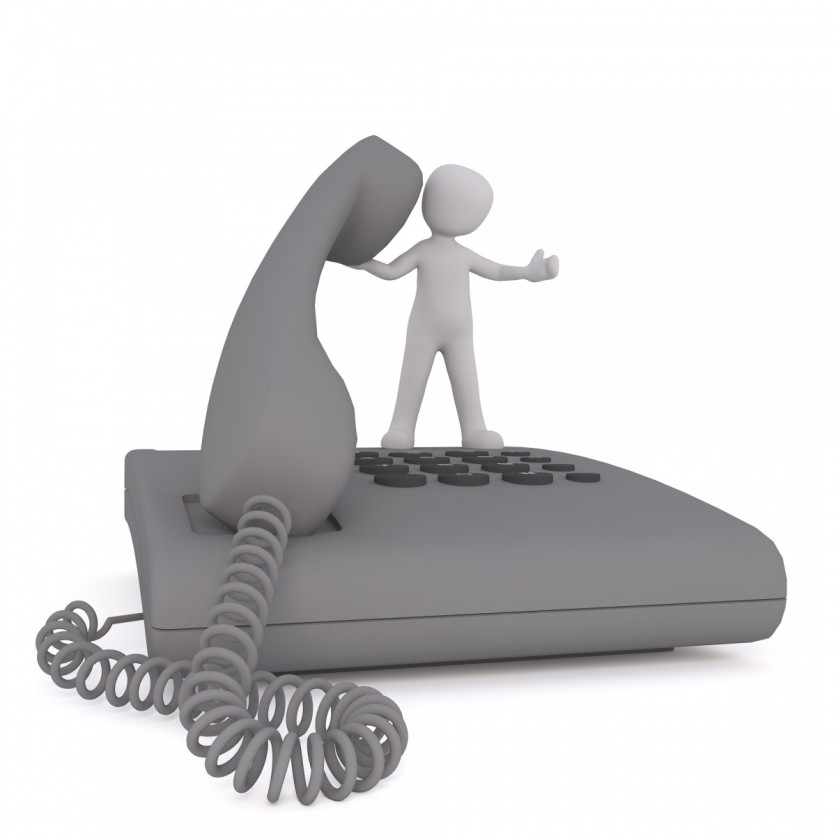
From analogue telephone lines to 5G connectivity, the telecommunications industry has grown by leaps and bounds in just a quarter of a century. The internet of things (IoT) is ushering us into an age of unprecedented connectivity. The past few years - especially post-pandemic - have witnessed a surge in the remote working model and, with it, the proliferation of IP technologies, multimedia applications, next-generation networks, and multiple access methods. These changes have driven rapid uptake among the masses, and with good reason.
Unfortunately, the changed landscape lends itself too easily to malpractices. A significant fallout of the altered work patterns is burgeoning telecommunications fraud. According to industry estimates, a whopping 90% of operators encounter scammers daily,[1] creating a pressing need to turn to artificial intelligence (AI) for telecom fraud prevention.
Types of telecommunications fraud
Let us have a look at what constitutes telecommunications fraud. Fraudulent activities in telecom fall into three broad categories, such as:
Subscribers as the target: This includes scams such as identity theft, PBX hacking, and international revenue sharing fraud (IRSF).
Service providers as the target: SIM box frauds, roaming arbitrage frauds, and first-party subscription frauds fall into this category.
General phone fraud: General fraud includes all types of fraudulent behaviour experienced over the telephone, such as Wangiri (one-ring-and-cut) fraud and common cases of SMS phishing. Although phishing may not result in revenue losses for the telcos, it may cause a serious loss of reputation.
Why telcos need to reconsider their traditional fraud detection systems?
Traditional fraud detection systems are inadequate - systems equipped to 'detect' rather than foresee or prevent disaster. Here are some of the fundamental shortcomings of current fraud management systems.
They function on rules-based software, which is trained to detect known fraud patterns. They are thus equipped to fight common cases of subscription fraud, superimposed fraud, or PBX hacking. However, they fall short as fraudsters foray deeper into telecom ecosystems and find sophisticated ways to circumvent rule-based procedures.
The global reach of the internet means that frauds are not limited to one country. Adding to the challenge is that bigger telcos resell their services to local networks, which are more vulnerable and less traceable.
Call volumes have grown exponentially in a short span of time and traditional systems are unable to process the sheer amount of data generated through them.
The only way out for telcos is to upgrade their infrastructure from conservative fraud detection models to intelligent systems that self-adapt to changing fraud patterns.
AI in fraud prevention
The biggest limitation of rules-based fraud management systems is that they do not 'learn' or update on their own. They require constant manual intervention to be effective in non-conformist scenarios. We can tweak the rules to detect new attack patterns; however, increasing the rules complexity results in complicating their management as well. What communication service providers (CSPs) need is to deploy fraud prevention models with AI and ML (machine learning) that can potentially:
Handle large volumes of data
Deal with increasing levels of agility and sophistication in fraudulent behaviour
Identify trends and anomalies and use them to forecast frauds before they occur
Update and evolve in response to a dynamic landscape
Six ways AI in fraud prevention can spell big wins
1. Efficient data handling
The exponential growth of the telecom sector, and of call volumes in particular, generates an over-abundance of data, which traditional detection systems are unable to process. Despite the burgeoning crime rates, crime data forms only a miniscule portion of the total call information, leading to a 'data imbalance.'
Traditional detection systems - and humans who are in the loop - are often unable to flag anomalous activity within this tiny window. Deploying AI for the task can eliminate the need for human intervention, ensure that the available data is handled efficiently, and minimise false positives.
2. Spotting usage patterns and anomalies in real time
Traditional systems merely provide descriptive data such as altered traffic levels or changes in call duration. Interpreting anomalies is left to human experts. AI-based models apply diagnostic and predictive analytics to statistical data to detect abnormal patterns and raise alarms in real time, thus averting fraud.
3. Minimising damage with diagnostic and predictive analytics
By converting data into diagnostic and predictive insights, AI-powered systems can detect incongruities such as unauthorised network access and fake profiles. They block access to suspected fraudsters, thus minimising damage.
4. Tracking assets with movement analytics
AI and ML engines continually monitor the place and nature of service usage by tracking device movement paths. This is useful in case of duplicated SIM cards, devices used in suspicious locations, or roaming arbitrage cases.
5. Plugging vulnerabilities through transaction data analysis
AI in fraud prevention can read invoice content and amounts, detect suspicious credit transfers, or credit accumulation on prepaid accounts. By combining this data with usage patterns and historical data, AI can detect suspicious activity and prompt billing teams to check for potential fraud.
6. Anticipating future threats
Intelligence on case outcomes feeds back into the detection system, enabling its algorithms to update automatically and evolve in response to changing fraud patterns. This helps to anticipate future threats and prevent potential fraud.
Implementing AI in fraud prevention
Considering that telcos lose 2% or over $1.5 trillion annually[2] to fraudulent transactions, they need to leverage AI's superior analytical capabilities at the earliest. AI and ML together can help handle humungous amounts of data, plug network vulnerabilities, detect or even predict anomalies faster, and facilitate self-updating fraud prevention models.
The recent success of a Canadian telecommunications company with AI is a case in point.[3] By leveraging AI best practices, this telecom giant has developed a 'self-tuning' fraud detection solution that enables:
10% increase in scam detection
150% improvement in the time taken for detecting losses, post the occurrence of fraud
200% improvement in the time taken for identifying new fraud schemes
Here is a quick checklist for successfully transitioning to AI from your existing fraud management system:
Choose AI and ML-integrated solutions that provide effective assessment and automation.
Integrate supervised and unsupervised ML models. The former are better are identifying common fraud types, while the latter (using unlabeled data) can be more effective in detecting non-conformist fraud patterns.
Store data from different time periods and perform data cleaning regularly to ensure that your system performs optimally.
Conduct gap analysis to identify your workforce and workflow holes. AI can automate workflows efficiently only when the gaps are bridged.
Ensure that employees have the right skill sets to work alongside AI. After all, implementing AI in fraud prevention is a human as well as a technology change.