AI cheating detectors, touted as a solution to identify content written by AI, may not be as effective as previously believed, according to a recent study conducted by Stanford scholars.
These detectors, designed to flag cheating, plagiarism, and misinformation, are proving to be particularly unreliable when it comes to non-native English speakers, as per the study.
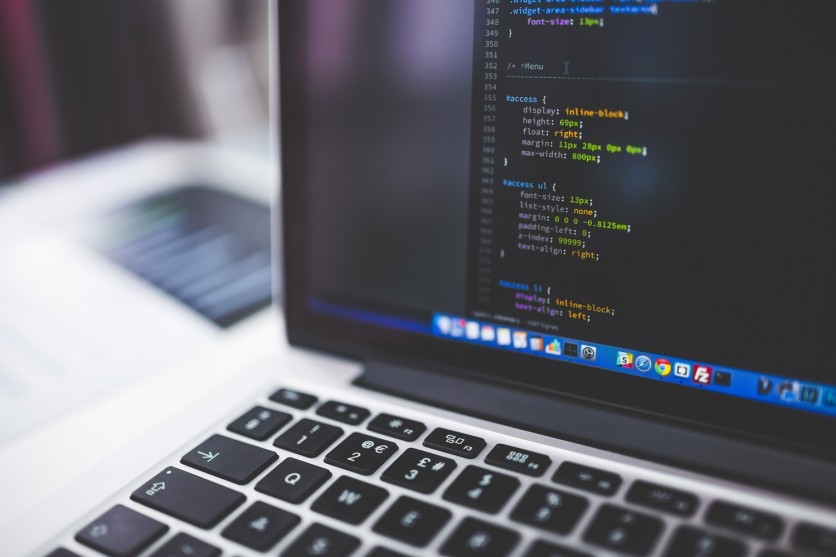
AI on Educational Institutions
AI cheating detectors are on the rise due to the increasing prevalence of AI-generated content and concerns about academic integrity.
The demand for these detectors cannot be overstated with the rise of AI chatbots such as OpenAI's ChatGPT and Google's Bard. In fact, plagiarism detection service Turnitin can now detect whether AI generated a paper or any content.
Educational institutions, in particular, are keen to leverage AI technology to streamline the process of identifying plagiarized or AI-generated content, saving time and resources compared to manual detection methods.
This has particularly prompted Palm Beach State College, which recently implemented AI and cheating detection schools. But the new study now raises the accuracy and reliability of AI detection tools.
Alarming Statistics
The study reveals alarming statistics. While the detectors performed well in evaluating essays by American eighth-graders, they misclassified over 60% of TOEFL (Test of English as a Foreign Language) essays written by non-native English students as AI-generated. Remarkably, all seven AI detectors unanimously labeled 19% of the TOEFL essays and flagged 97% of them as AI-generated.
Professor James Zou, the senior author of the study, explains that the detectors rely on a metric called "perplexity," which assesses the complexity of writing and correlates with its sophistication.
Naturally, non-native speakers tend to score lower on perplexity measures such as lexical richness, diversity, and syntactic and grammatical complexity, according to the researchers.
The study raises significant concerns about the objectivity of AI detectors and the potential for unjust accusations or penalties for foreign-born students and workers. Zou highlights the ethical implications and cautions against overreliance on these AI detectors.
Moreover, Zou points out that the detectors can be easily bypassed through a technique known as "prompt engineering." Students can exploit the detectors' vulnerabilities by requesting generative AI to "rewrite" essays with a more sophisticated language.
To address this issue, Zou proposes several suggestions. In the short term, he advises against relying on detectors, particularly in educational settings with a significant number of non-native English speakers.
In addition to perplexity, developers are urged to explore alternative metrics and consider incorporating watermarks into the content generated by AI.
These watermarks would include subtle hints about the AI's identity. Furthermore, efforts should be focused on improving the models' ability to withstand circumvention.
Given the supposed unreliability of current AI cheating detectors and the potential consequences for students, Zou emphasizes the need for rigorous evaluation, significant refinements, and a cautious approach before fully embracing these technologies.
"The detectors are just too unreliable at this time, and the stakes are too high for the students, to put our faith in these technologies without rigorous evaluation and significant refinements," Zou said in a press release statement.
The study, titled "GPT detectors are biased against non-native English writers," was published in arXiv.
Related Article : How To Use ChatGPT for Trip Plans? New Survey Says 1/3 of US Travelers Already Doing It!
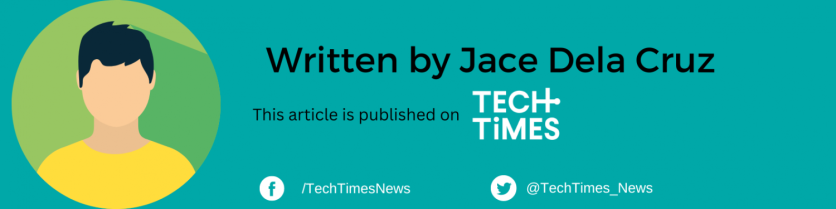
ⓒ 2025 TECHTIMES.com All rights reserved. Do not reproduce without permission.