MIT researchers have developed a groundbreaking approach to enhancing the quality of AI-generated captions for online charts.
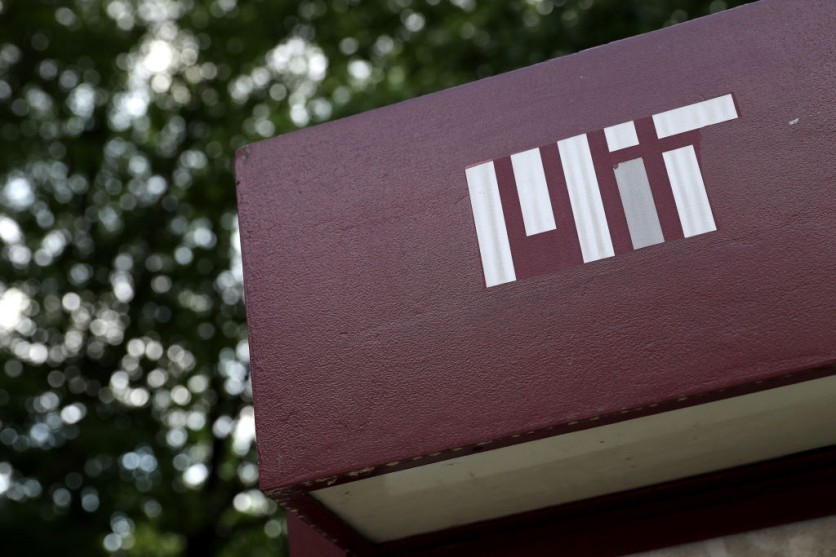
VisText
Chart captions play a crucial role in helping readers comprehend complex data patterns and are especially vital for individuals with visual impairments who rely on captions for information.
Creating informative chart captions can be a time-consuming process. While auto-captioning techniques have been developed to alleviate this burden, they often struggle to provide contextual information and describe intricate data features accurately.
To address these challenges, a team of MIT researchers has introduced a dataset called VisText. This dataset aims to improve automatic captioning systems for charts by training machine-learning models to generate captions of varying complexity and content based on user requirements.
The researchers observed that machine-learning models trained on the VisText dataset consistently produced precise, semantically rich captions that effectively described data trends and complex patterns.
Their models outperformed other auto-captioning systems, as validated through quantitative and qualitative analyses.
The MIT team intends to make the VisText dataset available as a valuable resource for researchers working on chart auto-captioning. By leveraging these automated systems, uncaptioned online charts can be equipped with informative captions, thereby enhancing accessibility for individuals with visual disabilities.
Human-centered Analysis into Autocaptioning
The inspiration for developing the VisText dataset stemmed from prior work conducted by MIT's Visualization Group. In a previous study, the group discovered that sighted users and visually impaired users have varying preferences regarding the complexity of semantic content in captions.
To integrate this human-centered analysis into auto-captioning research, the researchers created the VisText dataset, consisting of charts and associated captions. This dataset serves as training data for machine-learning models to come up with accurate, semantically rich, and customizable captions.
Autocaptioning systems face challenges. Current approaches treat charts like images, ignoring the distinct interpretation of visual content in charts. Some methods rely only on data tables, which may not be available later.
To address this, the VisText dataset represents charts as scene graphs, including chart data and image context. Scene graphs capture most image information and work well with language models.
The dataset has 12,000+ charts as tables, images, and scene graphs with captions. Low-level captions describe chart construction, while high-level captions provide statistics and trends.
Low-level captions are auto-generated, and high-level captions come from crowdsourcing. Captions follow accessibility guidelines and include important chart details for visually impaired readers.
The MIT team's next steps involve further refining the models to reduce common errors and expanding the VisText dataset to include more complex charts. Additionally, they aim to gain insights into what these auto-captioning models learn about chart data.
Related Article : Are Talking Spaceships Coming Soon? NASA to Revolutionize Space Exploration with AI-Powered ChatGPT-Like Interface
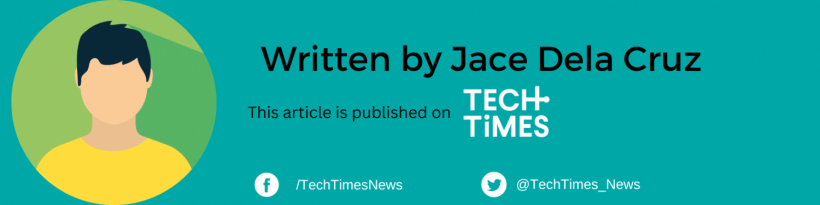
ⓒ 2025 TECHTIMES.com All rights reserved. Do not reproduce without permission.