Researchers at Carnegie Mellon University have developed a new method for determining parts of the genome that are crucial to understanding how species traits of species evolved.
Under the leadership of Andreas Pfenning, an Assistant Professor in the School of Computer Science, the Zoonomia Project aims to sequence the entire genomes of 240 mammals.
The aim of this endeavor is to shed light on essential features of genes and traits that carry considerable importance in safeguarding human health and preserving the diversity of species.
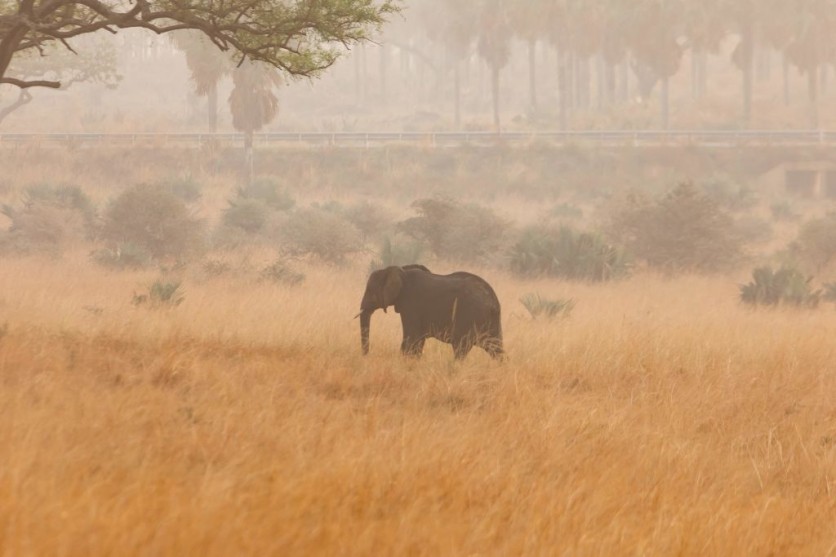
The TACIT
To gain a better understanding of how enhancer regions of the genome function, the research team developed a machine-learning approach known as the Tissue-Aware Conservation Inference Toolkit (TACIT).
Enhancers, which are noncoding DNA regions, control the activation of specific genes, playing a crucial role in the evolution of species. Since enhancers account for a significant portion of the genome, their study is essential to understanding evolution.
"TACIT provides an unprecedented opportunity to predict the function of parts of the genome outside of genes in species for which we cannot get primary tissue samples," said Irene Kaplow, a lead author on the paper and a postdoctoral associate and Lane Fellow in CBD.
"As ML methods and methods for identifying enhancers from specific cell types improve, I anticipate that we will be able to broaden the functions of TACIT to provide new kinds of insights into mammalian evolution."
Read Also : Researchers Develop Machine Learning Prescriptions and Reduce 'Antibiotic Resistance' By Half
Genomic Sequences in 240 Mammals
The research team used TACIT to predict the function of genomic sequences in the 240 mammals and subsequently applied it to detect the genomic regions that have evolved for bigger brains in these animals. Their findings revealed that these regions were often located near genes known to be associated with brain-size disorders in humans.
Additionally, the team identified an enhancer linked to social behavior in mammals, which is specific to a particular type of neuron known as the parvalbumin-positive inhibitory interneuron.
"We think this is just the tip of the iceberg," said Andreas Pfenning, senior author of the study.
"We found interesting relationships by applying TACIT to a small number of tissues and a small number of traits, but there is still a lot more to discover."
This breakthrough in using machine learning to identify enhancer regions has important implications for conservation biology, as it allows scientists to make predictions about how enhancers function in endangered or threatened species where controlled laboratory experiments are impossible.
By identifying which parts of the genome have evolved for certain traits, scientists can better understand how species have adapted over time and develop new strategies for protecting biodiversity.
The team's findings were published in the journal Science.
Related Article : Biotech Startup Uses Machine Learning Algorithms to Predict the Progress of Cancerous Tumors | How Does it Work
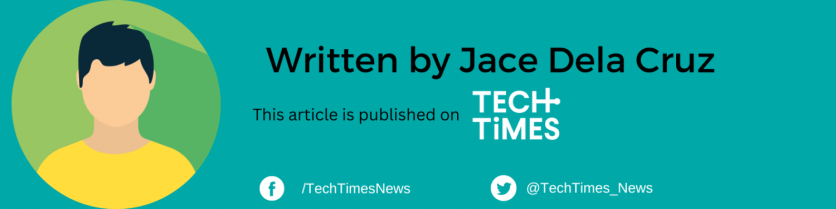