Artificial intelligence has advanced rapidly in several sectors and its applications have become expansive as well. However, researchers argue that despite its advancement, it will never measure up to a human's visual processing ability.
In real-world AI applications, Deep Convolutional Neural Networks (DCNNs) may not perceive things in the same manner that humans do due to configural form perception, says Professor James Elder, a co-author of a new York University study that was released in the journal iScience.
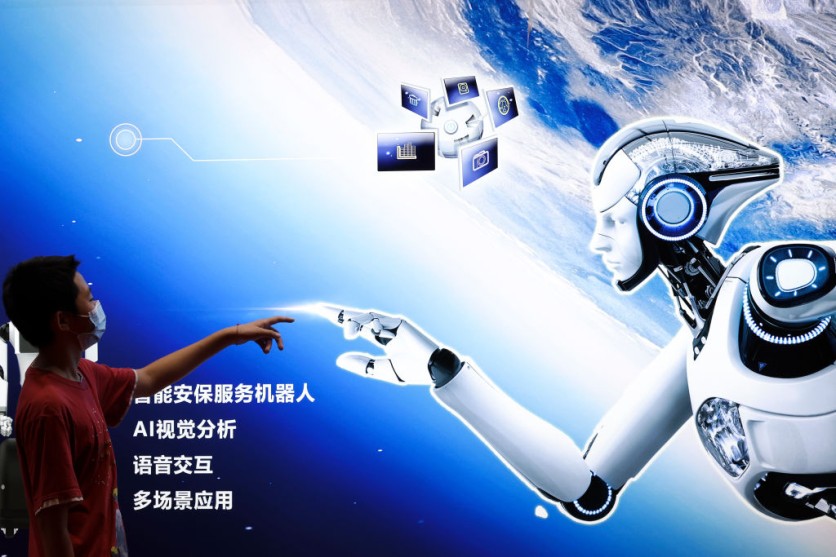
Deep Learning Models
As reported first by SciTechDaily, deep learning models fall short in capturing the configural nature of human shape perception, according to a study led by Elder, who holds the York Research Chair in Human and Computer Vision.
The findings illuminate why deep AI models falter in specific scenarios and emphasize the necessity to take into account activities other than object recognition in order to comprehend how the brain processes visual information, as per Elder.
Elder emphasizes that when attempting to solve challenging recognition problems, these deep models frequently use "shortcuts."
Although these quick cuts may be effective in many situations, the author notes that they can be risky in some real-world AI applications we are now developing with industrial and government partners.
An example of such use is traffic video safety systems. Elder noted that "the objects in a busy traffic scene - the vehicles, bicycles, and pedestrians - obstruct each other and arrive at the eye of a driver as a jumble of disconnected fragments."
He emphasized that the brain must correctly categorize those fragments to identify accurate categories and locations of objects. He claims that an AI system for safety monitoring in traffic will only perceive those fragments individually, which ultimately fails at its task.
Elder said that this could lead to "potential misunderstanding risks" for road users.
According to the research team, networks need to be trained to handle more complex object problems than only category classification to match human configurable sensitivity.
Bias in AI
In another recent study published in Philosophy and Technology, researchers from Cambridge's Centre for Gender Studies claim that AI recruiting tools are superficial and comparable to "automated pseudoscience."
They assert that it is a dangerous example of "technosolutionism," which they define as the use of technology to address difficult problems like discrimination without making the necessary investments or changes to organizational culture.
According to a news release from the university, the researchers worked with a group of undergraduate computer science students at Cambridge to create an online AI tool to debunk claims that AI eliminates bias in the workplace.
The "Personality Machine" demonstrates how arbitrary changes to facial expression, attire, lighting, and background may result in radically different personality readings, which could mean the difference between being rejected and being hired out of the current crop of job applicants vying for graduate positions.
The Cambridge team claims that when AI is used to narrow candidate pools since it is designed to find the employer's ideal candidate, it may ultimately promote uniformity rather than variation in the workforce.
Related Article : This New AI-Powered Method Can Accurately Predict Where and When Wildfires Will Occur
This article is owned by Tech Times
Written by Jace Dela Cruz
ⓒ 2025 TECHTIMES.com All rights reserved. Do not reproduce without permission.