MIT and IBM have collaborated to develop a new tool aimed at assisting users in selecting the appropriate artificial intelligence (AI) method, according to MIT News.
In real-world scenarios where machine-learning models are utilized, it is crucial for human users to determine when to trust the predictions made by these models.
However, due to the complexity of these models, even their creators often lack a comprehensive understanding of how they generate predictions. To address this challenge, researchers have developed saliency methods that aim to explain the behavior of these models.
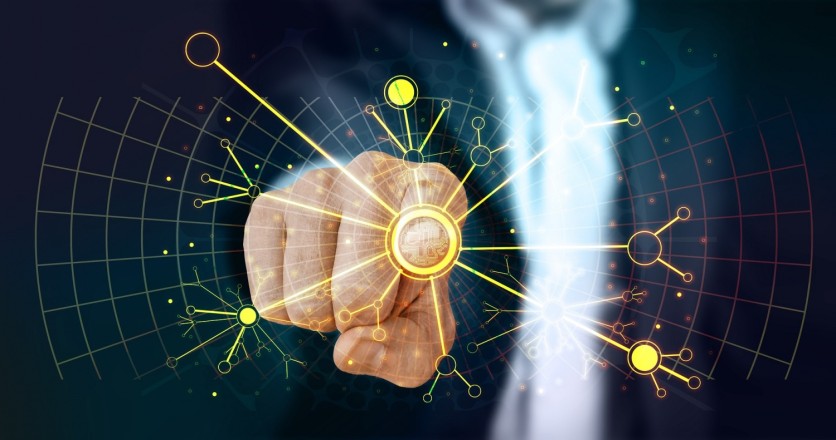
Saliency Cards
Recognizing that new saliency methods are continually being introduced, researchers from MIT and IBM Research have devised a tool to aid users in choosing the most suitable saliency method for their specific tasks.
They have created saliency cards, which offer standardized documentation of how a method operates, containing information about its strengths, weaknesses, and explanations to facilitate accurate interpretation.
Co-lead author Angie Boggust, a graduate student in electrical engineering and computer science at MIT, explains that the intention is to empower users to deliberately select the appropriate saliency method based on the type of machine-learning model they are utilizing and the task it is performing.
The researchers conducted interviews with AI experts and professionals from various fields, revealing that the saliency cards facilitate a quick comparison of different methods, enabling users to choose the most suitable technique for their task.
By selecting the right method, users gain a more accurate understanding of their model's behavior and can correctly interpret its predictions.
Boggust emphasizes that the saliency cards are designed to provide a concise summary of a saliency method, highlighting its most critical attributes in a user-centric manner.
The cards cater to a wide range of users, including machine-learning researchers and individuals seeking to understand and select a saliency method for the first time.
Sparking Further Investigation
In addition to facilitating method selection, the saliency cards serve as a means to identify gaps in research. The researchers discovered that they were unable to identify a saliency method that was both computationally efficient and universally applicable to any machine-learning model.
This finding has sparked further investigation to explore whether such a method exists or if there is an inherent conflict between these two requirements.
The team conducted a user study involving eight domain experts, including computer scientists and a radiologist unfamiliar with machine learning. The participants found the concise descriptions provided by the saliency cards to be valuable in prioritizing attributes and comparing methods.
Notably, even the radiologist, despite lacking familiarity with machine learning, was able to comprehend the cards and utilize them to participate in the method selection process.
Looking ahead, the researchers aim to investigate attributes that have received less evaluation and potentially create saliency methods specifically tailored to certain tasks.
Moreover, they seek to enhance their comprehension of how individuals perceive the outputs of saliency methods, which could result in improved visual representations.
To encourage collaboration and receive valuable input, the researchers are making their work accessible on a public repository.
Boggust envisions the saliency cards as living documents that will evolve alongside the development of new saliency methods and evaluations. Ultimately, this initiative marks the beginning of a broader conversation on the attributes of saliency methods and their relevance to different tasks.
Related Article : AI Expert Says Deepfakes Harder to Spot Than Ever Before
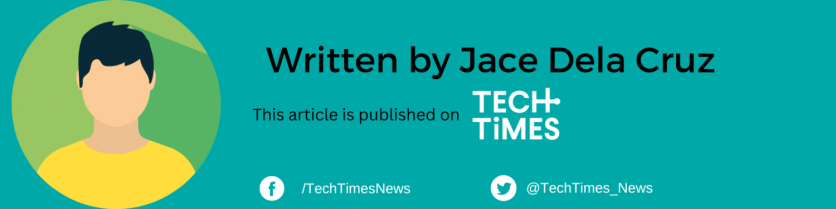