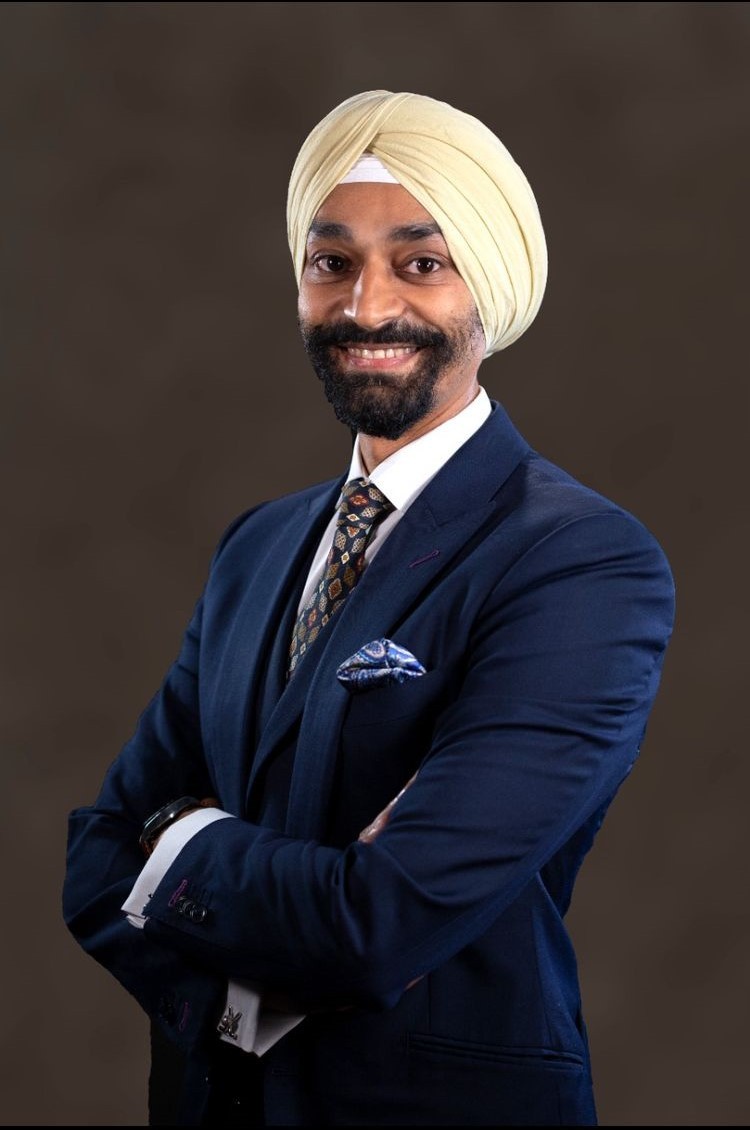
The manufacturing industry has always struggled with balancing raw materials supply and demand. Finding the right balance is crucial as it can impact both production costs and profits. Inefficiencies in raw material management can lead to costly overproduction, excess inventory, and reduced profitability. The solution to this problem lies in advanced analytics and optimization, which can help manufacturers optimize their raw material management processes and maximize their profits.
In a telephonic conversation with TechTimes, Gunangad Singh Maini, who is an LDP in strategic supply chain planning at Niagara Bottling spoke about how advanced analytics can optimize raw material management. Maini, who has worked with this bottling giant since 2022, was responsible for the optimized raw material planning for Niagara's largest manufacturing plant that resulted in significant reduction in its holding costs, logistics costs and improved optimized warehouse space allocation for finished goods and raw materials.
According to Maini, advanced analytics and optimization techniques such as predictive modeling, machine learning, and simulation can help manufacturers in several ways. These tools can be used to forecast demand, optimize inventory levels, and identify opportunities to improve operational efficiency.
Benefits of advanced analytics and optimization for raw material management:
Improved forecast accuracy:
Advanced analytics and optimization techniques can help manufacturers improve their forecast accuracy for raw materials, which can have several benefits. By analyzing historical data on raw material consumption, manufacturers can identify patterns and trends in their usage. This can help them make more accurate predictions about future demand, based on factors such as seasonality, market trends, and other external factors. Similarly, machine learning algorithms and predictive models can be trained on historical data to identify patterns and make accurate predictions about future demand. These models can be updated regularly to reflect changing market conditions and demand patterns. Real-time data on inventory levels, production schedules, and customer orders can also be analyzed using advanced analytics techniques such as data mining and machine learning. This can help manufacturers adjust their production schedules in real-time to meet changing demand, reducing the risk of overproduction and excess inventory. By accurately predicting demand for raw materials, manufacturers can plan their production schedules better, ensuring that they have the right amount of materials on hand to meet customer orders without excess inventory. This can lead to cost savings by reducing waste and avoiding the need for costly storage and handling of excess inventory. Additionally, accurate demand forecasting can help manufacturers identify opportunities for process optimization and efficiency improvements, leading to further cost savings and competitive advantage.
Better inventory management:
Advanced analytics and optimization techniques can also help manufacturers improve their inventory management. Optimization models can be used to determine the optimal inventory levels for raw materials, reducing the risk of stockouts or overstocking. These models take into account factors such as demand variability, lead times, and supply chain constraints to calculate the optimal levels of inventory required to meet customer demand. By maintaining optimal inventory levels, manufacturers can reduce the risk of stockouts and the associated costs of rush orders and lost sales, while also avoiding the costs of excess inventory and the associated storage and handling fees. This can lead to cost savings, improved customer satisfaction, and a more efficient supply chain. Furthermore, optimization models can be updated in real-time as demand patterns and supply chain conditions change, ensuring that manufacturers always have the optimal levels of inventory on hand to meet customer demand.
Optimization models can also identify opportunities to reduce holding costs associated with raw material storage and transportation. All in all, by optimizing the raw material management process, manufacturers can streamline their production processes, reduce waste, and improve their overall efficiency.
Best practices for implementing advanced analytics and optimization for raw material management:
Maini who has extensive experience in successfully managing complex projects, including chiller automation of the tallest building in the world, the largest gas-to-liquids (GTL) plant in the world, the largest shopping mall in the world and leading the replacement and installation of 1 million smart meters on time and within budget under strict deadlines for Dubai's largest utility and was responsible for overseeing a team of 70 engineers and technicians, where he optimized operations across 5 engineering divisions, stated that to successfully implement advanced analytics and optimization for raw material management, manufacturers should consider the following best practices:
Start with a pilot project: A pilot project can help identify potential challenges and opportunities before scaling up to a full implementation.
Collaborate across departments: Raw material management involves multiple departments, and collaboration is key to optimizing the entire process.
Use a scalable solution: To achieve long-term success, it's important to use a solution that can scale as the business grows and evolves.
Leverage external expertise: Engaging with external experts can help bring in specialized skills and knowledge that may not be available in-house.
Conclusion:
Advanced analytics and optimization are powerful tools that can help manufacturers improve their raw material management processes, leading to increased profits and operational efficiency. "By using these tools, manufacturers can reduce the risk of overproduction, stockouts, and excess inventory, and optimize their raw material balance to achieve a sustainable competitive advantage, "says Maini.