OpenAI has made significant strides in its latest development, a text-to-3D object system called Shap-E AI, reported first by New Atlas.
This generative AI tool is available for open-source download, and it can generate 3D assets directly from text descriptions or even construct them using supplied images.
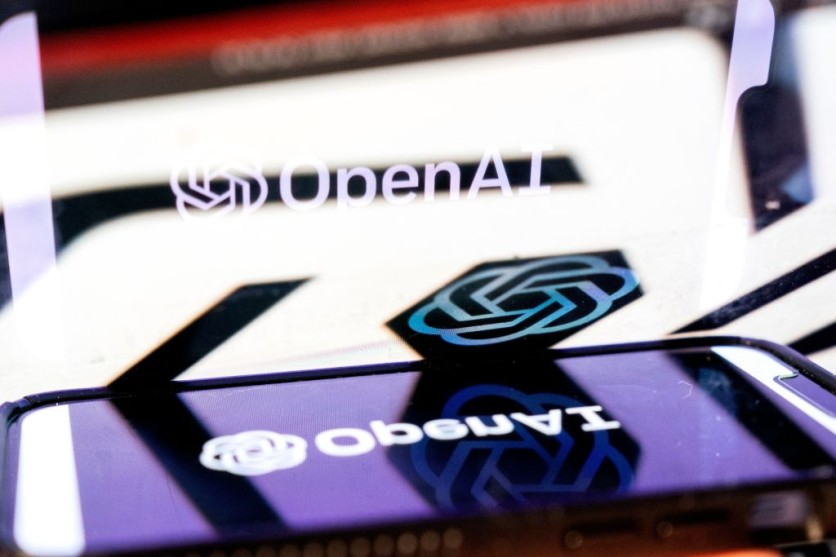
"Implicit Functions"
In a previous release called Point-E, OpenAI introduced a system capable of transforming text prompts into basic 3D models in the form of point clouds.
However, the new Shap-E system represents a major leap forward, as it is not only faster but also capable of building models as "implicit functions."
These functions are mathematical formulas that can be rendered as textured meshes or neural radiance fields (NeRFs), which are 3D models generated from 2D images via machine learning.
While the technical aspects may seem complex, the potential applications are truly fascinating. These 3D models are specifically designed to be compatible with downstream applications, leading to exciting possibilities.
This technology could represent an early step toward verbally-programmed 3D visual effects, offering the potential to generate everything from virtual outfits to personalized homes or even virtual companions in VR/AR applications.
As the capabilities of the Shap-E system progress, it is expected to interface seamlessly with 3D printing. This means that the shapes created by these AI systems could soon become tangible objects in the real world, produced with higher quality.
In the future, users may not directly interact with the system itself but communicate with a language model-based AI assistant that will generate the appropriate prompts for the 3D-maker AI, resulting in more efficient and accurate outputs.
Read Also : Meta's AI Sandbox is a Generative AI for Advertisers Now Available for Users to Test New Tools
Conditional Generative Model
OpenAI introduces Shap-E as a revolutionary conditional generative model for 3D assets. Unlike previous 3D generative models that produce a single output representation, Shap-E directly generates the parameters of implicit functions that can be rendered as both textured meshes and neural radiance fields.
The training process involves two stages: first, an encoder is trained to map 3D assets into the parameters of an implicit function; second, a conditional diffusion model is trained using the encoder's outputs.
By training on a vast dataset of paired 3D and text data, the resulting models exhibit the capability to generate intricate and diverse 3D assets within seconds.
In comparison to Point-E, an explicit generative model over point clouds, Shap-E demonstrates faster convergence and achieves comparable or even superior sample quality despite modeling a higher-dimensional, multi-representation output space, as stated by the researchers behind the new system.
OpenAI has made the model weights, inference code, and samples available to the public, enabling further exploration and innovation in the field. It is available here.
Related Article : Microsoft Introduces Bing Chat, Features More than AI Text Generation, Available for Open Preview
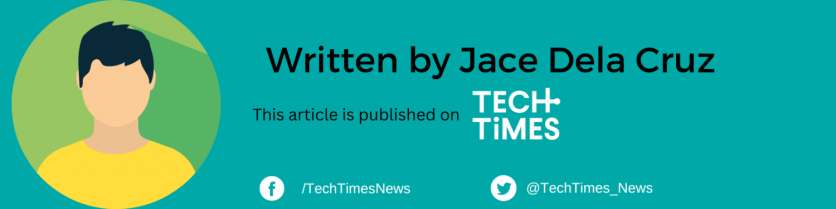