field and the sources that generate it.
"Diffusion models have become a critical driving force behind the revolution in generative AI," Yang Song, a research scientist at OpenAI, said in a press statement.
"PFGM++ presents a powerful generalization of diffusion models, allowing users to generate higher-quality images by improving the robustness of image generation against perturbations and learning errors. Furthermore, PFGM++ uncovers a surprising connection between electrostatics and diffusion models, providing new theoretical insights into diffusion model research," he added.
The interdisciplinary collaboration between physicists and computer scientists has led to the development of PFGM++. It leverages century-old physics concepts, introducing extra dimensions of space-time for powerful synthetic dataset generation.
'Electric Field'
The underlying mechanism of PFGM involves envisioning data points as tiny electric charges on a flat plane within an expanded dimensionality. These charges create an "electric field" where they seek to move upwards into an extra dimension, forming a uniform distribution on an imaginary hemisphere.
Rewinding this process allows the model to learn and generate new data that mirrors the original. PFGM++ extends this electric field concept into a more intricate, higher-dimensional framework.
This balance between robustness and ease of use promises more efficient image and pattern generation. The team also introduced a new training method for enhanced learning of the electric field.
The model's performance was evaluated using the Frechet Inception Distance (FID) score, demonstrating higher resistance to errors and robustness. The researchers aim to refine the model for specific data, architectures, and tasks, optimizing its performance further.
"In recent years, AI-based generative models have yielded numerous eye-popping results, from photorealistic images to lucid streams of text," said Jesse Thaler, theoretical particle physicist in MIT's Laboratory for Nuclear Science's Center for Theoretical Physics, who was not involved in the study.
"Remarkably, some of the most powerful generative models are grounded in time-tested concepts from physics, such as symmetries and thermodynamics. PFGM++ takes a century-old idea from fundamental physics - that there might be extra dimensions of space-time - and turns it into a powerful and robust tool to generate synthetic but realistic datasets," Thaler added.
The findings of the team were published in arXiv.
Related Article : MIT, Microsoft Introduce 'Ekho' System to Sync Cloud-Based Games
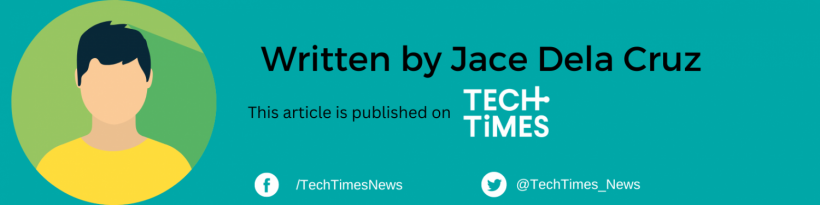