The Massachusetts Institute of Technology (MIT) researchers have found a way for drivers to avoid idling at red lights through Artificial Intelligence (AI).
In a new study, MIT researchers demonstrate a machine-learning approach that can help control a fleet of autonomous vehicles as they approach a signalized intersection to help traffic go smoothly.
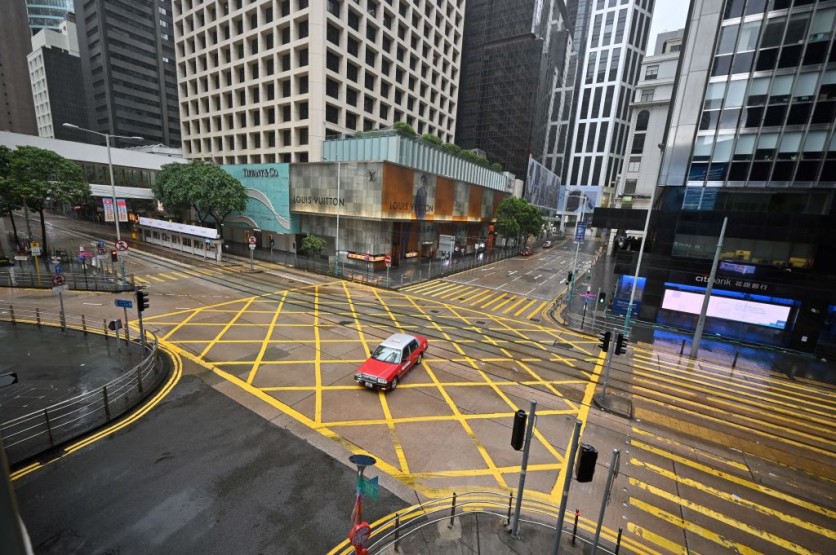
The researchers performed simulations that not only will the approach help with traffic, but can also reduce fuel consumption and emissions will improve the average vehicle speed.
This approach will deliver the best results if all cars on the road are autonomous. However, even if only 25 percent use this, it may still deliver significant results.
According to senior author Cathy Wu, an assistant professor of the Gilbert W. Winslow Career Development in the Department of Civil and Environmental Engineering, "This is really interesting place to intervene."
He adds, "No one's life is better because they were stuck at an intersection."
She also referenced the ongoing interventions in climate change that could impact the quality of life. Wu mentioned that the improvements in the quality of life through their approach using AI could be more possible in the near-term, which would have also a positive impact on our climate.
Also Read: AI Will NOT Take Over The World And Drive Humanity To Extinction--Here's Why
The Technique
The scientists approached it with a model-free technique, which is also known as deep reinforcement learning. Deep reinforcement learning is a trial-and-error method where the control algorithm learns to make a sequence of decisions.
It is rewarded when it finds a good sequence and leverages assumptions learned by a neural network that finds shortcuts to good sequences, despite the billions of possibilities.
Moreover, they want the system to learn a strategy to help reduce fuel consumption and limit the impact on travel time, which causes conflict.
To solve this, they employed reward shaping, a technique that gives the system some domain knowledge that it won't learn on its own. In this case, they penalized the system every time the vehicle came to a full stop to avoid that action.
Following an effective control algorithm, the researchers evaluated it through a traffic simulation platform with a single intersection. They applied it to a fleet of connected autonomous vehicles that communicate with upcoming traffic lights to receive signal phase and timing information and observe their immediate surroundings.
The algorithm tells each vehicle how to accelerate and decelerate. In the simulations, there are more cars than they made through in a single green phase, which resulted in bigger fuel consumption and emissions reductions.
The work is still in its early stages but the researchers are optimistic that they could be implemented in the near future.
The future of AI in vehicles seem to be getting more solid as time goes by. It's safe to say that this technology will could really pave the way to a safer environment.
Related Article: MIT Scientists Build VR Training Grounds For AI Drones
This article is owned by TechTimes
Written by April Fowell