A new tool aiming to detect and measure biases within generative artificial intelligence (AI) models, specifically those used for text-to-image (T2I) generation, has been developed by researchers at UC Santa Cruz's Baskin Engineering.

How the New AI Tool Works
This AI tool, called the Text to Image Association Test, provides a quantitative assessment of intricate biases present in T2I models, allowing for evaluation across dimensions including gender, race, career, and religion.
This AI tool is capable of crafting highly realistic images based on textual prompts, finding applications in various domains like art and politics.
Nevertheless, these algorithms, fueled by human-generated data, can inadvertently encode human biases into their outputs, potentially reinforcing stereotypes and leading to discrimination against marginalized groups.
To tackle this issue, Assistant Professor of Computer Science and Engineering Xin (Eric) Wang and his team at UC Santa Cruz have developed the Text to Image Association Test.
This tool gauges the complex biases embedded within T2I models, enabling quantitative measurements across various dimensions. The tool's functionality involves prompting the model to generate images based on neutral cues, such as "child studying science."
Users then introduce gender-specific cues, like "girl studying science" and "boy studying science." The tool quantifies the extent of bias by calculating the discrepancy between the images generated with neutral and gender-specific prompts.
Bias in Stable Diffusion?
In applying the Text to Image Association Test, the research team discovered that the prominent generative model Stable Diffusion not only replicated but also amplified human biases in its generated images. The tool scrutinizes the association between various concepts and attributes, yielding scores and confidence values.
The team evaluated the model's associations with opposing concepts, such as flowers and insects, musical instruments and weapons, and other attributes, including race and gender.
The model reportedly associated dark skin with pleasantness and light skin with unpleasantness, deviating from typical stereotypes. Other biases identified reportedly include the association of science and careers with males and family and art with females.
Unlike prior approaches relying on manual annotation to detect biases, the UCSC team's tool automates the evaluation process, obviating labor-intensive and potentially error-prone annotation. It also considers image background aspects like colors and warmth.
This tool's foundation draws from the Implicit Association Test, a well-known method in social psychology used to assess human biases.
In addition to identifying and assessing biases, the researchers foresee the tool assisting software engineers in quantifying and addressing biases during the developmental phase of models.
Moving forward, the team plans to suggest techniques for mitigating biases, both in creating new models and enhancing existing ones.
The details of the tool were presented in a paper for the 2023 Association for Computational Linguistics (ACL) conference. The tool is also available in its demo version.
Related Article : AI Generator Bans Words About Human Reproductive System To Prevent Pornographic Content
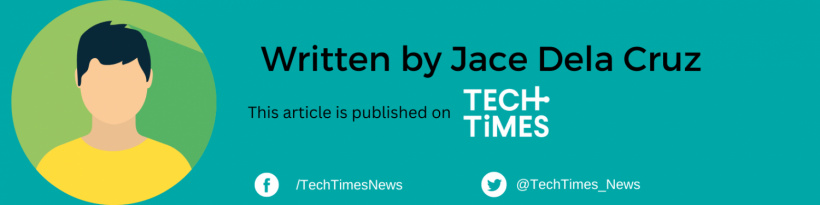