Money laundering remains a substantial global challenge, accounting for an estimated 2% to 5% of the annual global GDP, which is equivalent to a staggering £632 billion ($773 billion) to over £1.5 trillion ($1.8 trillion), according to the United Nations Office on Drugs and Crime (UNODC).
In response to this pervasive issue, computer scientists have developed a novel tool for swifter and more precise detection of money laundering. This tool boasts the impressive capability to scan 50 million transactions in less than one second.
The innovation is from researchers in the Department of Informatics from the King's College London, who have introduced a unique approach to identifying money laundering.
This new tool is based on advanced algorithms that quickly identify when criminals are dividing a large sum of money into multiple smaller transactions between numerous bank accounts, a technique commonly known as "smurfing."
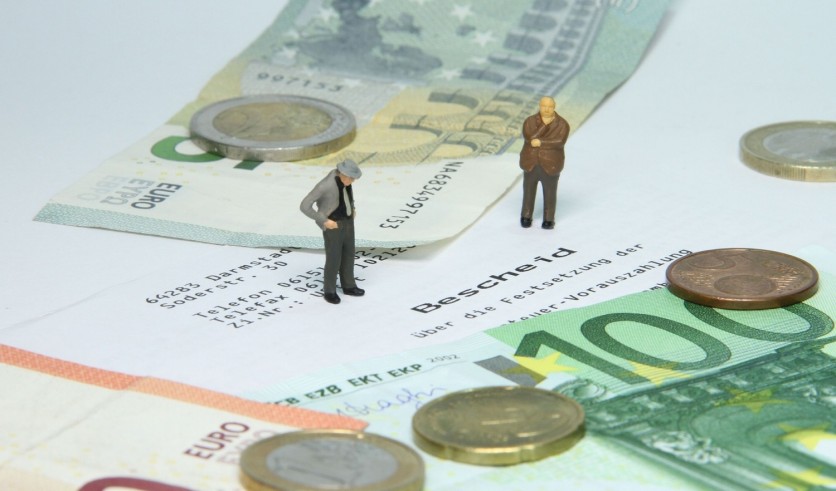
How the Tool Detects Money Laundering
The algorithms operate on data extracted from multiple bank accounts, represented as nodes in a complex graph. The software zeroes in on the segment of the graph exhibiting the most suspicious activity.
For instance, if there's a deposit of one million pounds, the software traces every instance of this exact sum, even if it's fragmented across various accounts and expenditures.
The tool's effectiveness significantly outpaces current detection methods, being over three times more efficient. According to the research team, it can also process larger volumes of data.
Existing money laundering detection methods are primarily rule-based, or machine-learning based, relying on preset criteria or analyzing extensive transaction histories for suspicious patterns. However, researchers noted that these methods often fall short in uncovering intricate schemes like smurfing.
Moreover, they necessitate prior knowledge of money laundering patterns, which can be lacking when dealing with emerging or evolving techniques. This limitation results in reduced accuracy.
Dr. Huiping Chen, lead researcher and PhD student at the Department of Informatics, underscored the critical importance of enhancing money laundering detection, recognizing it as a pressing global issue.
The Tool's Automation
Dr. Grigorios Loukides, co-lead researcher, emphasized the optimal efficiency of their method, surpassing current state-of-the-art approaches by 3.2 times. He highlighted the tool's automation and accelerated data analysis, empowering experts to swiftly identify suspicious actors.
"Our tool is also more automated and enables a far more rapid analysis of the data than what's currently available. By allowing money laundering experts to survey vast amounts of data faster than ever before, we can empower them to pick up on actors with bad intent efficiently," Loukides noted.
The researchers said the software for the new tool, being open source, is freely available to access. They noted that the tool can analyze huge amounts of data over long periods of time, filter out and sound an alarm to indicate to the bank when it detects potential illicit activity.
In testing, the team reported that the tool successfully identified all suspicious transaction patterns, using real data from a Czech bank and fictional cases based on projected common patterns and activity present in cases of real money laundering scenarios.
Beyond catching suspicious financial activity, the researchers claimed that the tool also holds promise in optimizing marketing campaigns by allowing retailers to detect the most profitable product bundles, improving the precision of retail data at speed.
The team's findings were published in the Proceedings of the 2023 SIAM International Conference on Data Mining.
Related Article : India Investigating Several Crypto Cases for Money-laundering Schemes
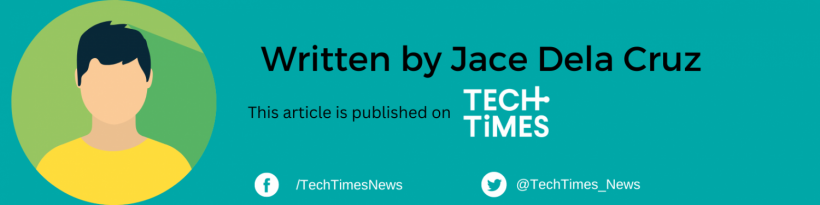