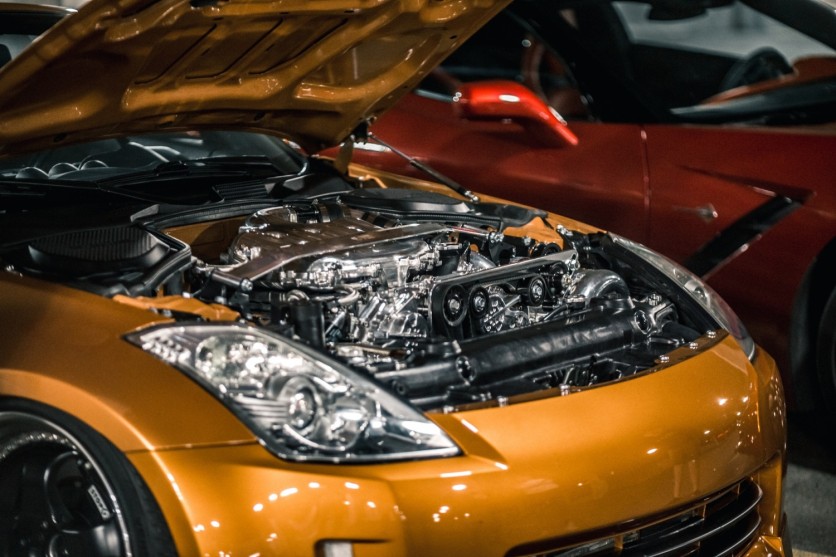
"Machine learning algorithms enable vehicles to 'learn' from real-world conditions and evolve," says Dr. Rohit Ugle, a visionary Technical Specialist who champions new technological tools to improve the strides of passenger and off-road vehicles.
As per recent reports by Gitnux Market Data, the total revenue of the US automotive repair and maintenance market reached an impressive $146.2 billion. Projections indicate that the global automotive repair and maintenance services market could skyrocket to $810 billion by 2026. This staggering figure underscores the substantial number of vehicles necessitating repair and maintenance services and the astronomical financial investment devoted to these services. The automotive repair and maintenance industry significantly impacts the US economy.
How can technological advancements effectively address the surging global demand? For Dr. Ugle, the answer lies in machine learning. By employing methodologies grounded in data-driven insights, predictive maintenance solutions, and condition-monitoring technologies, Dr. Ugle thinks the entire industry around vehicle maintenance can be reshaped to facilitate preemptive interventions and yield efficiency gains in terms of time, resources, and cost.
Dr. Ugle had designed a novel methodology to reduce repair costs and increase customer satisfaction. The current maintenance process is outdated and a significant source of customer frustration. Also, the lack of trained technicians on modern vehicle changes makes it difficult for the technicians to address the problem quickly when the vehicle is in the maintenance bay.
The Landscape of Modern Maintenance
Imagine a world where vehicles possess the foresight to signal their imminent issues, giving mechanics the time and insight needed for precision repairs. According to Dr. Ugle, this scenario is not just a sci-fi dream but a realistic outcome through machine learning.
One of the primary abilities of using machine learning is access to predictive maintenance. Traditionally, vehicles followed standardized servicing schedules, often leading to components being overused or replaced prematurely. Machine learning, meanwhile, offers a shift to this approach. Algorithms can accurately predict when a specific component is on the brink of faltering by employing sensors that capture data on many parameters-engine performance, wear on critical parts, and environmental conditions.
Dr. Ugle explains that machine learning models analyze data from each vehicle, identifying wear patterns unique to individual components. As an anomaly arises, these tools can trigger an alert where mechanics receive advance notice, proactively enabling them to replace the worn part. This measure avoids the impending breakdown and the cascading effect it might have had on delivery schedules, customer satisfaction, and overall costs.
Dr. Ugle's contributions have an impact on current maintenance procedures. The next-generation processes identify fault signatures from real-time driving signals like vehicle speed, engine temperature, tire pressure, etc., and alert the drivers about the possible failure of vehicle components. The data-driven technology has far-reaching effects on minimizing warranty costs for the OEMs, motivating technology deployment, and creating a win-win situation for the dealers, OEMs, and customers.
"No more unnecessary downtime due to premature replacements and catastrophic breakdowns resulting from overlooked wear. The implications are profound," Dr. Ugle explains.
Optimal Fuel Management and Efficiency
As the world embraces sustainability and energy efficiency, machine learning steps in to optimize fuel management, one of the critical components of vehicle maintenance. According to Dr. Ugle, traditional fuel management systems rely on predetermined settings that may not account for real-time driving conditions. Machine learning can adapt fuel consumption strategies based on traffic congestion, road gradients, and driving patterns.
Consider a public transportation network aiming to reduce fuel costs and environmental impact. With machine learning models, it can formulate efficient routes, adjusting for traffic conditions and passenger load by analyzing historical and real-time data. This ability minimizes fuel consumption and enhances operational efficiency, translating into cost savings for the operator and reduced emissions for the environment.
Dr. Ugle's research in optimizing the performance of electric vehicle batteries is a classic example of where modern data pipelines can improve efficiency and optimize available energy for electric vehicle users. The machine learning model takes dynamic data points like energy prices for the next day, typical usage of the vehicle every day, battery health, charge time, peak loads on the grid, etc., and proposes an optimized usage cycle that recommends a charging strategy route planning for the user.
Ability to Launch Personalized Maintenance
Vehicles have unique characteristics and usage patterns that influence their maintenance requirements. Machine learning can tailor maintenance schedules and recommendations to individual and diverse fleets of vehicles, including sedans, hybrids, and electric cars, optimizing resources and ensuring each vehicle receives precisely what it needs.
Machine learning algorithms can analyze usage data, driving patterns, and vehicle specifications for a ride-sharing platform operating different vehicles to develop tailored maintenance plans. This option guarantees efficiently allocated maintenance efforts where technicians can provide the proper requirements, saving effort and time while minimizing operational costs.
Dr. Ugle has developed and deployed "Health Detective.ai," which monitors real-time vehicle diagnostic codes. This virtual product analyzed the patterns of the diagnostic codes. It was an ensemble of several machine learning models built, e.g., Xgboost and Linear-SVM, to alert the user of future failure and its timeline. Not only is the customer alerted about the possible anomaly, but the dealer and technicians are also recommended with possible fixes to the predicted loss. This feature significantly increases the technician's efficiency in identifying and performing correct service procedures. The machine learning models helped customers resolve their problems on their first dealership visit.
Perspective to Ponder
While the integration of machine learning into vehicle maintenance has proven efficiency and predictability, some may argue the importance of critically assessing the ethical dimensions of this advancement. The reliance on algorithms to determine maintenance schedules and predict component failures raises concerns about data privacy, ownership, and potential algorithm biases.
Such dangers call for the importance of ethical considerations. Although technology carries benefits, it is a human partner tool, not a substitute. The goal is not to replace mechanics but to empower them with new tools.
These situations also spotlight the need for mechanics to evolve into technicians adept at interpreting algorithmic insights. Dr. Ugle's knowledgeable algorithm recommendations in the form of predictive alerts are valuable inputs to inform their assessments rather than the sole determinant of maintenance actions. This ability fosters a symbiotic relationship between data-driven insights and skilled craftsmanship.
Rohit Ugle's Pivotal Role
As the automotive industry inches toward data-driven insights, technical specialists like Dr. Ugle are architects of innovation. As he fuses machine learning and physics-based principles, his designs aim to "optimize vehicle components' utility and minimize the cost of ownership to the customers."
With Dr. Ugle's educational background, a bachelor's in Mechanical Engineering with a Ph.D. in Engineering, numerous vehicle maintenance research citations, and data science skills earned through practical application of solving real-life vehicle maintenance problems, he created data pipelines that efficiently harness massive influx of data. He also creates intuitive visualizations that allow end-users-mechanics, fleet operators, or drivers-to understand the health of their vehicles at a glance with clarity, saving time and effort while improving results.
Dr. Ugle's initiatives and expertise mirror the growing industry trend: a shift from reactive maintenance to proactive, cost-efficient solutions that ensure smooth vehicle operation and streamline expenses. With the leading technical specialist at the forefront of machine learning integration, creating end-to-end solutions for every stage of vehicle maintenance sees a brighter and limitless future.